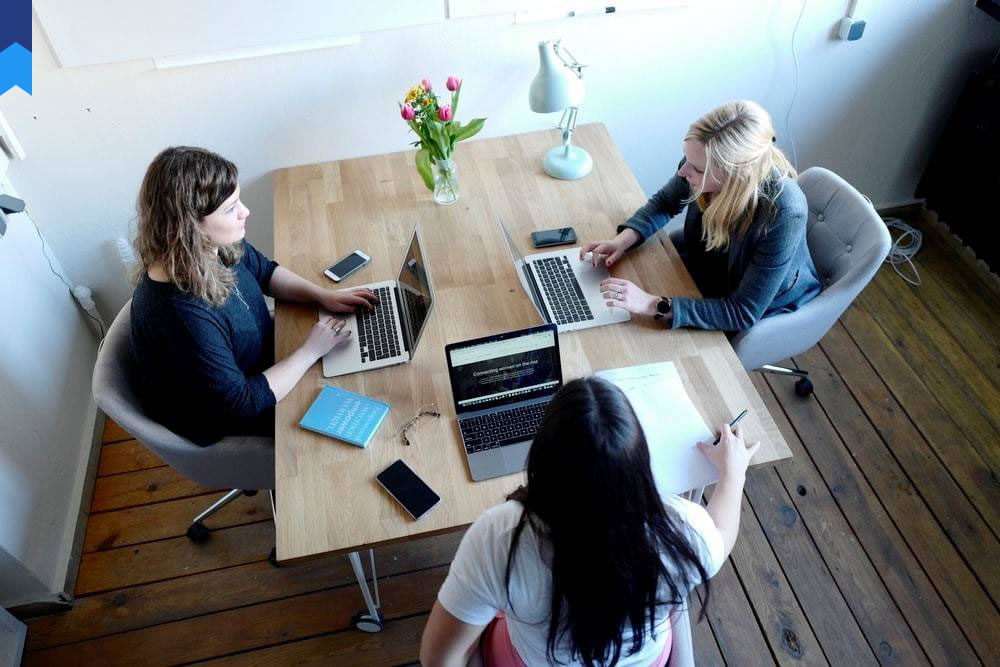
Data-Driven Electrical Troubleshooting Methods
Introduction
Troubleshooting electrical issues can be a daunting task, often involving time-consuming trial-and-error methods. This article presents a data-driven approach, leveraging modern technologies and analytical techniques to streamline the process and improve accuracy. We move beyond traditional guesswork, embracing a more efficient and reliable system. By integrating data analysis and predictive modeling, we can identify potential problems before they arise, leading to significant cost and time savings in both residential and industrial settings. This new approach utilizes readily available data points and presents a step-by-step guide to help both beginners and experienced electricians diagnose problems faster and more accurately. We will explore practical applications of these methods, focusing on specific, real-world scenarios.
Data Acquisition and Sensor Technology
The foundation of data-driven electrical troubleshooting lies in effective data acquisition. This involves strategically placing sensors to monitor key parameters within electrical systems. Smart sensors, capable of wireless communication and real-time data transmission, are increasingly prevalent. These devices can measure voltage, current, power factor, temperature, and other crucial metrics. For instance, installing current transformers (CTs) on major circuits provides continuous current monitoring. This allows for the early detection of anomalies, such as gradual increases in current draw that might indicate a developing fault. Similarly, temperature sensors placed on equipment like motors or transformers can identify overheating issues before they lead to catastrophic failures. Consider a case study of a large manufacturing facility using hundreds of such sensors; real-time monitoring revealed an impending motor failure, preventing costly downtime. Another example involves a data center where smart sensors detected unusual power fluctuations, pinpointing a failing power supply unit before it triggered a system outage. The deployment of smart sensors is crucial for effective data collection and proactive problem resolution.
Data from these sensors can be aggregated and analyzed using specialized software, allowing for pattern recognition and predictive modeling. By analyzing historical data, it’s possible to identify trends and anticipate potential failures. For example, a consistent increase in motor current draw, coupled with rising temperature readings, could indicate impending bearing failure. Another example involves a network of smart meters monitoring energy usage in an apartment complex. Anomalies in energy consumption in a particular unit can signal an electrical fault or faulty appliance. Smart sensors are not just about detection, but also provide valuable insights for preventative maintenance, optimizing energy efficiency and extending the lifespan of critical equipment. Statistical analysis of the gathered data can further reveal hidden correlations and provide early warnings. Data acquisition and sensor technology is evolving rapidly, with advancements in sensor miniaturization, wireless communication, and data analytics paving the way for even more sophisticated electrical monitoring and troubleshooting systems.
Advanced Analytics and Predictive Modeling
Once the data is collected, advanced analytical techniques can be applied to extract valuable insights. Machine learning algorithms can identify patterns and anomalies that might be missed by human observation. For example, a machine learning model can be trained to detect subtle changes in voltage waveforms indicative of a failing capacitor. This predictive capability allows for proactive maintenance, preventing unexpected downtime. Consider a large-scale power grid where machine learning models can predict the likelihood of power outages based on weather patterns and historical data. This helps utility companies allocate resources effectively and mitigate the impact of potential disruptions. Another example involves industrial robots in a manufacturing plant. Sensors monitor motor performance and other vital parameters, and machine learning algorithms can predict potential failures with high accuracy, preventing costly production delays. The ability to predict failures allows for scheduled maintenance, minimizing disruption and maximizing equipment lifespan. In addition to predictive modeling, statistical process control (SPC) methods can be employed to monitor the stability of electrical systems and detect deviations from normal operating parameters. SPC charts visually represent the data and help identify trends, making it easier to spot anomalies and take corrective action. Combining these analytical techniques provides a powerful approach to data-driven electrical troubleshooting, moving beyond simple diagnostic methods and enabling proactive problem resolution. The combination of sensor data and advanced analytics empowers electricians to work smarter, not harder.
Predictive models can be deployed to various systems, such as industrial control systems (ICS), providing proactive monitoring and alert systems. This prevents cascading failures and system-wide shutdowns. For example, a manufacturing plant uses a predictive model to monitor the health of its programmable logic controllers (PLCs). Any deviation from normal operation triggers an alert, enabling immediate intervention and preventing costly production downtime. Moreover, the continuous data monitoring allows for improvements in system design and maintenance procedures. For example, repeated failures in a specific component might indicate design flaws or issues with the installation process. By analyzing these failure patterns, it is possible to enhance system reliability and prevent future occurrences. By combining advanced analytics with historical data and real-time sensor readings, predictive maintenance strategies can significantly reduce downtime and improve system reliability across a wide range of applications.
Case Studies and Real-World Applications
Numerous real-world case studies demonstrate the effectiveness of data-driven electrical troubleshooting. One example is a large data center that implemented a comprehensive sensor network to monitor power consumption and equipment performance. By using machine learning algorithms to analyze the data, they were able to predict and prevent several critical failures, avoiding significant downtime and financial losses. This proactive approach resulted in a substantial reduction in maintenance costs and improved overall system reliability. Another compelling case involves a smart city initiative that implemented smart meters and sensor networks to monitor the entire city's electrical grid. The collected data enabled efficient energy management and allowed for real-time detection of anomalies and potential faults. This proactive approach reduced power outages and improved the overall quality of service. These examples highlight the significant benefits of adopting a data-driven approach to electrical troubleshooting. In the first case study, the data-driven approach allowed the data center to avoid a major outage that could have cost millions of dollars. In the second case study, the data collected from the smart grid helped the city to reduce energy costs and improve the reliability of its power system. These are just two examples of the many ways that data-driven electrical troubleshooting can be used to improve efficiency and reliability.
Consider a manufacturing plant experiencing frequent motor failures. By installing sensors on the motors to monitor their temperature, vibration, and current draw, it's possible to identify subtle indicators of impending failure. Machine learning models can analyze this data and predict when maintenance is necessary, reducing downtime and extending the lifespan of the motors. In another instance, a building management system (BMS) could use sensor data to optimize energy consumption, reducing energy bills and environmental impact. The BMS can learn patterns in energy usage and adjust accordingly, minimizing waste and maximizing energy efficiency. These scenarios demonstrate the adaptability of data-driven methodologies across various applications, showcasing their potential for improving efficiency and minimizing unexpected disruptions. The implementation of data-driven strategies empowers professionals with proactive solutions, significantly reducing reactive maintenance and leading to long-term cost savings. Data visualization tools facilitate clear interpretation of collected data, making it easier to identify potential issues. This improves the efficiency and effectiveness of troubleshooting.
Future Trends and Implications
The field of data-driven electrical troubleshooting is rapidly evolving. Advancements in sensor technology, machine learning algorithms, and data analytics are constantly improving the accuracy and efficiency of these methods. We can expect to see even more sophisticated sensors that can monitor a wider range of parameters, providing a more comprehensive picture of the electrical system's health. Machine learning algorithms will become even more powerful, capable of identifying even subtle anomalies and predicting failures with greater accuracy. This will lead to more proactive maintenance, further reducing downtime and improving system reliability. The integration of these technologies with existing building management systems (BMS) and industrial control systems (ICS) will streamline data collection and analysis, making it easier to manage and maintain complex electrical systems. Improved data visualization tools will also make it easier to understand and interpret the data, making it accessible to a wider range of users. The growing adoption of the Internet of Things (IoT) will further expand the possibilities for data collection and analysis. This will allow for a more interconnected and integrated approach to electrical troubleshooting, leading to a more efficient and reliable electrical infrastructure. The development of edge computing and cloud-based analytics will enable real-time data processing and analysis, providing immediate insights and improving decision-making.
The increasing reliance on renewable energy sources, such as solar and wind power, presents new challenges and opportunities for data-driven troubleshooting. The intermittent nature of these sources requires sophisticated monitoring and control systems to ensure grid stability. Data-driven methods will play a crucial role in managing the integration of renewable energy sources and ensuring the reliability of the power grid. Furthermore, the increasing complexity of electrical systems, particularly in smart cities and industrial automation, necessitates more advanced troubleshooting techniques. Data-driven methods provide the tools needed to manage and maintain these systems effectively. The combination of advanced sensors, machine learning, and cloud-based analytics will enable proactive maintenance and predictive modeling, minimizing disruptions and maximizing efficiency. As technology continues to evolve, expect data-driven electrical troubleshooting to become even more sophisticated and widely adopted, leading to a more resilient and efficient electrical infrastructure.
Conclusion
Data-driven electrical troubleshooting offers a significant advancement over traditional methods. By leveraging sensor technology, advanced analytics, and predictive modeling, we can move from reactive to proactive maintenance, significantly reducing downtime and improving overall system reliability. The numerous case studies and real-world applications demonstrate the effectiveness of this approach across various industries and applications. As technology continues to evolve, we can expect even more sophisticated tools and techniques to emerge, further enhancing the accuracy and efficiency of data-driven electrical troubleshooting. This approach isn't just about fixing problems; it's about preventing them, leading to significant cost savings and improved operational efficiency. The integration of these technologies is crucial for maintaining the reliability and efficiency of electrical infrastructure in an increasingly complex and interconnected world. The future of electrical troubleshooting is data-driven, enabling proactive problem-solving and greater operational efficiency.