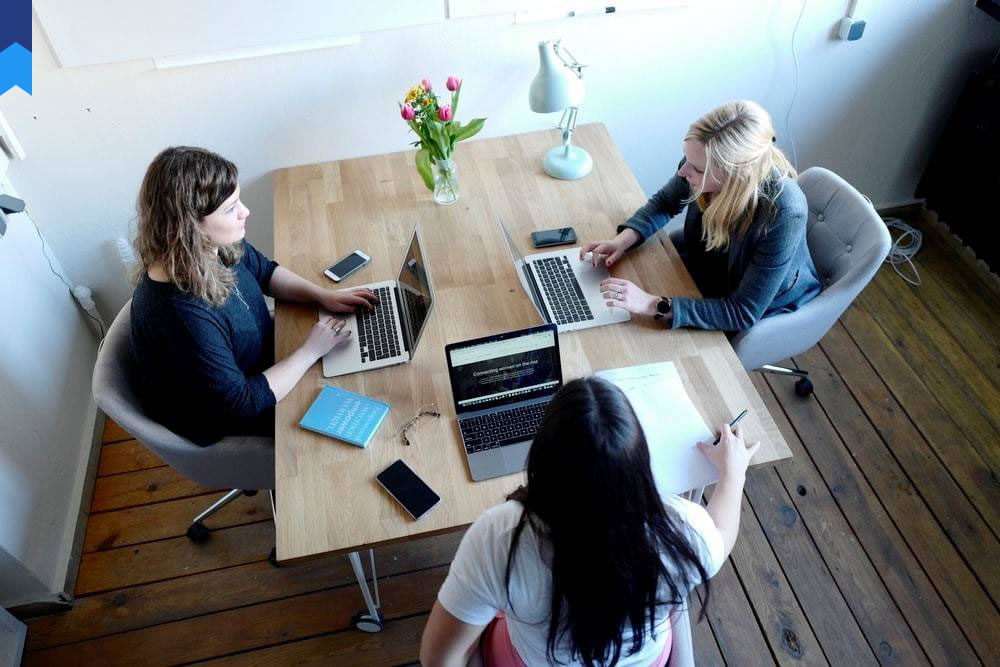
Data-Driven Tech Disruption Methods
The digital landscape is in constant flux, a maelstrom of innovation and disruption. Understanding the underlying currents driving this change requires more than gut instinct; it demands a data-driven approach. This article explores how data analysis is reshaping the tech industry, revealing not only trends but also the unforeseen consequences and opportunities they present. We'll delve into specific cases, examine emerging methodologies, and offer insights into navigating this ever-evolving environment.
Data-Driven Innovation in AI
Artificial intelligence is no longer a futuristic concept; it's rapidly transforming various sectors. Data is the lifeblood of AI, fueling its algorithms and shaping its capabilities. Analyzing vast datasets allows developers to identify patterns, predict user behavior, and personalize experiences. For example, Netflix's recommendation engine leverages data to suggest movies and shows tailored to individual preferences, increasing user engagement and subscription retention. Similarly, Amazon utilizes data analysis to optimize its supply chain, forecasting demand and minimizing inventory waste. The effectiveness of AI hinges on the quality and quantity of data it's trained on. Poor data quality can lead to biased algorithms and inaccurate predictions. Consequently, robust data governance and rigorous cleaning processes are crucial.
A striking case study is the use of AI in healthcare. Companies like IBM Watson are using AI to analyze medical images, detect diseases earlier, and personalize treatment plans. However, ethical considerations regarding data privacy and algorithmic bias remain paramount. The development and deployment of AI systems require careful consideration of these ethical implications. Another example is the use of AI in fraud detection, with companies utilizing machine learning algorithms to identify and prevent fraudulent transactions. The accuracy of these algorithms depends heavily on the quality and diversity of the training data. Failure to consider these factors can lead to false positives and negatively impact the user experience.
Furthermore, AI is increasingly used in autonomous vehicles. Self-driving cars rely on massive datasets to train their perception systems, enabling them to navigate complex environments. The challenge here lies in handling edge cases and ensuring the safety and reliability of these systems. Data analysis plays a critical role in improving the performance and safety of autonomous vehicles. The data collected from real-world driving is used to train and refine the algorithms, leading to continuous improvement in the system's capabilities.
Data-driven approaches in AI development also involve continuous monitoring and evaluation. Regularly analyzing the performance of AI systems allows developers to identify areas for improvement and optimize their algorithms. This iterative process ensures that AI systems remain accurate, reliable, and effective over time. The ability to adapt and evolve is essential in the rapidly changing technological landscape.
Data-Driven Cybersecurity Strategies
The digital realm is increasingly vulnerable to cyber threats. Data breaches are becoming more frequent and sophisticated, resulting in significant financial losses and reputational damage. Effective cybersecurity strategies require a proactive and data-driven approach. Analyzing network traffic, user behavior, and system logs can help identify anomalies and potential security vulnerabilities. Machine learning algorithms can be employed to detect and prevent malicious activities in real-time. The use of security information and event management (SIEM) systems is crucial for collecting and analyzing security data from various sources.
One compelling case study is the use of data analytics to detect insider threats. Analyzing employee access patterns and system usage can reveal suspicious activity that might indicate malicious intent. Another example involves the use of threat intelligence platforms to gather and analyze information about emerging threats. This information can be used to proactively protect systems and networks from known vulnerabilities. These platforms provide insights into the latest attack vectors and help security teams prioritize their efforts. Organizations can effectively mitigate risks and enhance their overall security posture by leveraging data-driven insights.
Furthermore, data analysis can be used to assess the effectiveness of existing security measures. By analyzing security incident reports, organizations can identify weaknesses in their security infrastructure and improve their response capabilities. This iterative process allows organizations to continuously strengthen their defenses against cyber threats. The use of penetration testing and vulnerability scanning is essential for identifying potential weaknesses in systems and applications. Regularly evaluating the security posture is critical in this ever-evolving landscape. Data-driven cybersecurity strategies require a proactive and adaptive approach to keep pace with the ever-evolving threat landscape.
The increasing sophistication of cyberattacks requires organizations to adopt a more dynamic and data-driven approach to security. Real-time threat detection and response capabilities are crucial for mitigating the impact of attacks. Integrating security analytics into existing workflows allows organizations to improve their overall security posture and reduce their vulnerability to cyber threats.
Data-Driven Market Analysis and Prediction
In the dynamic world of technology, understanding market trends is crucial for success. Data analysis provides valuable insights into customer preferences, competitor activities, and emerging market opportunities. By analyzing sales data, social media trends, and customer reviews, businesses can gain a deeper understanding of their target audience. This information can be used to refine their products, improve their marketing strategies, and make informed business decisions. Analyzing website traffic and user engagement metrics helps businesses understand user behavior and optimize their online presence. Market analysis techniques are evolving rapidly, becoming more sophisticated and powerful, using machine learning to forecast future trends.
A prime example is the use of A/B testing to optimize marketing campaigns. By testing different versions of advertisements or website designs, businesses can identify which approaches are most effective in converting leads into customers. Another powerful tool for market analysis is sentiment analysis. Analyzing customer reviews and social media posts to understand the overall sentiment toward a product or brand can help businesses identify areas for improvement and address potential issues. This allows companies to proactively respond to negative feedback and prevent potential crises.
Furthermore, predictive analytics can help businesses forecast future demand and optimize their inventory management. Analyzing historical sales data and market trends can help businesses anticipate future demand and avoid stockouts or overstocking. This capability is essential for efficient operations and profitability in any industry, allowing for better resource allocation. Predictive analytics also helps businesses identify potential risks and opportunities, allowing for proactive planning and mitigation strategies. Data-driven market analysis techniques are becoming increasingly important for businesses to stay competitive in this rapidly evolving market. Leveraging this data allows for smarter, faster decisions that improve operational efficiency and drive growth.
The increasing availability of data and advanced analytics tools empowers businesses to make more informed decisions. This ability to predict future trends and anticipate customer needs is critical for success in today's competitive market. Continuous monitoring and evaluation of market trends are essential for adapting to changes in the market landscape. The ability to rapidly respond to evolving customer needs and market demands is a key driver of success in this field.
Data-Driven Product Development
The development of successful tech products is no longer a matter of intuition alone. Data analysis is increasingly playing a crucial role in guiding the entire product lifecycle, from initial concept to post-launch optimization. By analyzing user feedback, market research, and competitor analysis, product teams can gain a better understanding of user needs and preferences. This information can be used to develop products that better meet customer expectations and generate higher user engagement. Data-driven approaches ensure that product development is focused on creating products that solve real user problems and provide value to the customer. Understanding user needs is the first step in developing successful products.
A classic case study is the iterative development approach used by companies like Apple. By collecting user feedback and analyzing usage patterns, Apple continually refines its products and improves the user experience. Another example is the use of A/B testing to compare different product designs or features. This enables product teams to identify what aspects of the product resonate the most with users. Companies are using this approach to refine their products and enhance user engagement. This allows for making data-driven decisions across every stage of the product development lifecycle.
Furthermore, data analysis can be used to track product performance after launch. Analyzing user reviews, app store ratings, and usage data provides valuable insights into how the product is performing in the real world. This data can be used to identify areas for improvement and optimize the product over time. Continuous monitoring and improvement of the product is critical for its success in the market. Product development involves ongoing improvement and adaptation to user feedback. A data-driven approach helps to make this process more efficient and effective.
The use of data analytics in product development is transforming the way products are conceived, developed, and launched. By leveraging the power of data, companies can create products that are more aligned with user needs and more likely to succeed in the market. This approach ensures that the products developed not only meet the requirements but also exceed user expectations.
Data-Driven Resource Allocation
Efficient allocation of resources is crucial for the success of any tech company. Data analysis can provide valuable insights into where resources should be allocated to maximize their impact. By analyzing historical data and projecting future trends, companies can make informed decisions regarding staffing, budget allocation, and investment priorities. Data-driven approaches help to ensure that resources are allocated effectively, leading to improved efficiency and profitability. Analyzing operational data helps to identify areas where resources can be optimized and inefficiencies reduced. This systematic approach is crucial for effective resource management.
A practical example is the use of data analysis to optimize marketing spend. By analyzing campaign performance data, companies can identify which channels are most effective and allocate their budget accordingly. Another use case is the allocation of engineering resources. By tracking the progress of different projects and analyzing developer productivity, companies can optimize their engineering workforce and ensure efficient project completion. This data-driven approach ensures that resources are focused on the most critical and valuable tasks.
Furthermore, data analysis can help companies identify areas for cost reduction and efficiency improvements. By analyzing operational data and identifying areas of waste, companies can optimize their processes and reduce costs. This data-driven approach helps to improve profitability and sustainability. This systematic analysis of processes enables the identification of inefficiencies and optimization opportunities. Real-time data analytics dashboards can provide insights into operational performance and guide resource allocation decisions.
The use of data-driven approaches in resource allocation is becoming increasingly crucial for tech companies looking to maximize their efficiency and profitability. By leveraging the power of data, companies can make more informed decisions about where to allocate their resources and achieve their strategic goals. This approach is fundamental to achieving sustainable growth and operational excellence.
Conclusion
Data is the new gold in the tech industry. The ability to collect, analyze, and interpret data effectively is no longer a luxury; it's a necessity for survival and success. This article has explored how data-driven methods are revolutionizing various aspects of the tech world, from AI development to cybersecurity and product management. By embracing a data-driven approach, tech companies can unlock new opportunities, mitigate risks, and achieve sustainable growth. However, it's crucial to remember that data is only as good as the interpretation and the ethical considerations surrounding its use. A responsible and ethical approach to data analysis is vital to ensure fair and equitable outcomes.
Looking ahead, the increasing availability of data and the development of more sophisticated analytics tools will further accelerate this trend. Companies that fail to adopt a data-driven approach risk falling behind their competitors. Therefore, investing in data infrastructure, developing analytical capabilities, and fostering a data-driven culture is essential for companies seeking to thrive in this rapidly changing landscape. Continuous learning and adaptation are essential to keep pace with the evolving technological landscape.