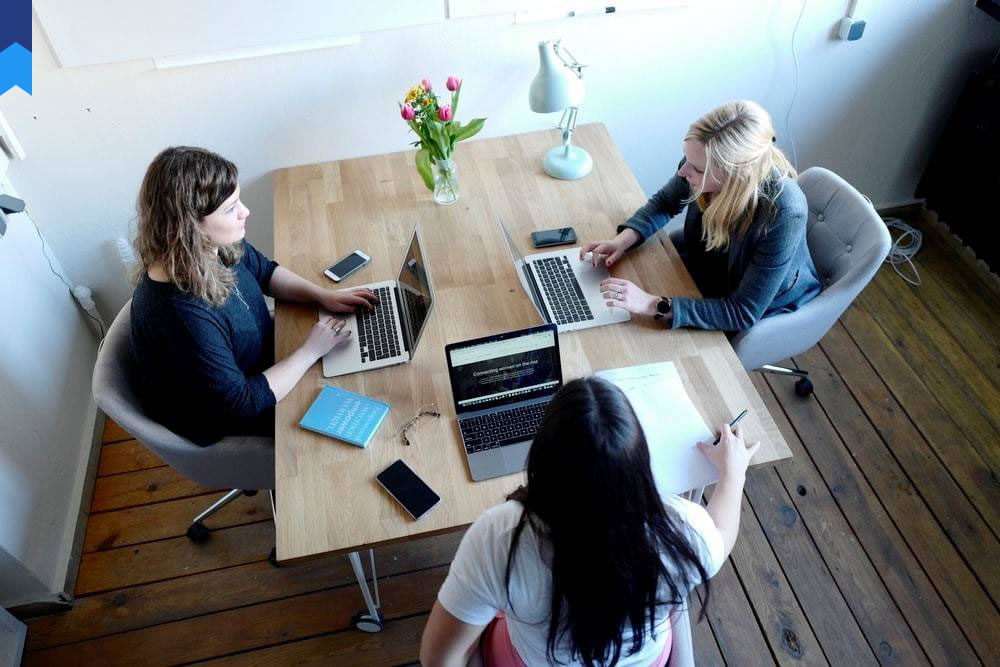
Data-Driven Conversion Optimization Methods
Digital marketing is evolving rapidly. What was cutting-edge yesterday may be obsolete tomorrow. To remain competitive, businesses must embrace a data-driven approach to conversion optimization, constantly analyzing results and adapting strategies. This means moving beyond intuition and guesswork, and instead leveraging the power of data analytics to identify opportunities for improvement and drive tangible results. This article explores specific, practical, and innovative methods for achieving superior conversion rates.
Understanding Your Audience Through Data
Effective conversion optimization begins with a thorough understanding of your target audience. Data analytics provides invaluable insights into user behavior, preferences, and motivations. By analyzing website traffic, social media engagement, and email marketing data, you can create detailed customer personas that inform every aspect of your marketing strategy. For example, analyzing Google Analytics data can reveal which pages have high bounce rates, indicating potential usability issues or irrelevant content. This allows for targeted improvements to the user experience.
Consider a case study of a major e-commerce company that used heatmap technology to analyze user interactions on its product pages. The heatmaps revealed that many users were ignoring the "add to cart" button, leading to a low conversion rate. By redesigning the page to make the button more prominent, the company saw a significant increase in conversions. Another example involves A/B testing different call-to-action buttons to see which produces the highest click-through rate. Data can pinpoint exactly which variation performs better, eliminating guesswork and improving ROI.
By leveraging data on demographics, interests, and online behavior, you can create hyper-targeted advertising campaigns that reach the right audience with the right message at the right time. This targeted approach increases the likelihood of engagement and conversion. Furthermore, analyzing customer segmentation data can reveal distinct groups within your audience, each with unique needs and preferences. This allows for the creation of personalized marketing messages that resonate with each segment, resulting in higher conversion rates. In essence, data allows you to shift from a generic approach to highly personalized engagement.
Data-driven personalization isn't just about demographics; it encompasses behavior as well. For example, website behavior tracking can reveal which products users are viewing, adding to their carts, and ultimately purchasing. This allows you to recommend related products or offer personalized discounts, further optimizing the shopping journey and increasing the likelihood of conversion. Analyzing customer feedback through surveys and reviews provides crucial qualitative insights that complement quantitative data. Understanding what customers value and what frustrates them is invaluable for optimizing the customer journey and improving satisfaction.
Optimizing the User Experience with Data-Driven Insights
User experience (UX) plays a pivotal role in conversion optimization. Data analytics provides a wealth of information to identify areas for UX improvement. Analyzing website analytics reveals patterns in user behavior, such as high bounce rates on specific pages or long loading times. Addressing these issues directly improves the user experience and contributes positively to conversions. For instance, a slow-loading website can lead to high bounce rates and lost sales. Optimizing website speed through image compression, code optimization, and efficient hosting can significantly enhance the user experience and improve conversion rates. In addition to website speed, usability is crucial for conversions.
Case study: A company redesigned its website navigation, based on data showing users struggled to find specific products. The changes resulted in a significant increase in sales. Another case study involved a business that analyzed user heatmaps to discover that the placement of its call-to-action button was hindering conversions. By adjusting the placement, the business saw a significant rise in click-through rates. A/B testing different versions of a website, landing page, or checkout process allows for data-driven decision-making. For example, testing different variations of a website's headline, imagery, or call-to-action button helps determine which version resonates most with users and produces the highest conversion rate. Testing variations of page layouts, navigation menus, and form designs provides continuous opportunities for improvement.
Using data to improve website architecture ensures a seamless flow for users. For instance, data could indicate a high drop-off rate at a particular stage of the checkout process. Analyzing this data helps identify what is causing the drop-off (complicated forms, slow loading times, etc.) and provides evidence to implement targeted improvements. Analyzing user behavior can reveal preferences for certain types of content or formats. This allows businesses to customize content to better meet user needs and improve engagement.
The use of session recording tools offers valuable insights into the user experience. These tools record user interactions on a website, allowing businesses to observe user behavior in real-time. This provides a deep understanding of how users navigate the website and helps identify areas where the experience can be improved. It can highlight issues that would be difficult to uncover using website analytics alone. This detailed, visual feedback allows for targeted improvements that otherwise would have been missed.
Leveraging A/B Testing and Multivariate Testing for Optimization
A/B testing and multivariate testing are crucial data-driven methods for conversion optimization. A/B testing involves comparing two versions of a webpage or element to determine which performs better. This could involve testing different headlines, images, or call-to-action buttons. Multivariate testing, on the other hand, allows for the testing of multiple variations of several elements simultaneously. This provides a more comprehensive understanding of how different elements interact with each other and influence conversions. For example, a business might test different headline variations, image choices, and call-to-action button colors simultaneously to find the optimal combination for maximum conversions.
Consider a case study of a SaaS company that A/B tested two versions of its pricing page. One version emphasized the value proposition, while the other focused on affordability. The testing revealed that the value-focused version resulted in a significantly higher conversion rate. In another case, a retail business used multivariate testing to optimize its product pages. By testing different combinations of images, descriptions, and pricing, the business determined the most effective combination for driving sales. Using a robust A/B testing platform ensures accurate and reliable results. A well-designed experiment controls for extraneous variables, minimizing bias and maximizing the validity of results.
Continuous A/B testing allows for ongoing optimization. Instead of implementing changes based on assumptions or intuition, regular A/B testing enables data-driven adjustments, leading to consistent improvement in conversion rates. The insights gathered from A/B testing should inform future marketing decisions and strategies. This iterative process of testing, analyzing, and improving leads to a constantly optimized marketing strategy. Data analysis should be comprehensive, including statistical significance analysis to ensure results are reliable and not due to random chance. It’s important to interpret data accurately and avoid drawing premature conclusions.
It’s essential to carefully define goals and metrics for A/B tests. What exactly are you hoping to improve? Conversion rates? Click-through rates? Sign-up rates? Clearly defined goals ensure that the results of the test are relevant to your overall objectives. Choosing the right sample size for A/B tests ensures that the results are statistically significant and not just random fluctuations. Too small a sample size can lead to inaccurate conclusions, while too large a sample size can be inefficient.
Harnessing the Power of Predictive Analytics for Proactive Optimization
Predictive analytics utilizes historical data to forecast future outcomes and identify potential problems before they occur. In the context of conversion optimization, this allows marketers to anticipate user behavior and proactively address potential obstacles. For example, analyzing past website traffic data can predict future demand spikes, allowing for proactive scaling of server resources to prevent website crashes or slowdowns during peak periods. Predictive models can analyze various data points to forecast customer churn risk. This allows businesses to proactively reach out to at-risk customers with targeted retention offers.
One case study involved an e-commerce company that used predictive analytics to identify products likely to be popular during a specific season. This allowed them to proactively increase inventory levels and avoid stockouts, leading to increased sales. Another case study featured a subscription service that used predictive modeling to identify users at risk of canceling their subscriptions. This enabled them to provide personalized incentives to retain those customers and reduce churn.
By employing machine learning algorithms, marketers can create sophisticated models that identify complex patterns in user behavior and predict future outcomes with greater accuracy. This allows for highly targeted interventions to improve the customer experience and drive conversions. These models can be used to personalize website content, advertising messages, and email campaigns, leading to improved engagement and conversion rates. For instance, models can personalize recommendations for products or services based on user preferences and past purchase history.
Predictive analytics can also identify opportunities for cross-selling and upselling. By analyzing past purchase data, predictive models can identify customers likely to be interested in additional products or services, enabling businesses to proactively offer relevant recommendations and increase revenue. Real-time predictive analytics can provide immediate insights into user behavior, enabling businesses to respond swiftly to changes in market dynamics and adjust their strategies accordingly. This agility is crucial in today's dynamic digital landscape.
Integrating Data Across Different Marketing Channels for Holistic Optimization
Effective conversion optimization requires a holistic approach that integrates data from different marketing channels. By connecting data from various sources – such as website analytics, social media analytics, email marketing platforms, and CRM systems – marketers gain a comprehensive understanding of the entire customer journey. This allows for a more coordinated and effective marketing strategy. For example, connecting website analytics data with social media analytics data can reveal which social media campaigns are driving the most website traffic and conversions. This information can be used to optimize future social media campaigns and allocate resources more effectively.
One company integrated its email marketing data with its website analytics data to identify which email campaigns were leading to the highest website engagement and conversions. This enabled them to optimize their email marketing strategy and improve the overall return on investment. Another company used a unified marketing platform to track customer interactions across different channels, providing a complete view of the customer journey. This unified view enabled them to personalize customer interactions and improve conversions.
A robust marketing automation platform plays a crucial role in integrating data from various channels. These platforms automate marketing tasks, personalize customer interactions, and provide real-time insights into campaign performance. Integrating customer relationship management (CRM) systems with marketing automation platforms enables a more personalized approach. This ensures that customer data is accessible across different departments, enhancing communication and improving collaboration.
This holistic approach enables the creation of a single view of the customer, providing a more complete picture of their interactions with the brand across all channels. This allows for personalized messaging and targeted offers that resonate with individual customers, ultimately improving conversion rates. Data integration enables the identification of cross-channel attribution models. This reveals the true contribution of each channel to conversions, enabling businesses to allocate resources effectively. Accurate attribution is essential for measuring the effectiveness of marketing efforts and justifying investments in different channels.
Conclusion
Data-driven conversion optimization is no longer a luxury; it's a necessity for businesses seeking to thrive in today's competitive digital landscape. By embracing the methods discussed above—understanding your audience through data, optimizing the user experience, leveraging A/B and multivariate testing, harnessing predictive analytics, and integrating data across channels—businesses can unlock significant opportunities for growth and achieve superior conversion rates. The key is to move beyond reactive adjustments and embrace a proactive, data-driven approach that anticipates user needs and continuously refines the customer experience. Continuous monitoring and adaptation are essential for staying ahead of the curve and maximizing the return on marketing investments. The ability to analyze data, understand its implications, and translate insights into actionable strategies is paramount to success.