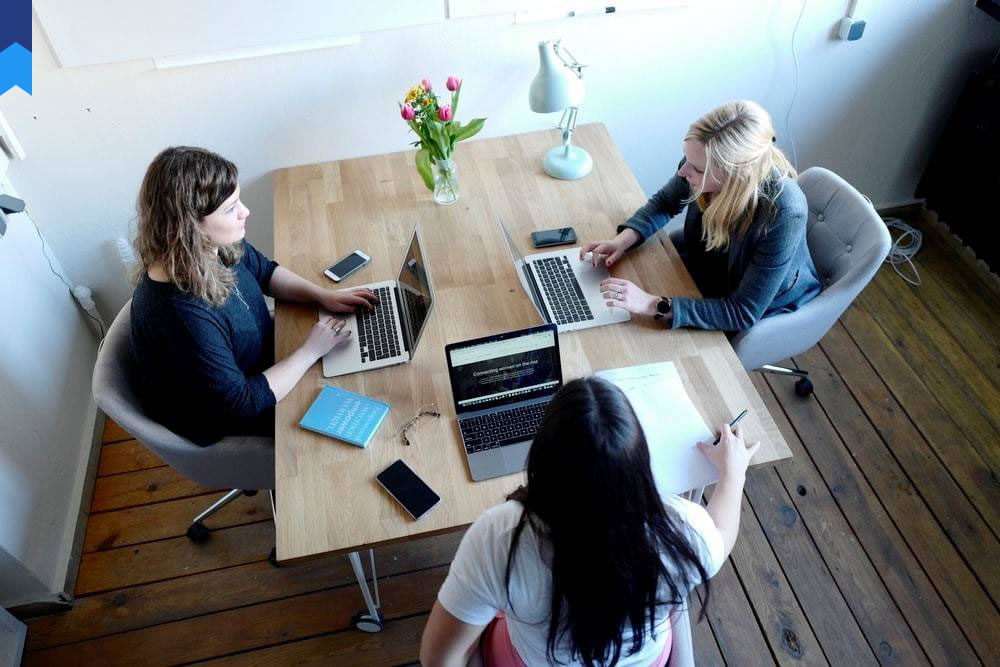
How to Implement Machine Learning for Predictive Maintenance
Predictive maintenance stands at the crossroads of technology and asset management, and with the integration of machine learning, it’s rapidly advancing. Businesses are recognizing the power of data-driven insights, turning towards technological solutions that offer proactive measures. As industries become more interconnected, the harmonization of machine learning with maintenance procedures represents an inevitable and essential evolution, driving efficiency and productivity to new heights.
Understanding Predictive Maintenance
At its core, predictive maintenance foresees potential problems in machinery before they escalate into severe issues. It's analogous to a health check-up for machines; however, instead of reacting to symptoms, it anticipates them. By reducing reactive measures and enhancing proactive interventions, industries can ensure the operational efficiency of machinery while ensuring that any downtime, if at all, is minimal and doesn't significantly disrupt workflows.
The Role of Machine Learning
Machine learning, a subset of artificial intelligence, thrives on data. Algorithms learn and recognise patterns by feeding it historical and real-time data about equipment performance, failures, and operating conditions. As the algorithms receive more data, they refine their predictions, and the system's ability to alert about potential failures improves, ensuring a seamless production process while minimizing unforeseen challenges.
Integrating machine learning into maintenance operations transforms traditional reactive models into proactive ones. This shift not only reduces the wear and tear on equipment but also extends its lifespan, offering significant cost benefits in the long run. Furthermore, the predictive insights provided by such systems allow businesses to schedule maintenance activities during non-peak hours, optimizing operational workflows and reducing disruptions.
Incorporating Data Collection Tools
Data serves as the lifeblood of machine learning. Sensors, IoT devices, and telemetry systems act as the arteries, collecting essential data from machinery such as temperature readings, vibration levels, or other vital metrics. With this influx of continuous data, predictive systems can analyze patterns over prolonged periods, understanding the standard operational behaviour and quickly identifying any anomalies.
This rapid identification enables swift intervention, minimizing potential damage or breakdowns. Advanced algorithms within machine learning models can also forecast when a machine might require maintenance, allowing for proactive measures. Such foresight can lead to cost savings, reduced equipment downtime, and optimized operational efficiency.
Leading the Predictive Maintenance Revolution
Within the predictive maintenance landscape, impeccable IVC technology stands as a testament to innovation and excellence. Its comprehensive approach to CBM, fortified by a team of certified analysts, spans across a multitude of industrial sectors. With a robust nationwide network, IVC underscores the pivotal importance of technology in pioneering maintenance methodologies.
Their unwavering dedication to integrating state-of-the-art solutions positions them at the forefront, crafting bespoke solutions for a wide array of industry requirements. Their proficiency not only aids businesses in streamlining operations but also in significantly reducing downtime. As a result, industries can achieve enhanced productivity and efficiency, further solidifying IVC's influence and impact in the domain of predictive maintenance.
Fine-tuning Machine Learning Models
Machine learning models, while robust, require consistent calibration and tuning. This iterative process involves feeding the model with real-world data, gauging its predictions, refining its accuracy, and then cycling through this process repeatedly. This commitment to continual refinement ensures the machine learning model remains relevant, adjusting to changing data inputs and evolving machinery behaviours, ensuring businesses remain prepared for all eventualities.
Benefits and Outcomes
The integration of machine learning into the maintenance realm doesn't just enhance machinery longevity; it revolutionizes operational processes. The predictive insights afforded by such integration mean resources are utilized more efficiently, maintenance teams can prioritize tasks better, and operational downtime becomes a rarity rather than a recurring challenge. As a result, businesses find themselves better equipped to meet production targets, assure quality, and drive profitability.
Overcoming Implementation Challenges
Transitioning to a machine learning-based predictive maintenance approach is not without its challenges. Organizations might face resistance due to a shift from traditional methods, or there could be a learning curve associated with new technologies. However, with proper training, stakeholder buy-in, and a clear roadmap, these hurdles can be effectively managed. Prioritizing employee education and phased integration can ease the transition, ensuring the organization reaps the benefits without overwhelming its workforce.
As industries delve deeper into the digital age, the blend of machine learning with maintenance becomes not just desirable but essential. This amalgamation signifies an industry on the cusp of a transformative phase, with companies that embrace this change positioned for unparalleled growth. Forward-thinking organizations recognize this trajectory and are investing in tools and technologies that elevate their maintenance strategies, ensuring they stay ahead of the curve in a competitive landscape.
SIIT Courses and Certification
Also Online IT Certification Courses & Online Technical Certificate Programs