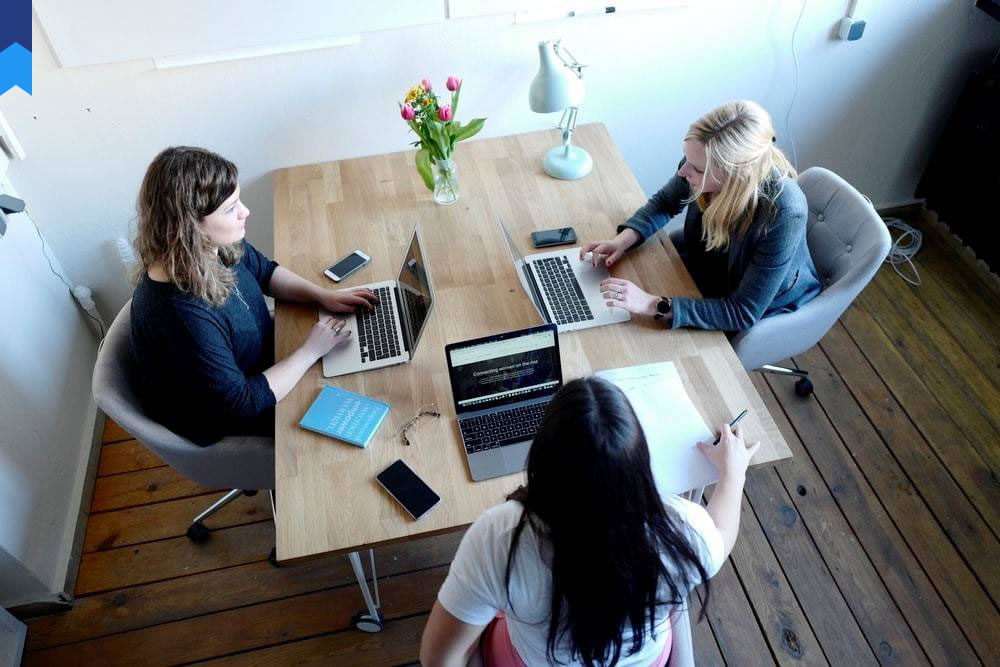
Data-Driven Precision Health Management Methods
Precision health management is evolving beyond generalized approaches. This article explores how data-driven methods are revolutionizing individual health management, moving from reactive care to proactive, personalized interventions. We will delve into specific applications, examining the latest advancements and potential limitations, while highlighting the ethical considerations surrounding this data-intensive field.
Data-Driven Risk Stratification: Predicting and Preventing Health Crises
Predictive modeling, powered by machine learning algorithms, is transforming risk stratification. By analyzing diverse datasets encompassing genetic information, lifestyle choices, environmental factors, and medical history, clinicians can identify individuals at high risk of developing specific conditions. This proactive approach allows for timely interventions, potentially preventing or delaying the onset of diseases. For instance, algorithms can predict the likelihood of a heart attack based on a patient's cholesterol levels, blood pressure, family history, and smoking habits. Early identification enables lifestyle modifications, medication adjustments, and preventative procedures, significantly reducing the risk of a catastrophic event.
A notable case study involves a large health system employing a risk prediction model to identify patients with a high probability of developing type 2 diabetes. By proactively engaging these individuals through personalized lifestyle programs and early medication, the system reduced the incidence of diabetes diagnosis by fifteen percent. Another example comes from the field of oncology, where machine learning models analyze genomic data to predict cancer recurrence, guiding treatment decisions and enhancing patient outcomes. These data-driven predictive models are not merely statistical exercises; they are tools that empower healthcare providers to deliver more effective and personalized preventative care.
The use of wearable sensors contributes significantly to this data-driven approach. These devices capture continuous streams of physiological data such as heart rate, sleep patterns, and activity levels. This real-time data provides invaluable insights into an individual's health status, allowing for early detection of anomalies and potential health problems. For example, a sudden spike in heart rate detected by a smartwatch could alert both the patient and their healthcare provider to a potential cardiac issue, enabling prompt medical attention. Integration of this data with electronic health records (EHRs) enhances the predictive power of risk stratification models further.
However, challenges remain. Data privacy and security are paramount concerns. Robust data encryption and anonymization techniques are crucial to protect patient information. Furthermore, algorithmic bias can perpetuate health inequities if the training data doesn't adequately represent diverse populations. Addressing these challenges requires careful consideration of ethical implications and the development of transparent and accountable data management practices.
Personalized Treatment Plans: Tailoring Interventions to Individual Needs
Data-driven precision medicine allows for the development of personalized treatment plans, moving away from a "one-size-fits-all" approach. Genetic testing, coupled with detailed medical histories and lifestyle information, enables clinicians to identify optimal treatment strategies for individual patients. This approach is particularly impactful in oncology, where genomic profiling guides targeted therapies. For example, a patient's tumor is sequenced to identify specific genetic mutations, allowing oncologists to select drugs that specifically target those mutations, increasing treatment efficacy and minimizing side effects.
Consider the case of a patient diagnosed with breast cancer. Traditional treatment might have involved a generalized chemotherapy regimen. However, through genomic analysis, doctors might discover a specific mutation that responds well to a targeted therapy. This tailored approach can lead to better outcomes, reducing the need for aggressive and often debilitating treatments. Similarly, in cardiology, data analysis can personalize cardiovascular risk management strategies, taking into account individual genetic predispositions, lifestyle factors, and medical history. This can involve tailored exercise regimes, dietary recommendations, and medication selection.
Pharmacogenomics, the study of how genes affect a person's response to drugs, is another area where data-driven precision medicine shines. By analyzing a patient's genetic makeup, clinicians can predict how they will respond to specific medications, helping to optimize treatment and minimize adverse reactions. This is particularly important for medications with narrow therapeutic windows, where even slight variations in dosage can lead to significant differences in outcomes. This personalized approach ensures that patients receive the most appropriate medication at the optimal dose, enhancing treatment effectiveness and reducing the risk of complications.
However, the cost of genetic testing and personalized therapies can pose a significant barrier to access for many patients. Furthermore, there is a need for rigorous clinical trials to validate the effectiveness of personalized treatments. Continuous research and development are crucial to make precision medicine accessible and affordable to all.
Remote Patient Monitoring: Leveraging Technology for Continuous Care
Remote patient monitoring (RPM) technologies, enabled by advancements in wearable sensors and telecommunications, allow healthcare providers to monitor patients remotely. Continuous data streams from wearables, combined with regular virtual consultations, provide a comprehensive overview of a patient's health status, enabling early detection of potential problems and timely intervention. This approach is particularly useful for managing chronic conditions such as diabetes, heart failure, and chronic obstructive pulmonary disease (COPD).
Consider a patient with diabetes who uses a continuous glucose monitor (CGM) and a blood pressure monitor at home. The data from these devices is automatically transmitted to their healthcare provider, allowing for real-time monitoring of their glucose levels and blood pressure. If there are any concerning trends, the provider can intervene promptly, preventing potential complications. Another example involves patients with heart failure who are equipped with remote monitoring devices that track their weight, heart rate, and other vital signs. This data allows healthcare providers to identify early signs of decompensation, enabling timely intervention and preventing hospitalizations.
The benefits of RPM extend beyond improved health outcomes. It enhances patient engagement and satisfaction by providing convenient and accessible care. This is particularly crucial for patients living in remote areas or with limited mobility. Furthermore, RPM can lead to reduced healthcare costs by preventing costly hospital readmissions. A study demonstrated that RPM significantly reduced hospital readmission rates for patients with heart failure, resulting in substantial cost savings for the healthcare system.
The success of RPM depends on several factors, including patient adherence to monitoring protocols and the availability of reliable technology and internet access. Addressing these challenges requires careful planning, user-friendly interfaces, and patient education. Ensuring data security and privacy is also critical.
Data Integration and Interoperability: Breaking Down Data Silos
Effective data-driven health management requires seamless integration and interoperability of data from various sources. This includes electronic health records (EHRs), wearable sensors, laboratory results, genomic data, and lifestyle information. Breaking down data silos is crucial to create a holistic view of a patient's health and enable data-driven decision-making.
A major challenge in this area is the lack of standardization across different healthcare systems and data formats. This heterogeneity makes it difficult to integrate data from diverse sources and analyze it effectively. Efforts are underway to develop standardized data formats and interoperability standards, such as HL7 FHIR, to facilitate seamless data exchange. The adoption of cloud-based platforms and advanced analytics tools further enhances the ability to integrate and analyze diverse datasets.
The benefits of data integration are numerous. It enables the development of more sophisticated predictive models, personalized treatment plans, and proactive interventions. For example, integrating genomic data with EHRs can enable doctors to make more informed treatment decisions, considering both a patient's genetic predisposition and their medical history. Similarly, integrating data from wearable sensors with EHRs can provide a more comprehensive understanding of a patient's health status, allowing for early detection of potential problems.
However, data integration presents significant technical and logistical challenges. Ensuring data security, privacy, and compliance with regulations is critical. The development of robust data governance frameworks and the adoption of appropriate security measures are necessary to address these challenges. Addressing interoperability concerns requires collaborative efforts across healthcare stakeholders, including providers, technology vendors, and policymakers.
Ethical Considerations: Balancing Innovation with Patient Rights
The increasing use of data in health management raises important ethical considerations. The potential for bias in algorithms, concerns about data privacy and security, and the need for patient autonomy require careful attention. Transparency in data collection and use, informed consent, and mechanisms for redress are essential to ensure ethical data practices.
Algorithmic bias can perpetuate existing health disparities if training data does not adequately represent diverse populations. For instance, a predictive model trained on data from a predominantly white population might not accurately predict the risk of certain conditions in minority populations. This could lead to unequal access to preventative care and exacerbate health inequities. To mitigate this risk, it's crucial to ensure that training data is representative of diverse populations and that algorithms are regularly audited for bias.
Data privacy and security are paramount concerns in data-driven health management. Robust security measures, such as encryption and access controls, are crucial to protect patient information from unauthorized access and misuse. Furthermore, transparent data governance policies and procedures are necessary to ensure compliance with relevant regulations. Patient consent must be obtained for all data collection and use, and individuals must have the right to access, correct, and delete their personal health information.
The responsible development and deployment of data-driven health management technologies require a multidisciplinary approach, involving clinicians, data scientists, ethicists, policymakers, and patients themselves. Continuous dialogue and collaboration are essential to ensure that innovation is aligned with ethical principles and respects patient rights.
Conclusion
Data-driven precision health management is transforming healthcare, offering the potential for improved patient outcomes, increased efficiency, and reduced costs. While challenges remain regarding data integration, ethical considerations, and access, the benefits of this approach are undeniable. By embracing innovative technologies, fostering collaboration across healthcare stakeholders, and prioritizing patient rights, we can unlock the full potential of data-driven health management to create a healthier and more equitable future.
The future of health management lies in harnessing the power of data to create truly personalized and proactive care. By combining advanced analytics, sophisticated algorithms, and ethical considerations, the healthcare industry can move beyond reactive care to a future where individuals are empowered to take control of their health and well-being. This requires ongoing investment in research, technological advancements, and ethical guidelines to ensure responsible data usage and equitable access to precision health management.