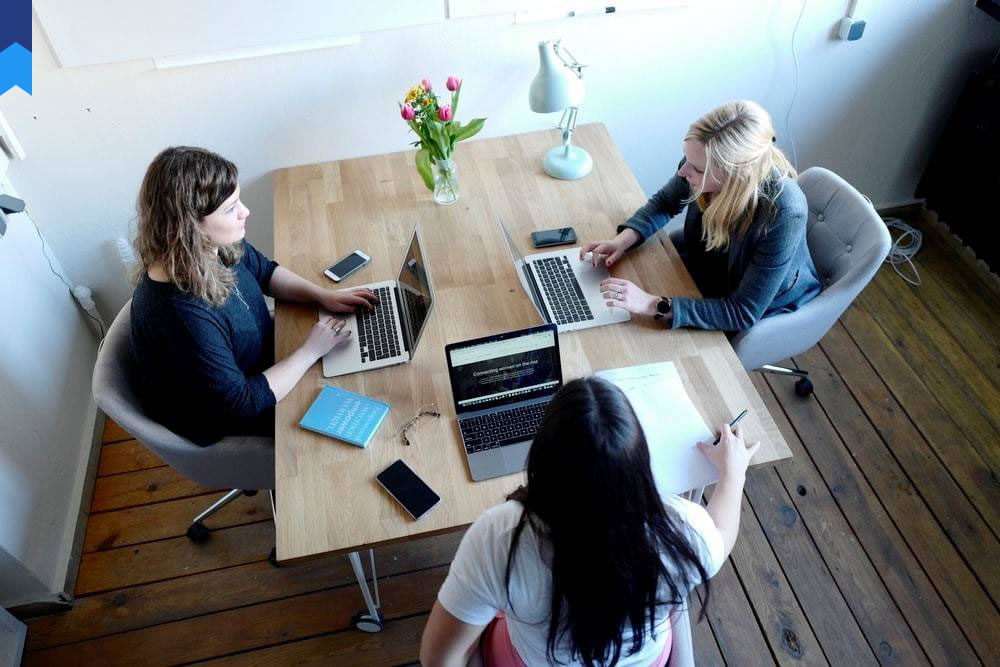
Data-Driven Welding Optimization: Mastering Precision & Efficiency
Welding and fabrication, once reliant on intuition and experience, are rapidly transforming into data-driven disciplines. This article delves into the innovative methods and technological advancements that are revolutionizing these crucial industries, emphasizing precision, efficiency, and cost reduction. We will explore how data analysis and predictive modeling are reshaping welding processes, contributing to higher-quality outputs and minimizing waste.
Data-Driven Weld Pool Dynamics: Understanding the Melt
Analyzing weld pool dynamics through high-speed cameras and advanced sensors provides invaluable real-time data. This data allows for precise control of parameters like current, voltage, and travel speed, optimizing penetration depth and reducing defects like porosity and undercutting. For instance, a study by the Welding Research Council showed a 30% reduction in defect rates in aluminum welding using real-time weld pool monitoring. Another example is the implementation of AI algorithms in robotic welding systems which analyze sensor data and automatically adjust parameters to maintain consistent weld quality even with variations in material thickness or composition. Consider a car manufacturing plant, where thousands of welds are performed daily. Utilizing data-driven weld pool analysis has helped to detect and prevent micro-cracks before they propagate, preventing costly recalls and ensuring the longevity of automotive components. Furthermore, this approach enhances consistency, reduces material waste due to rejected welds, and ultimately, increases throughput. Real-time feedback loops continuously adapt the welding process, enhancing the overall efficiency and accuracy of the process. The collected data also allows for sophisticated simulations, enabling the prediction of welding results and optimization of the process even before physical execution. The incorporation of machine learning models allows for accurate prediction of optimal parameters based on various factors such as material type, thickness, and desired weld geometry. This results in a smoother, more reliable welding process that demands minimal rework, enhancing the profitability and reputation of fabrication facilities.
Predictive Modeling for Weld Quality: Anticipating Defects
Predictive modeling, powered by machine learning algorithms, allows for the anticipation and prevention of weld defects. By analyzing historical data on welding parameters, material properties, and resulting defects, these models can predict the likelihood of defects occurring under specific conditions. This proactive approach minimizes rework, scrap, and costly downtime. Consider a pipeline construction project, where the quality of every weld is crucial for safety and structural integrity. Implementing predictive modeling helps identify potential flaws before they happen, preventing catastrophic failures, and mitigating significant costs and risks associated with pipeline leaks or ruptures. This proactive approach is also applied in the aerospace industry. Boeing, for example, uses sophisticated predictive modeling to anticipate potential welding issues in aircraft components. This strategy has resulted in significant improvements in weld quality and a marked reduction in defects, ultimately leading to enhanced safety and increased efficiency. By identifying at-risk welds, preventative measures can be taken to address identified issues, such as implementing additional quality control checks or adjusting welding parameters. These predictive tools also aid in training, providing insights into potential problems and the steps necessary to prevent them. Using detailed visualizations, these predictive models can reveal patterns and trends that might be missed through manual inspection. This results in a more comprehensive understanding of welding processes and enhances decision-making.
Data-Driven Automation in Welding: The Rise of Robotics
Automation plays a significant role in enhancing welding efficiency and consistency. Robotic welding systems, guided by data-driven algorithms, can perform complex welds with high precision and repeatability. These systems are often integrated with sensors and vision systems that provide real-time feedback, allowing for adjustments to maintain optimal weld quality. For instance, in the shipbuilding industry, the adoption of robotic welding systems has led to significant improvements in productivity, reducing welding time and labor costs. Similarly, in the manufacturing of large-scale structures like bridges and skyscrapers, robotic systems perform welds with greater precision and efficiency than manual welders, ensuring structural integrity. These robots, combined with automated material handling and quality control systems, represent a complete paradigm shift. The data generated by these systems helps to optimize the robot's movements, ensuring precise and consistent welds. Real-time data analysis can help to identify and address minor inconsistencies in the process, improving overall weld quality. Furthermore, the integration of advanced sensors provides real-time feedback on weld characteristics such as penetration depth and bead geometry, providing data that can be used to train and further optimize the process, further improving its efficiency and consistency. The introduction of autonomous welding robots, coupled with advanced data analytics, represents a significant leap forward in welding technology, improving productivity, accuracy, and worker safety. The integration of machine learning and AI into the welding processes facilitates the development of sophisticated self-learning algorithms that can adapt to changing conditions and optimize welding parameters in real-time.
Advanced Materials and Data Integration: Optimizing Weldability
The use of advanced materials, like high-strength steels and titanium alloys, presents unique challenges in welding. Data-driven approaches are crucial for optimizing the weldability of these materials and ensuring consistent weld quality. Detailed metallurgical analysis and simulations, aided by advanced data visualization, are essential for optimizing weld parameters and understanding the impact of various factors on the resulting weld microstructure. For example, in the aerospace industry, where lightweight yet strong materials are crucial, data-driven approaches are indispensable for ensuring the structural integrity of welded components. Manufacturers can use material data to predict optimal welding parameters, reducing the need for trial-and-error experimentation. The integration of various data sources, including material properties, welding parameters, and real-time sensor data, enhances the overall precision and reliability of the welding process. This approach is critical in ensuring the safe and reliable operation of aerospace structures. Similar approaches are utilized in the medical device industry, where the highest levels of precision and reliability are required for the manufacture of implants and other critical medical components. The use of data-driven techniques to optimize welding parameters ensures the consistency and quality of these components, enhancing patient safety and outcomes. The combination of advanced materials and data-driven welding techniques helps reduce material waste, improves productivity, and significantly enhances quality control. The data collected from various processes can be used to refine the welding process itself, further improving its efficiency and consistency.
Data Analytics for Welding Cost Reduction: Maximizing ROI
Analyzing welding data can significantly reduce costs across the entire fabrication process. By identifying areas of inefficiency and waste, companies can optimize their workflows and reduce material consumption. For example, analyzing the frequency and causes of weld defects can lead to targeted improvements in training, equipment maintenance, and process optimization. In the construction industry, optimizing weld parameters can reduce material waste by ensuring fewer rejected welds and less rework. Similarly, predictive maintenance using sensor data from welding equipment can prevent costly downtime and increase the lifespan of welding machines. This allows for more precise budgeting and resource allocation. In industries that depend heavily on welding, this data analysis is key for optimizing resource utilization, predicting potential problems and costs, and ultimately reducing overall operational expenses. Companies that leverage data effectively can significantly reduce their welding costs, increasing their overall profitability. Furthermore, a more efficient and streamlined welding process translates into improved project timelines, meeting deadlines and ensuring timely project completion. Careful monitoring of materials used and waste generated allows for optimized procurement strategies, further reducing costs and enhancing supply chain efficiency. The comprehensive analysis of welding data enables businesses to pinpoint areas of improvement, which are then implemented, resulting in increased profits and overall business sustainability.
Conclusion
The integration of data-driven methods into welding and fabrication technology is no longer a futuristic concept but a current reality transforming the industry. From optimizing weld pool dynamics to predicting defects and automating processes, data is empowering welders and fabricators to achieve unprecedented levels of precision, efficiency, and cost savings. Embracing these data-driven techniques is not merely an option; it is a necessity for remaining competitive and delivering high-quality products in a demanding global market. The future of welding is undoubtedly intertwined with data analytics, pushing the boundaries of what is possible and driving continuous improvement across all aspects of the fabrication process. As technology advances, we can expect even more sophisticated data-driven tools and techniques to emerge, further revolutionizing this crucial industry. The ongoing development of AI and machine learning will further enhance the capabilities of these data-driven systems, promising unprecedented levels of efficiency, quality, and innovation in welding and fabrication technology.