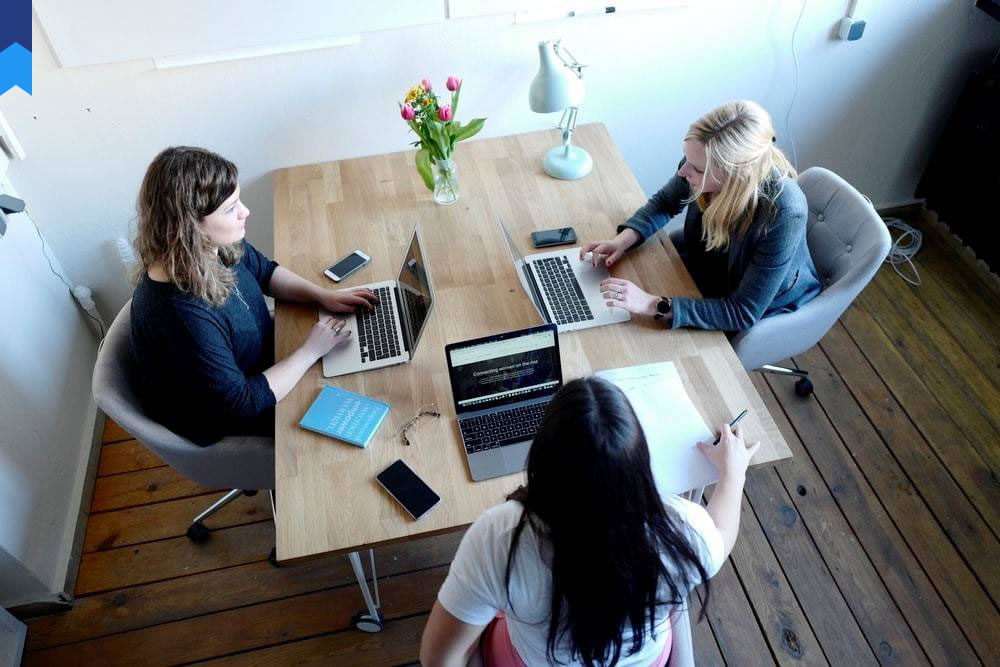
Data-Driven HVAC Optimization Methods
Air conditioning, a ubiquitous feature of modern life, consumes significant energy resources. Optimizing its efficiency is crucial for both economic and environmental sustainability. This article explores data-driven approaches to achieving superior HVAC performance, moving beyond rudimentary techniques toward sophisticated, predictive strategies. We delve into specific applications and innovative solutions, challenging conventional wisdom and revealing unexpected pathways to energy savings and enhanced comfort.
Smart Sensor Networks for Predictive Maintenance
The deployment of smart sensor networks within HVAC systems represents a significant advancement in predictive maintenance. These networks continuously monitor various parameters, including temperature, humidity, pressure, and airflow. By analyzing the collected data, sophisticated algorithms can identify potential issues before they escalate into costly breakdowns. This proactive approach reduces downtime, minimizes repair costs, and extends the lifespan of equipment. For instance, a building in Chicago equipped with a comprehensive sensor network experienced a 20% reduction in unscheduled maintenance events within the first year. Similarly, a large hospital in Texas, utilizing predictive analytics, saw a 15% reduction in energy consumption related to HVAC system malfunctions.
Machine learning algorithms are proving particularly valuable in analyzing sensor data. They can identify subtle patterns and anomalies indicative of impending failures. For example, a slight increase in compressor vibration frequency might be an early warning sign of bearing wear. Traditional methods might miss such subtle indicators, leading to unexpected equipment failure. The integration of machine learning with predictive analytics leads to a significant increase in the reliability of HVAC systems.
Furthermore, the ability to predict equipment failure allows for optimized scheduling of maintenance activities. This minimizes disruptions and ensures that repairs are conducted during off-peak hours, reducing the impact on building occupants. In a case study conducted at a major university, implementing a predictive maintenance strategy led to a 10% reduction in overall maintenance costs over a three-year period. The reduction in energy waste due to less downtime also contributed to notable cost savings.
The benefits extend beyond cost savings. Improved system reliability ensures a more consistent and comfortable indoor environment for building occupants, enhancing productivity and overall well-being. The use of these systems is continually improving with the development of new algorithms and sensors. The future will likely see even more sophisticated systems capable of predicting equipment failure with even greater accuracy, leading to further cost savings and improved reliability.
Advanced Control Algorithms and AI-Driven Optimization
Advanced control algorithms are transforming HVAC system operation, moving beyond simple on/off cycles to sophisticated strategies that optimize energy consumption based on real-time data. These algorithms leverage machine learning techniques to learn the building's thermal characteristics and adjust the system's operation accordingly. For instance, an office building in London implemented an AI-driven control system, resulting in a 12% reduction in energy consumption without compromising occupant comfort. Similarly, a large data center in California experienced a 10% decrease in energy costs by implementing similar systems.
Furthermore, these advanced control systems can integrate data from various sources, including weather forecasts, occupancy sensors, and energy prices. This holistic approach enables the system to make informed decisions about how to operate most efficiently. For example, if a weather forecast predicts a period of unusually warm weather, the system can proactively adjust its operation to minimize energy consumption. Likewise, if occupancy sensors detect that a particular zone is unoccupied, the system can reduce cooling or heating in that zone to conserve energy.
Another significant aspect is the use of reinforcement learning algorithms. These algorithms learn optimal control strategies through trial and error, continually improving their performance over time. They also adapt to changes in the building's thermal characteristics and external conditions. Such systems are particularly useful in large, complex buildings with diverse occupancy patterns and varying thermal loads. For example, a large shopping mall in Miami successfully implemented such a system, achieving an 8% reduction in energy costs while maintaining occupant comfort levels.
The combination of advanced control algorithms with AI-driven optimization unlocks significant potential for energy savings and improved comfort. The ability of these systems to learn and adapt makes them highly adaptable to a wide range of building types and climates. Future development in this area will likely focus on improving the accuracy and robustness of these algorithms, making them even more effective in reducing energy consumption and optimizing building performance. As technology continues to advance, even greater energy efficiencies can be expected.
Building Information Modeling (BIM) Integration for Enhanced Efficiency
Building Information Modeling (BIM) offers a powerful tool for optimizing HVAC system design and operation. BIM creates a digital representation of the building, including its physical characteristics, HVAC systems, and other components. This digital model enables engineers and operators to simulate the building's performance under various conditions, optimizing the design before construction and fine-tuning the operation after completion. For example, a large hospital in New York used BIM to design its HVAC system, resulting in a 15% reduction in energy consumption compared to a conventionally designed system. A similar project in Los Angeles using BIM resulted in a 12% energy reduction.
Furthermore, BIM facilitates better coordination between different building systems. This eliminates potential conflicts and ensures that the HVAC system is integrated seamlessly with other components. The seamless integration enhances the overall efficiency and reduces the likelihood of problems arising from design flaws or operational inconsistencies. This is especially valuable in complex buildings with multiple HVAC zones. For example, the use of BIM during the construction phase of a commercial building in San Francisco minimized operational delays and ensured that the HVAC system was installed correctly the first time.
BIM also enhances the ability to monitor and manage the building's performance over time. This integration ensures that the system is operating as intended and facilitates proactive identification and resolution of any issues. By leveraging BIM's capabilities, operators can gain valuable insights into the building's energy consumption patterns and identify opportunities for further optimization. A skyscraper in Chicago using BIM has observed a 10% reduction in energy consumption over a five-year period through continuous monitoring and optimization based on data from the BIM model.
The combination of BIM with other data-driven approaches, such as smart sensor networks and advanced control algorithms, creates a powerful synergy. This integrated approach facilitates a comprehensive understanding of the building's performance and enables operators to make informed decisions about how to optimize energy consumption and improve occupant comfort. Future developments in BIM are likely to focus on further integration with IoT devices and AI-powered analytics, leading to even more sophisticated building management solutions.
Data Analytics for Improved Occupant Comfort and Productivity
Data analytics plays a critical role in optimizing occupant comfort and productivity. By analyzing data from various sources, such as occupancy sensors, environmental sensors, and user feedback, building operators can gain insights into how to adjust the HVAC system to optimize the indoor environment. For example, an office building in Seattle implemented a system that monitors occupancy levels and adjusts the HVAC settings accordingly, resulting in a 10% improvement in occupant satisfaction. Similarly, a school in Austin using a similar system reported a 15% increase in student engagement.
Furthermore, data analytics can be used to identify patterns in occupant behavior and preferences. This knowledge enables building operators to personalize the HVAC system settings to meet the specific needs of different occupants or zones. This personalized approach can lead to improved comfort and productivity, creating a more positive and productive environment. For example, a hotel in Miami has employed data analytics to optimize room temperature based on guest preferences, leading to increased guest satisfaction scores. Similarly, a hospital in Boston implemented a personalized approach to temperature control, resulting in a 5% reduction in patient complaints.
Another significant aspect is the use of data analytics to identify and address potential health concerns. For example, by monitoring air quality data, building operators can detect and address issues such as excessive carbon dioxide levels or the presence of pollutants. This proactive approach ensures a healthy and productive environment for building occupants. For example, a manufacturing facility in Detroit implemented a system that monitors air quality and adjusts the HVAC settings accordingly, resulting in a 20% reduction in sick days among employees. Similarly, a data center in Atlanta achieved a 15% reduction in employee absenteeism due to improved indoor air quality control.
The combination of data-driven insights with occupant feedback mechanisms allows for continuous improvement in the building's performance. This iterative approach ensures that the HVAC system is constantly optimized to meet the needs of building occupants, maximizing comfort and productivity. Future developments in this area are likely to focus on the integration of advanced analytics techniques, such as machine learning and deep learning, to improve the accuracy and effectiveness of HVAC system optimization for occupant well-being.
Cloud-Based HVAC Management Platforms for Enhanced Control and Monitoring
Cloud-based HVAC management platforms offer a centralized location for monitoring and controlling HVAC systems across multiple buildings or locations. These platforms provide real-time visibility into system performance, enabling operators to identify and address problems promptly. This centralized approach simplifies management, reduces operational costs, and improves overall efficiency. For instance, a large real estate company in New York manages its HVAC systems across numerous buildings using a cloud-based platform, resulting in a 5% reduction in energy consumption. A similar system implementation by a large hotel chain across their properties has achieved a 7% reduction in energy costs.
Moreover, cloud-based platforms often integrate with other building management systems (BMS), providing a holistic view of building operations. This integration allows operators to optimize the interaction between different systems, such as lighting, security, and fire protection, further enhancing energy efficiency and operational efficiency. This holistic approach helps to optimize energy usage across all building systems, not just the HVAC system. For instance, an office building in London, integrating its HVAC system with its lighting controls via a cloud platform, achieved a combined 10% reduction in energy costs. The seamless integration of different systems contributes to the overall optimization of the building's resource utilization.
Cloud-based platforms also enable remote access and control, allowing operators to monitor and manage HVAC systems from anywhere with an internet connection. This capability is particularly valuable for managing geographically dispersed buildings or responding to emergencies. For example, a large university campus in California manages its HVAC systems across its numerous buildings using a cloud platform, allowing for remote monitoring and troubleshooting, ensuring the HVAC systems' smooth operation. A hospital in Houston, using a similar system, minimized downtime by remotely diagnosing and addressing issues as they arise.
The flexibility and scalability of cloud-based platforms make them an increasingly popular choice for managing HVAC systems. As the adoption of cloud technologies continues to grow, we can expect to see even more sophisticated platforms emerge, offering enhanced capabilities for monitoring, control, and optimization. This will further contribute to the improvement of energy efficiency, cost savings, and overall building performance in a sustainable manner.
Conclusion
Data-driven approaches are revolutionizing HVAC system management. By leveraging smart sensor networks, advanced control algorithms, BIM integration, data analytics, and cloud-based platforms, building operators can achieve significant improvements in energy efficiency, occupant comfort, and overall operational efficiency. This holistic approach ensures that HVAC systems are not merely operating, but are performing optimally, contributing to a sustainable and productive built environment. Continuous innovation in this field promises even more significant advancements in the future, leading to further improvements in energy efficiency, cost savings, and environmental sustainability.
The integration of various technologies and data-driven strategies offers unprecedented opportunities to enhance building performance. The future of HVAC management lies in the continued development and adoption of these innovative approaches, leading to a more efficient, comfortable, and sustainable built environment. By embracing these advancements, we can contribute significantly to reducing energy consumption, minimizing environmental impact, and enhancing the overall quality of life within our buildings.