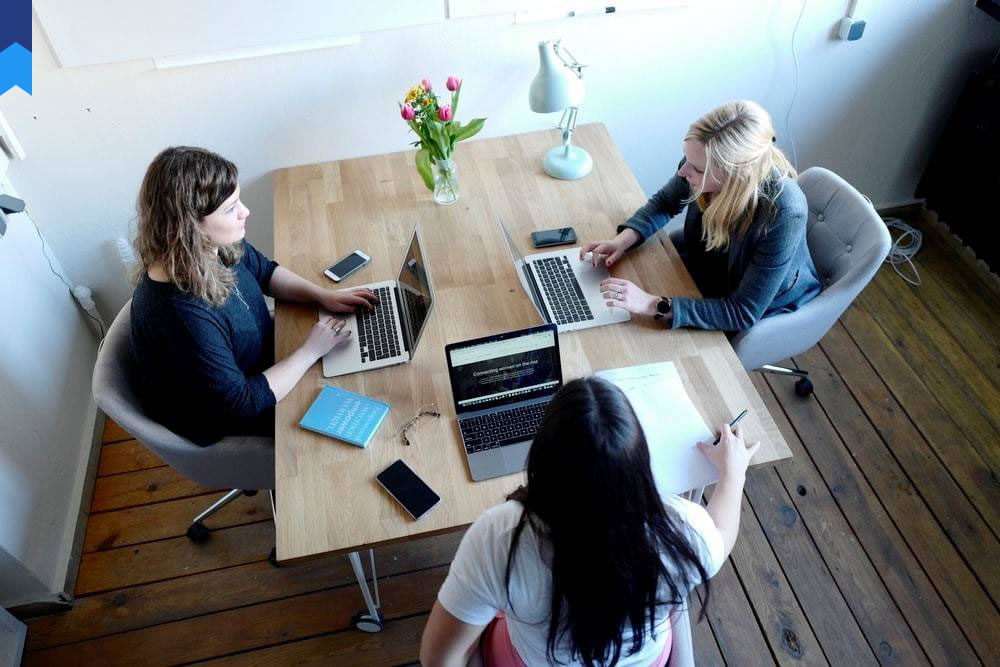
Uncovering The Truth About Data Analytics' Hidden Power
Data analytics is no longer a niche skill; it's a fundamental requirement across all industries. However, many still perceive it as a complex, impenetrable field. This article aims to dispel those myths, unveiling the practical, innovative, and surprisingly accessible aspects of data analytics. We will explore specific techniques, highlight real-world applications, and debunk common misconceptions, empowering you to unlock the true potential of data.
Unlocking the Power of Predictive Modeling
Predictive modeling, a core component of data analytics, uses historical data to forecast future outcomes. This is not about crystal balls; it's about leveraging sophisticated algorithms to identify trends and patterns. For example, a retail company might use predictive modeling to forecast demand for specific products, optimizing inventory management and reducing waste. This involves analyzing sales data, seasonality, marketing campaigns, and external factors like economic indicators. Accurate predictions can significantly improve profitability and resource allocation.
Consider Netflix, a prime example of successful predictive modeling. Their recommendation engine, fueled by vast amounts of user data, predicts what users will enjoy next, driving engagement and subscription retention. Similarly, Amazon utilizes predictive modeling to optimize its logistics and delivery systems, predicting demand spikes and efficiently routing packages for faster delivery times. These companies have built robust data infrastructure to support their complex predictive models, demonstrating the crucial role of technological investment.
The accuracy of predictive modeling relies on data quality and the appropriate choice of algorithms. Inaccurate or incomplete data can lead to flawed predictions. Different algorithms excel in different scenarios, so understanding their strengths and weaknesses is crucial. A business should thoroughly clean and validate its data, and possibly experiment with multiple algorithms to find the best fit for its specific needs. Choosing the right approach is often an iterative process.
Moreover, predictive modeling is constantly evolving. The incorporation of machine learning techniques, like deep learning and neural networks, is improving the accuracy and sophistication of predictive models. These advanced techniques can analyze large datasets and identify complex patterns that traditional methods might miss, leading to even more accurate forecasts and deeper insights. The future of predictive modeling lies in its ability to handle increasing volumes of diverse data sources.
Mastering the Art of Data Visualization
Data visualization isn't just about creating pretty charts; it's about effectively communicating complex information in a clear and engaging way. A well-designed visualization can instantly reveal trends and patterns that would be hidden within raw data. Consider a scenario where a company is analyzing customer demographics. A simple bar chart can clearly show the age distribution of their customer base, while a map visualization could reveal geographical clusters of customers. Data visualization makes data accessible and understandable to a wider audience.
Take the example of Tableau, a widely used data visualization tool, empowers analysts to quickly create interactive dashboards that provide insights into business performance. Similarly, Power BI allows users to connect to a variety of data sources, create engaging reports, and share them with colleagues. These tools have simplified the process of data visualization, making it accessible even to non-technical users. The ability to interact with visualized data is also crucial for fostering greater understanding and insights.
Effective data visualization requires careful consideration of the audience and the message. A visualization designed for executives will differ from one designed for technical analysts. Choosing the right chart type is also critical. Pie charts are great for showing proportions, while line charts are better for illustrating trends over time. The principles of visual design, such as color choice and layout, also play a significant role in creating clear and impactful visualizations.
Data visualization is not a static field; it's constantly evolving. New visualization techniques and tools are emerging, offering more sophisticated and interactive ways to explore data. The integration of augmented reality and virtual reality is also creating new opportunities for immersive data visualization. These innovative approaches will likely play an increasingly important role in future data analysis.
The Ethics of Data Analytics: A Crucial Consideration
The ethical implications of data analytics are paramount. As we collect and analyze increasing amounts of personal data, it's crucial to consider the privacy implications. This involves ensuring data is collected and used responsibly, in compliance with relevant regulations, and with the informed consent of individuals. Ethical data practices build trust and maintain the integrity of the data analysis process.
Consider the Cambridge Analytica scandal, a stark reminder of the potential misuse of personal data. The harvesting and manipulation of user data on Facebook led to serious concerns about privacy and the ethical use of data analytics. This highlighted the importance of robust data governance frameworks and ethical guidelines. Similar incidents underscore the need for transparency and accountability in data handling practices.
Businesses must prioritize data security and protect sensitive information from unauthorized access. This requires implementing strong security measures, including encryption and access controls. Furthermore, data analysts should be trained on ethical data practices, understanding the implications of their work and adhering to ethical guidelines. These practices are not just about compliance but about building trust and maintaining a positive reputation.
Ethical data analytics extends beyond individual privacy to broader societal issues. Bias in algorithms can perpetuate societal inequalities, leading to unfair or discriminatory outcomes. Therefore, it's essential to design and implement algorithms that are fair, transparent, and accountable. This requires careful consideration of potential biases in data and the development of mitigation strategies. The future of data ethics demands a proactive approach, anticipating and addressing potential challenges.
Data Storytelling: Communicating Insights Effectively
Data storytelling is about transforming raw data into compelling narratives that resonate with audiences. It involves combining data analysis with narrative techniques to create engaging and persuasive stories. Instead of just presenting findings, a data storyteller creates a context, establishes a narrative arc, and ultimately leads the audience to a clear understanding and interpretation of the data.
Consider a marketing campaign that utilizes data to understand customer behavior and preferences. Instead of simply reporting on website traffic or conversion rates, a data storyteller would craft a narrative around customer journeys, highlighting key moments of interaction and explaining how these insights can inform future marketing strategies. This approach helps to communicate data insights in a way that inspires action and drives business decisions.
Effective data storytelling involves selecting the right data, framing it within a compelling narrative, and choosing appropriate visual aids. Using data visualization techniques to illustrate key points enhances understanding and engagement. The key is to tell a story that's both informative and persuasive, leading the audience to a clear understanding and appreciation of the data's implications.
Data storytelling is becoming increasingly important in various fields, from business to journalism to scientific research. As data becomes more abundant, the ability to communicate insights effectively through data storytelling is becoming a critical skill. The future of data storytelling will likely see the integration of more sophisticated visualization techniques and interactive storytelling formats, enhancing engagement and comprehension.
Big Data and its Impact on Data Analytics
Big data, characterized by its volume, velocity, and variety, presents both challenges and opportunities for data analytics. The sheer scale of big data requires specialized tools and techniques to process and analyze it effectively. However, the insights derived from big data can be invaluable for businesses and organizations across various sectors. This requires advanced infrastructure and expertise to handle the volume and complexity.
Consider the healthcare industry, where big data is used to analyze patient records, predict disease outbreaks, and personalize treatments. Similarly, financial institutions leverage big data to detect fraud, manage risk, and improve customer service. These applications require robust data processing capabilities and advanced analytical techniques to derive meaningful insights from massive datasets.
The analysis of big data often involves using distributed computing frameworks such as Hadoop and Spark, which allow for parallel processing of large datasets. Machine learning algorithms are also crucial for extracting valuable insights from complex big data. These technologies enable the extraction of complex patterns and relationships that might be missed by traditional methods, leading to more profound insights.
The future of big data analytics is promising, with advancements in cloud computing, artificial intelligence, and machine learning driving innovation in this field. The integration of these technologies will enhance the speed, efficiency, and accuracy of big data analysis, unlocking new possibilities for businesses and organizations across various industries. This ongoing development requires continuous upskilling and adaptation from professionals in the field.
Conclusion
Data analytics is more than just numbers; it’s a powerful tool for understanding the world around us and making data-driven decisions. This article has explored various aspects of data analytics, emphasizing its practical applications and innovative techniques. By understanding predictive modeling, mastering data visualization, embracing ethical considerations, engaging in data storytelling, and harnessing the power of big data, we can unlock the true potential of data. The future of data analytics hinges on our ability to utilize these tools responsibly and creatively, ultimately driving positive change and progress.
The journey into the world of data analytics is ongoing, a constant process of learning, adapting, and innovating. The information presented here provides a solid foundation, but continuous exploration and refinement of techniques are essential for staying ahead in this ever-evolving field. This is not just a technical endeavor but a crucial skillset in navigating the complexities of our increasingly data-driven world. Remember that ethical consideration and responsible use of data are paramount in any data-driven endeavor.