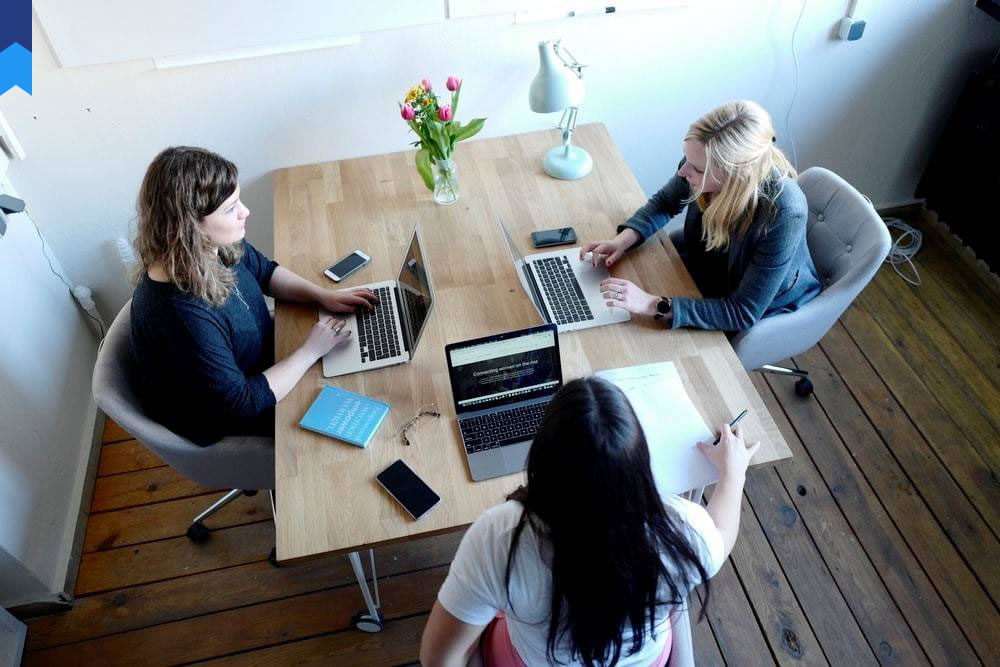
What Neuroscience Can Teach Us About Personalized Learning
Personalized learning, the tailoring of education to individual student needs, is a rapidly evolving field within EdTech. While the promise is immense – improved student outcomes, increased engagement, and reduced learning gaps – the reality is often hampered by a lack of deep understanding of the learning process itself. This is where neuroscience steps in, offering invaluable insights into how the brain learns, remembers, and processes information.
The Power of Neuroplasticity in Adaptive Learning Platforms
Neuroplasticity, the brain's remarkable ability to reorganize itself by forming new neural connections throughout life, is fundamental to effective learning. Adaptive learning platforms, powered by artificial intelligence, leverage this principle by constantly adjusting the difficulty and content based on a student's performance. For instance, a platform might identify a student struggling with a particular concept and provide additional practice exercises, or offer alternative explanations tailored to their learning style. Khan Academy’s adaptive exercises exemplify this approach, dynamically adjusting the complexity of problems based on student responses. Another example is DreamBox Learning, which uses adaptive algorithms to personalize math instruction, catering to individual learning paces and strengths.
Studies have shown that personalized learning interventions significantly improve academic outcomes. A meta-analysis of over 200 studies found that personalized learning led to a substantial improvement in student achievement (Hattie, 2009). However, these gains are often amplified when informed by a neuroscientific understanding of how individual brains respond to different teaching methods and learning materials. For example, understanding working memory capacity can inform the design of microlearning modules, preventing cognitive overload. A study by Klingberg et al. (2002) demonstrated the trainability of working memory, suggesting that targeted interventions can significantly enhance cognitive skills vital for learning.
Furthermore, neuroimaging techniques like fMRI can reveal brain activity patterns associated with different learning strategies, providing objective data to optimize learning experiences. By analyzing brain activity during learning, educators can identify optimal approaches for each student. For example, some students might benefit from visual aids, while others may respond better to auditory explanations. This approach allows for a deeper understanding of personalized learning than simply relying on student self-reports or teacher intuition.
The integration of neuroscience with adaptive learning platforms is still in its early stages. However, future developments promise sophisticated systems that can dynamically adjust the learning path based on real-time brain activity data. This would involve the development of non-invasive brain-computer interfaces that continuously monitor learning processes and optimize the delivery of educational content. Imagine a future where every student has their own personalized learning ecosystem, dynamically responsive to their unique cognitive profile.
Emotional Engagement and the Reward System: Motivating Learners
Neuroscience highlights the crucial role of emotions in learning. The brain's reward system, involving dopamine and other neurotransmitters, plays a critical role in motivation and engagement. Effective personalized learning systems should leverage this knowledge by incorporating elements that trigger positive emotions and a sense of accomplishment. Gamification, for instance, uses game mechanics to motivate students, capitalizing on the brain's reward pathways. Duolingo and other language-learning apps successfully incorporate gamification techniques, offering points, badges, and leaderboards to enhance motivation and engagement. This approach aligns with the neuroscientific principle of rewarding desired behaviors, leading to improved learning outcomes.
Personalized feedback, tailored to the individual student's strengths and weaknesses, also plays a crucial role in optimizing the reward system. Positive reinforcement, focusing on achievements and progress, is far more effective than simply pointing out errors. A study by Deci and Ryan (2000) showed that intrinsic motivation, driven by internal rewards, is crucial for sustained learning. Personalized learning, by providing tailored support and acknowledgment of effort, can significantly boost intrinsic motivation.
Furthermore, understanding individual differences in emotional regulation can inform the design of effective learning experiences. Some students may be more susceptible to anxiety or frustration, requiring strategies to manage emotional responses during challenging tasks. Mindfulness techniques, integrated into personalized learning platforms, can help students regulate their emotions and enhance focus. This approach aligns with the growing body of research on the benefits of mindfulness for cognitive performance and emotional well-being.
The incorporation of emotional intelligence principles into personalized learning systems is a key area for future development. Personalized systems can potentially identify students at risk of emotional distress and provide targeted interventions to improve their mental health and academic performance. This integration would leverage advances in affective computing, which uses technology to analyze emotional states, allowing for more responsive and supportive learning environments. A future where technology can empathize with the learner is within reach.
Cognitive Load and the Design of Effective Learning Materials
Cognitive load theory, a cornerstone of instructional design, emphasizes the limitations of working memory. Neuroscience provides a deeper understanding of these limitations, informing the design of learning materials that minimize cognitive overload. Personalized learning systems can utilize this knowledge to present information in manageable chunks, optimizing the flow of information and reducing the burden on working memory. Breaking complex topics into smaller, digestible modules, and providing frequent opportunities for practice and feedback, is a key principle of this approach.
The use of multimedia learning principles is also crucial. Research by Mayer (2009) demonstrated the effectiveness of multimedia learning, combining visual and auditory channels to enhance learning. Personalized learning systems can adjust the format and modality of information based on individual learning preferences, ensuring optimal engagement and comprehension. For example, some students may benefit from visual learning materials, such as diagrams and videos, while others may prefer auditory learning, such as audio lectures or podcasts.
Furthermore, the design of assessment tools can significantly impact cognitive load. Personalized learning systems should incorporate assessments that are appropriately challenging and provide valuable feedback, without overwhelming the student. The use of formative assessment, integrated throughout the learning process, allows for continuous monitoring of understanding and timely adjustments to the learning path. This approach aligns with the neuroscientific understanding of learning as a continuous process of refinement and consolidation.
Future personalized learning systems may utilize sophisticated algorithms to analyze cognitive load in real-time, automatically adjusting the presentation of information based on individual student needs. This would involve the development of advanced technologies that can monitor brain activity and cognitive processes, providing insights into the optimal pace and complexity of learning materials. This would allow systems to seamlessly adapt to a learner's cognitive capacity, maximizing learning efficiency.
Metacognition and Self-Regulated Learning: Empowering Students
Metacognition, or "thinking about thinking," refers to the ability to reflect on one's own learning processes. Self-regulated learning, guided by metacognitive strategies, is crucial for achieving academic success. Personalized learning systems can foster metacognition by incorporating features that encourage self-reflection and self-monitoring. For example, students can be prompted to reflect on their learning strategies, identify areas of strength and weakness, and set learning goals. Tools that allow students to track their progress, visualize their learning journey, and engage in self-assessment are vital in this context.
The incorporation of learning analytics, which uses data to track student progress and identify learning patterns, can provide valuable insights into individual learning styles and needs. This data can be used to personalize feedback, suggest alternative strategies, and promote self-regulated learning. For instance, a personalized learning system might identify a student struggling with time management and suggest strategies for improved organization and planning. This data-driven approach allows educators to effectively support and empower students.
Furthermore, personalized learning systems can incorporate tools that promote self-directed learning. Students can be given greater control over their learning path, selecting topics that interest them, choosing their preferred learning methods, and setting their own learning goals. This aligns with the growing emphasis on learner autonomy and agency. The provision of open educational resources (OER) and self-paced learning modules gives students greater control and fosters a sense of ownership over their learning.
Future advancements in artificial intelligence may lead to the development of intelligent tutoring systems that can provide personalized metacognitive support. These systems could analyze student performance and provide tailored feedback, suggestions for improvement, and guidance on effective learning strategies. Imagine a future where AI tutors can not only provide answers but also help students understand their own learning processes and develop their metacognitive skills.
The Future of Personalized Learning: Integrating Neuroscience and Technology
The integration of neuroscience and technology holds immense promise for the future of personalized learning. As our understanding of the brain deepens, and as technology becomes more sophisticated, we can expect to see increasingly personalized and effective learning experiences. The development of sophisticated brain-computer interfaces, capable of monitoring brain activity in real-time, will revolutionize the way we design and deliver education. This will allow for dynamic adaptation to individual learning needs, ensuring that every student has access to the optimal learning environment.
The use of artificial intelligence will also play a crucial role. AI-powered systems can analyze vast amounts of data to identify individual learning patterns, predict challenges, and provide targeted interventions. This data-driven approach will allow for continuous improvement of personalized learning systems, ensuring that they remain effective and responsive to individual student needs. The future is about adapting to different learning styles seamlessly.
However, ethical considerations must be addressed as we move towards increasingly personalized learning experiences. Data privacy, algorithmic bias, and the potential for over-reliance on technology are critical issues that need careful consideration. It’s crucial to ensure that personalized learning systems are used responsibly, promoting equity and access for all students. Transparency and user control are paramount in the development and deployment of these technologies.
In conclusion, the integration of neuroscience into personalized learning is a transformative development, offering the potential to significantly improve educational outcomes for all students. By leveraging insights from neuroscience, we can design more engaging, effective, and equitable learning experiences, empowering students to reach their full potential.
CONCLUSION:
The convergence of neuroscience and EdTech is revolutionizing personalized learning. By understanding how the brain learns, we can create more effective and engaging learning environments tailored to individual needs. This involves harnessing the power of neuroplasticity, optimizing emotional engagement, managing cognitive load, and fostering metacognition. While challenges remain, the future holds immense promise for personalized learning, driven by advancements in technology and a deeper understanding of the human brain. The potential for improved learning outcomes is significant, but responsible development and ethical considerations must remain central to this transformative process. Personalized learning is not just about technology; it is about understanding and responding to the unique learning journey of each individual.