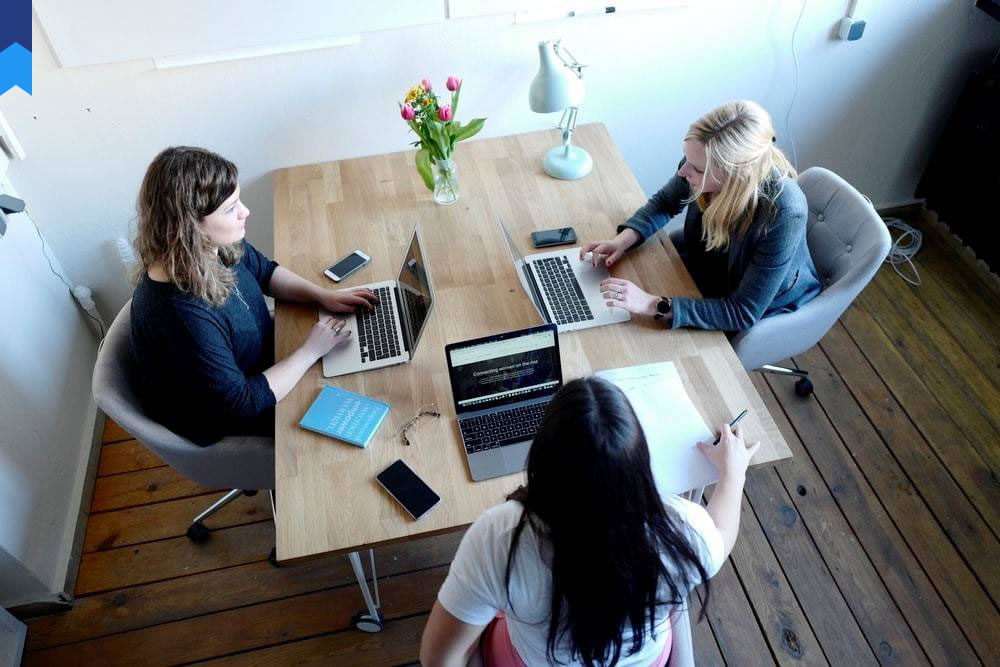
Beyond Traditional Surveys: A New Approach To Tech Market Research
Market research is crucial for success in the ever-evolving tech landscape. However, relying solely on traditional methods like surveys often fails to capture the nuances and complexities of the modern tech consumer. This article explores a new, more comprehensive approach, going beyond traditional limitations to provide a truly insightful perspective.
Understanding the Limitations of Traditional Surveys
Traditional surveys, while offering a structured approach, often fall short in several key areas. They can be overly simplistic, failing to delve into the underlying motivations and emotional drivers behind consumer behavior. For example, a simple question about smartphone preference might not uncover the reasons behind that preference, such as brand loyalty, specific feature requirements, or social influence. This lack of depth limits the actionable insights gained from such surveys.
Furthermore, traditional surveys often suffer from sampling biases. If the sample population doesn't accurately reflect the target market, the results will be skewed and unreliable. For instance, a survey conducted solely online might exclude a significant portion of the population who are not comfortable or do not have access to the internet. This can lead to flawed conclusions and misinformed strategic decisions.
Another significant limitation is the inherent limitations of self-reporting. Participants may not accurately reflect their own behavior, influenced by social desirability bias or a lack of self-awareness. They might overstate their tech savviness or underreport their concerns about privacy, leading to an inaccurate representation of reality.
Case Study 1: A major tech company relied heavily on traditional surveys to gauge consumer interest in a new virtual reality headset. The results indicated high interest, but the product launch was a dismal failure. Further analysis revealed that the survey sample was biased towards early adopters who were already enthusiastic about VR, not representative of the broader market.
Case Study 2: A mobile phone manufacturer conducted a survey asking users about their preferred features. The overwhelming response favored a larger battery. However, post-launch analysis showed that user complaints focused on the phone's increased size and weight, highlighting a disconnect between stated preference and actual usage patterns.
The rigidity of traditional survey design also limits the ability to explore unexpected or emergent themes. Researchers are often constrained by pre-defined questions, missing out on valuable insights that arise organically during interactions with consumers. To address these limitations, a multi-faceted approach is needed.
Traditional methods often fail to capture the dynamic nature of the tech market, where trends evolve rapidly and consumer preferences shift unpredictably. The static nature of many survey designs cannot adapt to these changes, resulting in data that quickly becomes obsolete.
Another crucial aspect is the growing sophistication of consumer behavior in the tech industry. Consumers are more aware of marketing tactics and may be less inclined to provide honest feedback in structured survey formats, especially if they perceive it as intrusive or manipulative. Therefore, new approaches are vital to uncover genuine insights.
The increasing complexity of technology itself necessitates a more nuanced approach to market research. Analyzing the intricacies of software functionality, user experience, and hardware performance requires methods beyond simple rating scales or multiple-choice questions.
In the age of big data, traditional surveys are often insufficient for analyzing large and complex datasets generated by user behavior on digital platforms. More sophisticated analytical techniques are needed to extract meaningful insights from these data streams.
Leveraging Ethnographic Research and User Observation
Ethnographic research offers a powerful alternative to traditional surveys. By immersing themselves in the target market's environment, researchers gain a deep understanding of consumer behavior in context. This approach emphasizes observation and interaction rather than reliance on self-reported data. By observing users in their natural settings—homes, workplaces, or even online communities—researchers can capture authentic and unfiltered insights into how technology is integrated into daily life.
For instance, observing how users interact with a specific app can reveal usability issues, unexpected use cases, and emotional responses that would be missed in a standard survey. Researchers might witness users struggling with a specific feature or discovering unexpected functionalities. This first-hand experience allows for a deeper understanding of the user experience than self-reported data alone can provide.
User observation, a key component of ethnographic research, allows for the detailed examination of how users engage with technology. Researchers can observe not just what users do, but how they do it—their body language, their expressions, their frustrations, and their moments of delight. This qualitative data provides rich context for quantitative findings.
Case Study 3: A social media platform employed ethnographic research to understand how users interact with their newsfeed algorithm. By observing users in their homes and workplaces, researchers gained insights into how users prioritize information, how they react to different types of content, and how they use the platform as a tool for social connection.
Case Study 4: A game development company used ethnographic methods to study how gamers interact with their new game. Researchers observed gaming sessions, capturing player reactions and interactions, leading to design modifications that enhanced the overall gameplay experience and user satisfaction.
The insights derived from ethnographic research are invaluable in informing product design, marketing strategies, and user experience optimization. However, ethnographic research is resource-intensive and time-consuming, requiring skilled researchers and careful planning. While costly, the detailed and nuanced insights often justify the investment.
Ethnographic studies often involve extensive field work and data analysis. The depth of understanding gained, however, far outweighs the challenges involved. This approach can significantly reduce the risk of product failure by ensuring alignment between product design and actual user needs and behaviors.
Combining ethnographic research with other methods, such as surveys and A/B testing, can provide a comprehensive and robust approach to market research. The rich qualitative data from ethnographic studies can contextualize and enrich the quantitative findings from other methods.
Ethical considerations are paramount in conducting ethnographic research. Researchers must obtain informed consent from participants and maintain their privacy and anonymity. They also need to be mindful of their own biases and avoid influencing the behavior of participants.
By emphasizing observation and user interaction, ethnographic research provides a holistic view of the relationship between technology and consumers, a perspective that is increasingly crucial in today's market.
Harnessing the Power of Big Data Analytics
The explosion of digital data offers unprecedented opportunities for market research in the tech industry. Big data analytics provides the tools to analyze massive datasets from various sources, including user activity on websites and apps, social media interactions, and online purchasing behavior. This data can reveal patterns and insights that would be impossible to discover through traditional methods.
One key application of big data analytics is predictive modeling. By analyzing past user behavior, researchers can predict future trends and anticipate consumer needs. For example, analyzing website traffic data can help predict which features are likely to be most popular, guiding product development and marketing efforts.
Another valuable use of big data is sentiment analysis, which involves analyzing social media posts, online reviews, and other text data to gauge public opinion on products, brands, and technologies. This can provide early warnings of potential issues and help proactively address negative sentiment.
Case Study 5: A streaming service used big data analytics to analyze viewing habits of its subscribers. This allowed them to personalize content recommendations, leading to increased engagement and retention rates.
Case Study 6: An e-commerce company leveraged big data to analyze customer purchase history and website browsing behavior, allowing them to tailor their marketing campaigns and personalize their recommendations, resulting in a significant increase in sales.
The challenge with big data analytics lies in effectively managing and interpreting massive datasets. This requires specialized skills and sophisticated tools. The data needs to be cleaned, organized, and transformed into a usable format before meaningful insights can be extracted.
Data visualization techniques are critical for effectively communicating the findings of big data analysis. Presenting complex data in an accessible and understandable format is essential for decision-making.
Ethical considerations related to data privacy and security are paramount when working with big data. Researchers must adhere to strict data protection regulations and ensure that user data is handled responsibly and ethically.
Big data analytics offers a powerful lens into consumer behavior and market trends. However, it's crucial to remember that data is not an end in itself; its value lies in the insights it generates and how those insights are applied to inform strategic decisions.
The combination of big data analytics and other qualitative research methods can provide a comprehensive and robust understanding of the tech market. Big data can identify patterns and trends, while qualitative methods can provide the context and explanation for those patterns.
Future trends in big data analytics include the increasing use of artificial intelligence (AI) and machine learning (ML) techniques to automate data analysis and enhance predictive modeling capabilities. This will further enhance the precision and efficiency of market research.
Integrating A/B Testing and Experimentation
A/B testing is a powerful method for evaluating the effectiveness of different design choices, marketing messages, or features. By presenting two or more variations to users and measuring their responses, researchers can identify which variation performs best. This empirical approach provides valuable data to inform product development and marketing decisions.
For example, A/B testing can be used to compare the effectiveness of different website designs, app features, or marketing emails. By measuring key metrics such as click-through rates, conversion rates, and engagement time, researchers can determine which variation leads to better results.
A/B testing is particularly valuable in the fast-paced tech industry, where rapid iteration and continuous improvement are essential. It allows for continuous optimization of products and services based on real-world user feedback.
Case Study 7: A mobile gaming company used A/B testing to compare two different versions of a new game's interface. One version resulted in a significantly higher player retention rate, leading to the adoption of that design.
Case Study 8: An e-commerce website used A/B testing to optimize its checkout process. Different variations of the checkout page were tested, leading to a reduction in cart abandonment rates and an increase in sales conversions.
Effective A/B testing requires careful planning and execution. It is crucial to define clear hypotheses, select appropriate metrics, and ensure that the test is statistically sound. The test should also be run for a sufficient duration to ensure reliable results.
A/B testing is not without limitations. It may not reveal underlying reasons for the observed differences between variations. Therefore, it's often valuable to combine A/B testing with qualitative methods to gain a deeper understanding of why certain variations perform better than others.
The increasing use of A/B testing reflects a shift towards a more data-driven approach to product development and marketing. This evidence-based approach enhances efficiency and effectiveness.
A/B testing is most effective when combined with a comprehensive understanding of the target audience. This allows researchers to tailor the variations to the specific needs and preferences of the users.
The future of A/B testing likely involves the increasing use of AI and ML to automate the process and optimize testing strategies. This will lead to faster and more efficient results.
By combining A/B testing with qualitative research methods, businesses can gain a rich and nuanced understanding of user behavior and optimize their products and services accordingly.
Combining Qualitative and Quantitative Methods for a Holistic View
The most effective approach to tech market research involves a mixed-methods strategy, integrating qualitative and quantitative approaches. This approach leverages the strengths of each method to provide a comprehensive and nuanced understanding of the target market. Quantitative methods, such as surveys and A/B testing, provide large-scale data that can identify trends and patterns. Qualitative methods, such as ethnographic research and in-depth interviews, provide rich contextual information and detailed insights into user motivations and behaviors.
Combining these methods allows researchers to analyze large-scale trends and then delve into the underlying reasons for those trends. For instance, quantitative data might reveal a decline in user engagement with a particular app feature. Qualitative research could then uncover the reasons behind this decline—perhaps users find the feature confusing or irrelevant to their needs.
This integrated approach enhances the validity and reliability of the research findings. Quantitative data can provide statistical support for qualitative findings, while qualitative data can add depth and meaning to quantitative results.
Case Study 9: A software company used a mixed-methods approach to evaluate user satisfaction with its new software. Quantitative surveys measured overall satisfaction, while qualitative interviews explored the reasons behind users' ratings and provided detailed feedback on specific features.
Case Study 10: A social media company combined large-scale surveys with focus groups to understand user perceptions of its privacy policies. The surveys provided data on overall trust levels, while the focus groups explored the reasons behind those perceptions and identified areas for improvement.
The key to successful mixed-methods research lies in careful planning and integration of the different methods. The research questions should guide the selection of methods, and the findings from each method should be analyzed and interpreted together to provide a holistic understanding.
Mixed-methods research allows researchers to triangulate their findings, strengthening the reliability and validity of their conclusions. By using multiple methods, researchers can reduce the risk of bias and obtain a more complete picture of the research topic.
The integrated approach is crucial for navigating the complexities of the tech market, where trends evolve rapidly and consumer behavior is influenced by a multitude of factors. A comprehensive approach is essential for informed decision-making.
The future of tech market research likely involves an even greater integration of quantitative and qualitative methods, leveraging the power of big data and advanced analytical techniques to uncover deeper insights into consumer behavior and market trends.
By adopting a comprehensive, multi-faceted approach, tech companies can gain a crucial advantage, making more informed decisions, optimizing their products, and ultimately, achieving greater success in the competitive tech market.
Conclusion
Moving beyond traditional survey-based market research is crucial for navigating the complexities of the modern tech landscape. By embracing a more nuanced approach that combines ethnographic research, big data analytics, A/B testing, and a mixed-methods strategy, tech companies can gain a deeper and more comprehensive understanding of their target markets. This understanding allows for more informed product development, targeted marketing campaigns, and ultimately, greater success in a rapidly evolving industry. This multi-faceted approach allows for a more accurate reflection of consumer behavior, leading to better decision-making and improved outcomes.
The adoption of these new strategies will empower businesses to not only react to changes in the market but to anticipate and shape them. Investing in this more holistic research methodology is not simply an expense but a strategic imperative for continued growth and competitiveness.
The integration of various research approaches minimizes biases inherent in individual methods, providing a more complete picture of consumer preferences and behaviors. Ultimately, this translates to more robust product development and more effective marketing strategies, leading to a greater return on investment in market research.