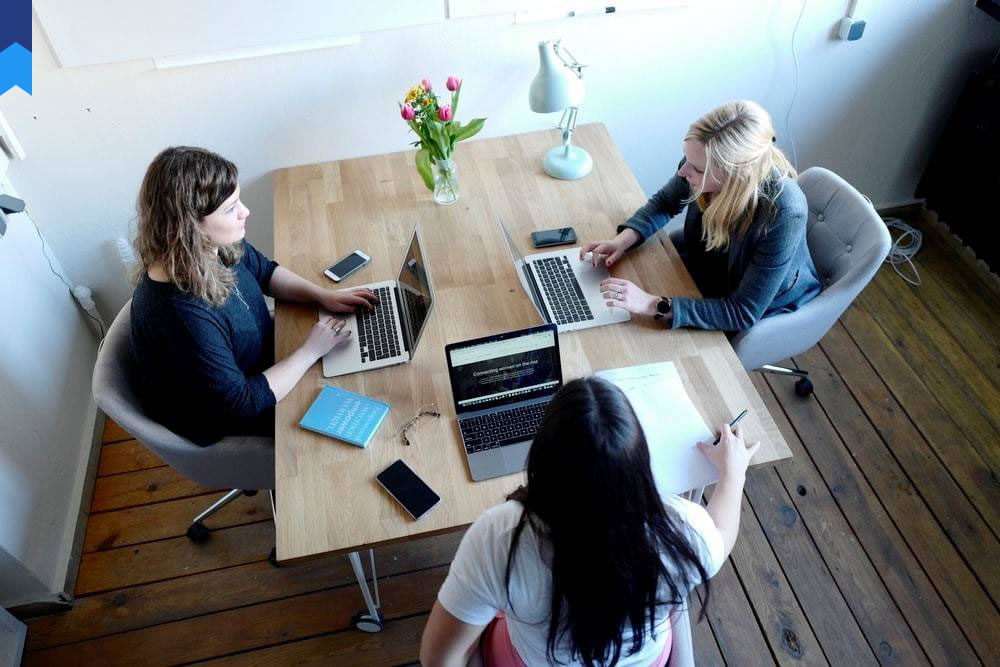
Data-Driven Precision Health: Revolutionizing Patient Care
Data-Driven Precision Health: Revolutionizing Patient Care
Introduction
The healthcare landscape is undergoing a dramatic transformation, driven by the exponential growth of data and the development of sophisticated analytical tools. This shift towards data-driven precision health offers unprecedented opportunities to improve patient outcomes, personalize treatment plans, and enhance the efficiency of healthcare systems. No longer relying solely on generalized approaches, healthcare providers are increasingly leveraging data to understand individual patient needs and tailor interventions accordingly. This approach requires a paradigm shift, moving from reactive care to proactive, predictive healthcare. This article explores various aspects of this revolution, examining how data is reshaping the way we approach health management and patient care.
Data-Driven Diagnostics and Early Detection
Early disease detection is crucial for effective treatment. Data analytics plays a significant role in identifying patterns and anomalies in patient data that may indicate the presence of a disease long before symptoms appear. For example, machine learning algorithms can analyze medical images, such as X-rays and MRIs, to detect subtle abnormalities that might be missed by the human eye, leading to earlier diagnosis of cancers and other life-threatening conditions. A study published in the Journal of Medical Imaging found that AI-powered diagnostic tools were able to detect lung cancer with an accuracy rate of 95%, significantly higher than traditional methods. Another example involves wearable sensors monitoring vital signs. Consistent deviations from a patient's baseline can trigger alerts, prompting timely medical intervention and potentially preventing serious complications. One case study showcases how a hospital implemented a predictive model based on patient data, resulting in a 20% reduction in hospital readmissions for heart failure patients. Furthermore, the ability to analyze large datasets from multiple sources – including electronic health records, genomics, and lifestyle information – allows for the identification of high-risk individuals who may benefit from preventive interventions. The power of data analytics lies in its ability to personalize risk assessment, enabling tailored preventive strategies for each individual.
Furthermore, advancements in genomic sequencing allow for the identification of genetic predispositions to certain diseases. This information can be used to develop personalized prevention strategies, targeting high-risk individuals with tailored interventions. For example, individuals with a high genetic risk for breast cancer can be offered more frequent screening or preventive measures. Similarly, genetic testing can inform decisions about medication selection, ensuring the best possible treatment outcome for each patient. This ability to predict and prevent diseases before they manifest is transformative, leading to improved quality of life and significant cost savings in the long run. The integration of genomics data into larger analytical models further enhances the accuracy of risk prediction and personalized treatment planning, allowing for proactive health management.
Personalized Treatment Plans and Medication Management
Data-driven approaches are revolutionizing treatment plans and medication management. By analyzing patient-specific data, including medical history, genetic information, and lifestyle factors, healthcare professionals can develop personalized treatment plans that are more effective and less likely to have adverse effects. For instance, pharmacogenomics, a field that studies how genes affect a person's response to drugs, is transforming medication management. By analyzing a patient's genetic makeup, doctors can predict how they will respond to specific medications, allowing for optimized dosage and reduced risk of adverse reactions. A leading pharmaceutical company reported a significant improvement in treatment success rates after incorporating pharmacogenomic data into their clinical trials. Another example is the use of data to personalize cancer treatment. Tumour profiling, which involves analyzing the genetic makeup of a tumour, allows for the selection of targeted therapies that specifically attack cancer cells while minimizing harm to healthy cells. This precision approach has led to dramatic improvements in survival rates for certain types of cancer. Moreover, data analytics is crucial for optimizing treatment schedules and managing chronic conditions. Through continuous monitoring of patient data, healthcare providers can adjust treatment plans as needed to ensure optimal outcomes. For instance, diabetes management relies heavily on tracking blood glucose levels and adjusting insulin dosages accordingly. Data analytics tools can facilitate this process, providing real-time feedback and guidance to both patients and healthcare professionals.
Real-world applications demonstrate the impact of personalized medicine. For example, a study published in the New England Journal of Medicine showed that personalized cancer treatment based on genomic data led to a significant improvement in survival rates compared to traditional chemotherapy. Another case study highlighted the successful implementation of a data-driven system for managing chronic heart failure. The system provided patients with personalized feedback and support, resulting in a reduction in hospitalizations and improved quality of life. These examples demonstrate the power of data to transform healthcare, enabling the development of more effective and efficient treatment approaches.
Remote Patient Monitoring and Telehealth
Remote patient monitoring (RPM) and telehealth are rapidly expanding, leveraging data to provide continuous care outside of traditional healthcare settings. Wearable sensors, mobile apps, and other connected devices collect physiological data, such as heart rate, blood pressure, and activity levels, providing real-time insights into a patient's health status. This continuous monitoring allows for early detection of potential problems, enabling prompt intervention and preventing serious complications. A recent study showed that RPM reduced hospital readmissions for patients with chronic heart failure by 25%. Moreover, telehealth platforms enable remote consultations, medication management, and remote monitoring of chronic conditions. Patients can receive care from the comfort of their homes, reducing the burden of travel and improving access to healthcare, especially for those in rural or underserved communities. A case study from a large telehealth provider showed a significant increase in patient satisfaction and improved health outcomes for patients using their remote monitoring services. The continuous flow of data allows clinicians to adjust treatment strategies based on real-time needs, enhancing effectiveness and minimizing risks.
The widespread adoption of RPM and telehealth has been facilitated by advancements in data storage, processing, and analytics. Cloud-based platforms and machine learning algorithms enable the efficient processing of large datasets from multiple sources, providing valuable insights into patient trends and health patterns. Furthermore, the integration of artificial intelligence into RPM systems allows for automated alerts and personalized recommendations, enhancing the effectiveness and efficiency of remote patient management. For instance, AI algorithms can analyze patient data to identify potential risks of deterioration, triggering alerts to healthcare providers and enabling timely intervention. This proactive approach to healthcare is transforming the way chronic conditions are managed, reducing the need for frequent hospital visits and improving patient outcomes.
Data Security and Ethical Considerations
The increasing reliance on data in healthcare necessitates robust data security measures to protect patient privacy and confidentiality. The Health Insurance Portability and Accountability Act (HIPAA) in the US, and similar regulations globally, set stringent standards for protecting patient data. Healthcare organizations must invest in advanced security technologies and implement rigorous data governance policies to ensure compliance and prevent data breaches. Data encryption, access control, and regular security audits are essential for safeguarding patient information. Moreover, ethical considerations are paramount in the use of patient data. Transparency, informed consent, and data minimization are critical principles that must be adhered to. Patients should have control over their data and be informed about how it is being used. The responsible use of AI and machine learning in healthcare requires careful consideration of bias and fairness. Algorithms should be designed and tested to ensure they do not perpetuate existing health disparities. Furthermore, the potential for data misuse or discrimination must be addressed through appropriate regulations and ethical guidelines. A robust framework for data governance is essential to ensure the ethical and responsible use of data in healthcare.
The development of robust data security infrastructure and ethical guidelines is crucial for maintaining trust in data-driven healthcare. Healthcare organizations must invest in comprehensive training programs for their staff to ensure they are aware of data security protocols and ethical considerations. Regular audits and independent reviews can help identify vulnerabilities and ensure compliance with regulations. Furthermore, ongoing collaboration between healthcare providers, technology developers, policymakers, and ethicists is necessary to develop a comprehensive framework for data governance in the age of precision health. This framework should address the ethical challenges of using AI in healthcare, including issues of bias, transparency, and accountability. Building trust and transparency in data management is vital for fostering widespread adoption of data-driven approaches in healthcare.
Conclusion
Data-driven precision health is reshaping healthcare, offering unprecedented opportunities to improve patient outcomes, personalize treatment, and enhance efficiency. From early disease detection through personalized treatment plans and remote patient monitoring, data analytics is transforming the way we approach health management. However, the responsible and ethical implementation of these technologies requires robust data security measures, transparent data governance, and ongoing attention to ethical considerations. As data continues to play an increasingly important role in healthcare, it's crucial that we prioritize patient privacy, build trust, and ensure that these advancements benefit all members of society.