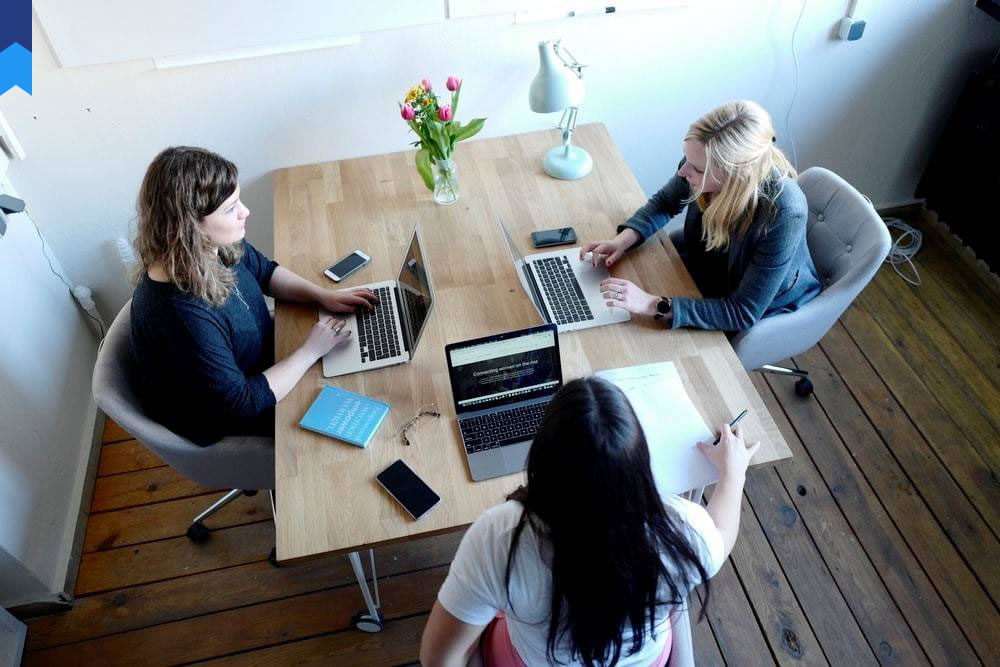
How to Choose the Perfect Data Labeling Service
One of the main hindrances to AI adoption for 24% of organizations is "the absence of tools or platforms for model development" and "the overwhelming complexity of data."
These challenges can be addressed by incorporating your data labeling process into a centralized, automated MLOps pipeline. With the right training data tools, you can achieve smooth integration with your entire tech stack, enabling you to develop AI products more quickly, cost-effectively, and with minimized risk.
In this blog, we’ll share some tips for sourcing data labeling platforms so you can develop robust AI models.
1. Types of Data Annotation
The data labeling tool you select must support all the data formats and annotation types necessary for your project.
While it might seem easy to check initially, looking closely at each tool's features is essential. Even if data labeling providers make promises, you should ensure the platform you choose invests in solutions for your specific industry.
For example, if you're working on annotating data for autonomous vehicles, find a platform that specializes in that area, regularly adds new features for the automotive sector, and has experts in autonomous technology to help you get the best results.
Make the most of the demos provided by each platform. Use this opportunity to inquire about your specific data and annotation needs, especially if you have numerous edge cases with unique features that might need customization.
2. Customer Support
Many data training platforms offer excellent data labeling features that help optimize annotation. However, no system is perfect. Selecting a tool with a responsive support team that can quickly address any issues is crucial, helping minimize downtime and keep your project moving smoothly.
Exceptional customer success and support teams do more than solve problems; they collaborate in your AI product development. These teams provide industry knowledge and software expertise, enhancing your data labeling process by introducing new technologies, staying abreast of industry trends, and proactively finding solutions to challenges you face with your training data.
Here are some of the main things you should expect:
- Quick Response Times: The support team should respond promptly to inquiries and issues. This minimizes downtime and helps maintain productivity.
- Technical Expertise: The team should deeply understand the data labeling tools and processes. This allows them to provide accurate and effective solutions to technical issues.
- Proactive Communication: Good support teams don’t just react to problems; they anticipate potential challenges and communicate with clients about upcoming updates, changes, or improvements.
- Customization Assistance: Since data labeling needs can vary widely depending on the project, the support team should be able to help customize the tools to fit specific requirements.
- Training and Resources: Providing comprehensive training sessions and resources helps clients make the most of the data labeling platform. This includes tutorials, FAQs, and best practice guides.
- Problem-Solving Capabilities: Beyond fixing bugs, the support team should be skilled in troubleshooting and devising strategic solutions to complex problems that may arise.
3. Consider your Company Size
Early-stage companies should focus on different priorities compared to those rapidly expanding or already at a large scale.
Why?
If your company is just starting out and you're building your own tool with open-source solutions, you'll need to find your own team. You could use crowdsourcing and the vendor's platform, but remember, crowdsourced workers are often anonymous and unvetted.
This means you won't have the same team working consistently, which can affect the quality and context of your data. Whether you build or buy a solution, ask vendors about their experience with different tools and if they can offer any recommendations.
If your company is growing and speeding up development, open-source tools give you more control over security, integration, and the flexibility to make changes. On the other hand, commercial tooling software often allows you to configure and deploy competitive features efficiently.
Open-source tools are well-planned and tested if your company operates at scale and aims to maintain this over time. These tools can support core long-term processes and stack integration, giving you maximum control over security and the flexibility to make changes. At this level, if you opt for commercial software, you can often get customized tooling tailored to your needs without requiring extensive development resources.
4. Cost Considerations
Data labeling service providers battle when it comes to price. They all understand that developing and successfully launching an AI model is expensive for the AI company; as a result, they’ll try to keep their prices low. However, they don’t tell you that their low charges affect the data quality you get.
This doesn’t mean you should choose expensive tools. It’s all about getting the right provider with the right budget to guarantee high-quality data.
That said, compare the pricing models of different tools to ensure they fit within your budget in the short and long term. Consider whether the tool requires a subscription, pay-per-use, or a one-time purchase, and assess any additional costs for features, support, or scaling.
It's also important to weigh the potential return on investment (ROI) by considering how the tool's efficiency and accuracy improvements might offset its cost. Ultimately, your goal should be to find a tool that offers the best value for your investment, balancing affordability with functionality and performance.
5. Reporting and Analytics Features
Effective reporting and analytics are crucial for tracking the progress and efficiency of your data labeling process. When evaluating a data labeling tool, look for robust reporting features that provide detailed insights into your labeling activities.
These features should include labeling speed, accuracy, and annotation quality metrics. Analytics tools can help you identify bottlenecks, monitor the performance of your labeling team, and make data-driven decisions to optimize the process.
A tool with comprehensive reporting and analytics capabilities will enable you to continually improve your labeling workflow and ensure high-quality data for your AI models.
6. Security and Compliance
Security and compliance should be top priorities when selecting a data labeling tool, especially when working with sensitive or proprietary data. Ensure that the tool adheres to industry-standard security protocols and offers features like encryption, access controls, and audit logs to protect your data from unauthorized access and breaches.
Additionally, check if the tool complies with relevant data protection regulations, such as GDPR, CCPA, or HIPAA, depending on your industry and location.
A secure and compliant data labeling tool safeguards your data. It helps maintain trust with your clients and stakeholders, reducing the risk of legal and financial repercussions associated with data breaches or non-compliance.
Conclusion
A lot goes into developing an AI model, and collecting data is even more challenging. That’s why you should be careful when choosing a data labeling service. You don’t want to risk all your hard work done during the research and development with low-quality service that reduces the effectiveness of your model.
By following these tips and keeping your long-term goals in mind, you can pick a data labeling tool that improves your data quality, speeds up your AI development, and leads to greater success and innovation in your projects.
You can consider data labeling services like Pareto AI, a human data collection platform that connects veteran data labelers and AI companies.
SIIT Courses and Certification
Also Online IT Certification Courses & Online Technical Certificate Programs