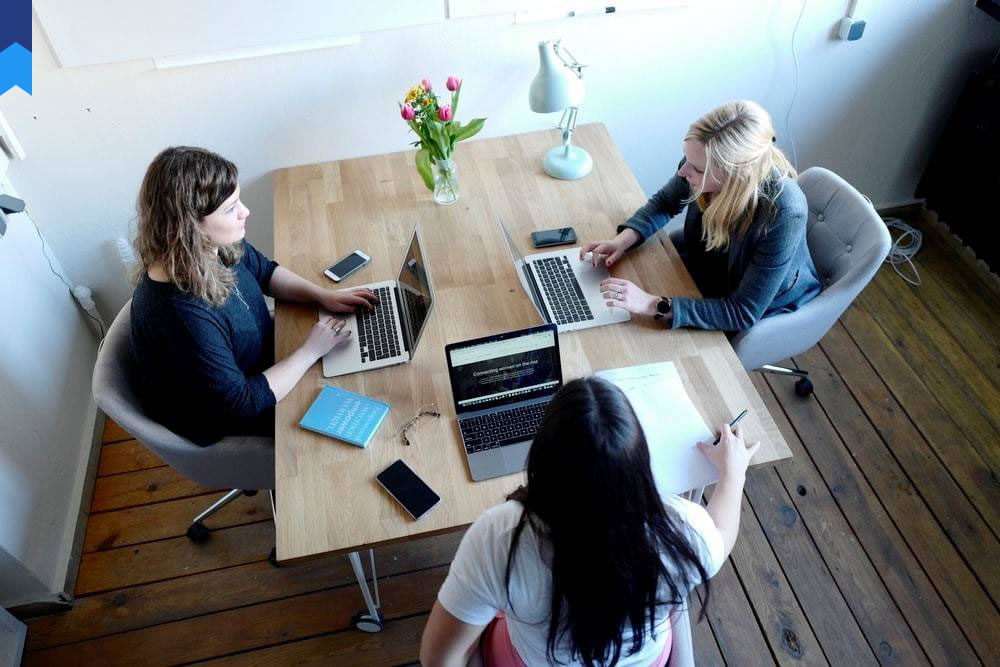
The Intersection of Data Science and AI: Opportunities and Challenges
Data science is a multidisciplinary field where you extract insights and knowledge from data using various techniques, including statistical analysis, machine learning, data mining, and visualization, to make data-driven decisions and solve complex problems. Artificial intelligence (AI), meanwhile, involves simulating human intelligence in machines so they can perform tasks such as analyzing large amounts of data, recognizing patterns, making predictions, and automating tasks.
Data science and AI can converge in various domains and applications. The use of advanced data analysis techniques, machine learning algorithms, and AI technologies can unlock valuable insights and enable intelligent decision-making. The convergence of data science and AI occurs when the application of advanced analytical and computational techniques can leverage data to drive innovation, improve efficiency, and solve complex problems across various industries and domains.
So, the intersection of data science and AI presents exciting opportunities. However, it’s not all sunshine and rainbows, as it also has its share of significant challenges. In this article, we are going to explore these opportunities and challenges.
Opportunities
1.Enhanced Data Processing and Analysis
The combination of data science and AI results in enhanced data processing and analysis capabilities, which enables organizations to derive deeper insights and make more informed decisions. Data science techniques, such as statistical analysis and data mining, are utilized to preprocess and clean large volumes of raw data, ensuring its quality and reliability for analysis.
AI algorithms, including machine learning and deep learning, are then applied to analyze complex datasets and identify patterns, correlations, and trends that may not be apparent through traditional analytical methods.
These advanced AI techniques enable organizations to extract valuable insights from their data, such as customer preferences, market trends, and predictive analytics, allowing for more accurate forecasting and informed decision-making.
Furthermore, the integration of data science and AI facilitates the development of intelligent data processing systems that can automate repetitive tasks and streamline data analysis processes. Machine learning algorithms can be trained to recognize patterns in real-time data streams, enabling organizations to respond to emerging trends more efficiently.
They also play a crucial role in data cleaning and preparation by automating the task of identifying and correcting anomalies, errors, inconsistencies, and missing values in large datasets. This, in turn, streamlines the data preprocessing pipeline and facilitates the transformation of raw data into clean, reliable datasets.
One good example is the e-learning industry, where data science and AI can be utilized to personalize learning experiences for students by analyzing their behavior, preferences, and performance data. With AI in e-learning, algorithms can recommend personalized learning paths, suggest supplementary materials based on individual learning styles, and provide real-time feedback to instructors on student progress, enhancing engagement and improving learning outcomes.
Ultimately, the combination of data science and AI enhances data processing and analysis by leveraging advanced computational techniques to extract actionable insights from large and complex datasets, empowering organizations to drive innovation, optimize operations, and gain a competitive edge in their respective industries.
2.Advanced Predictive Analytics and Modeling
When data science and AI intersect, it opens up new avenues for advanced predictive analytics and modeling, which makes it easy for organizations to anticipate future trends, outcomes, and behaviors with greater accuracy.
Data science techniques, such as regression analysis and time series forecasting, provide a foundation for predictive modeling by identifying relationships between variables and extrapolating future trends based on historical data.
AI algorithms enhance predictive analytics by enabling computers to learn from data patterns and make predictions without explicit programming. These advanced AI models can analyze large and complex datasets to uncover hidden patterns and correlations, resulting in more accurate and reliable predictions across various domains, including finance, healthcare, and marketing.
In healthcare, for example, data science techniques are used to analyze medical data, such as patient records and imaging data, to identify patterns and trends that can assist in diagnosis, treatment planning, and personalized medicine. AI algorithms are then utilized to develop predictive models and decision support systems that can accurately predict patient outcomes and recommend treatment strategies.
So it doesn’t come as a surprise that in 2021, the global healthcare AI market was worth around $11 billion and was forecast to reach almost $188 billion by 2030. This reflects a compound annual growth rate of 37% from 2022 to 2030.
Moreover, the integration of data science and AI enables the development of sophisticated predictive models that can adapt and learn from new data inputs, improving their accuracy and performance over time. Machine learning algorithms, such as neural networks and ensemble methods, can be trained on large datasets to identify complex patterns and make predictions with high precision.
Additionally, AI-driven predictive analytics platforms can automate the model-building process, from data preprocessing to model selection and evaluation, accelerating the development of predictive models and reducing the need for manual intervention.
By harnessing the power of data science and AI, organizations can leverage advanced predictive analytics and modeling techniques to gain valuable insights, optimize decision-making processes, and drive business growth in an increasingly competitive and data-driven world.
3.Automation and Decision-Making
The combination of data science and AI significantly contributes to automation and decision-making processes across various industries. Data science techniques, such as data mining and statistical analysis, enable organizations to extract valuable insights from large datasets, providing the foundation for informed decision-making.
AI algorithms enhance automation by enabling computers to learn from data patterns and make predictions or decisions autonomously. By integrating data science and AI, organizations can automate repetitive tasks and optimize resource allocation, leading to increased efficiency and productivity.
Take for example AI-powered tax automation tools, which can extract relevant information from financial documents, apply tax rules and regulations, and generate accurate tax returns, reducing manual errors and streamlining the tax filing process for individuals and businesses. Because of the benefits of tax automation tools, the global tax automation software market size reached $18.4 billion in 2023 and is expected to reach $44 billion by 2032.
The synergy between data science and AI empowers organizations to automate processes, leverage data-driven insights, and make informed decisions, ultimately driving innovation and competitive advantage in the digital age.
Challenges
1.Data Quality and Bias
The quality and quantity of data play a crucial role in determining the performance of AI models. High-quality data is accurate, complete, and representative of the problem domain enables AI algorithms to learn meaningful patterns and make reliable predictions.
Conversely, poor-quality data containing errors, inconsistencies, or biases can lead to inaccurate or biased AI outputs. Additionally, the quantity of data available for training AI models directly impacts their performance, as larger datasets provide more information for the algorithms to learn from, leading to more robust and generalizable models.
However, it's essential to strike a balance between quality and quantity, as an abundance of low-quality data may produce unreliable results, while a scarcity of high-quality data may limit the effectiveness of AI models.
One of the significant challenges in AI-driven decision-making is data bias, which occurs when the training data used to develop AI models is not representative of the population or contains inherent biases. Data bias can result in AI algorithms producing unfair or discriminatory outcomes, particularly in sensitive domains such as hiring, lending, and criminal justice.
To mitigate data bias, organizations can implement strategies such as using diverse datasets that reflect the diversity of the population, employing human oversight to identify and correct biases in the data, and regularly auditing AI models for fairness and equity.
Additionally, transparency and accountability in the AI development process are essential to ensure that biases are identified and addressed proactively, fostering trust and confidence in AI-driven decision-making systems.
2.Explainability and Transparency
The challenge of understanding how complex AI models arrive at their decisions, often referred to as the black box problem, poses significant obstacles to trust, transparency, and accountability in AI systems. Many modern AI algorithms, such as deep neural networks, are highly complex and comprise millions of parameters, making it difficult for humans to comprehend the reasoning behind their decisions.
This lack of transparency raises concerns about the potential for bias, discrimination, and errors in AI outputs, particularly in critical domains such as healthcare, finance, and criminal justice. Without visibility into the decision-making process of AI models, stakeholders may be hesitant to rely on their recommendations or conclusions, undermining their effectiveness and utility in real-world applications.
Explainability, or the ability to understand and interpret the reasoning behind AI decisions, is crucial for building trust and ensuring ethical AI development. By providing insights into how AI models arrive at their predictions or classifications, explainability enables stakeholders to assess the reliability, fairness, and potential risks associated with AI systems.
3.Job Displacement and Ethical Concerns
The potential for AI to automate tasks that are currently performed by humans raises concerns about job displacement and economic inequality. As AI technologies continue to advance, they can be deployed to replace human workers in various industries. This would lead to unemployment and exacerbate socioeconomic disparities.
Additionally, the rapid adoption of AI in decision-making processes, such as hiring, lending, and criminal justice, raises ethical concerns regarding bias, discrimination, and accountability. Issues such as data privacy, algorithmic bias, and the weaponization of AI further underscore the need for responsible AI development and regulation.
It is crucial to prioritize the development of human-centered AI solutions that prioritize fairness, transparency, and accountability, while also ensuring that AI technologies are deployed ethically and in ways that benefit society as a whole.
Conclusion
The intersection of data science and AI presents immense opportunities for organizations to leverage data-driven insights and intelligent automation to drive innovation and improve decision-making. With advanced AI algorithms, organizations can extract valuable insights from large and complex datasets, leading to more accurate predictions and better-informed decisions across various domains.
SIIT Courses and Certification
Also Online IT Certification Courses & Online Technical Certificate Programs