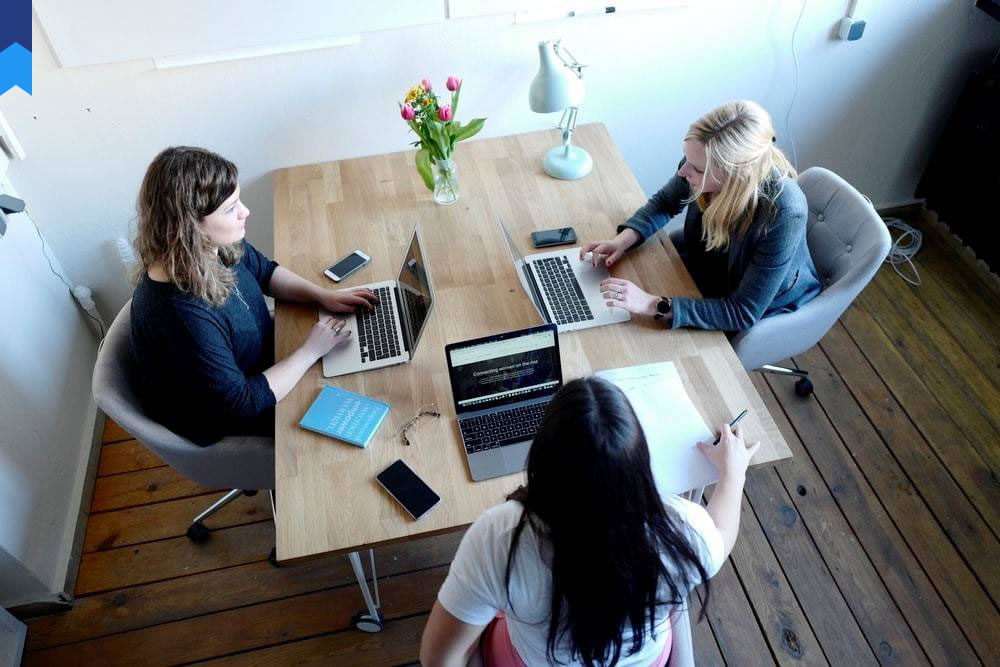
Hidden Truths About AI How-Tos: Mastering The Unseen
Artificial intelligence is rapidly transforming industries, but many practical aspects of its implementation remain shrouded in mystery. This article delves into the hidden truths behind successful AI integration, moving beyond the surface-level tutorials and exposing the nuanced challenges and unexpected solutions that truly drive effective AI deployment. We'll explore the often-overlooked aspects, providing a deeper understanding for those seeking to leverage AI's transformative power.
Data's Dark Side: Unveiling the Unseen Biases
The quality of data is paramount in AI, yet achieving truly unbiased data is an ongoing struggle. Hidden biases within datasets can lead to discriminatory outcomes, undermining the fairness and efficacy of AI systems. Consider facial recognition technology: studies have revealed significant inaccuracies in identifying individuals with darker skin tones, a direct consequence of biased training data predominantly featuring lighter-skinned individuals. This highlights the critical need for meticulous data curation and rigorous bias detection processes.
Another example is in loan applications. AI algorithms trained on historical data reflecting existing societal biases may perpetuate those biases by unfairly denying loans to certain demographic groups. This necessitates the implementation of fairness-aware algorithms and ongoing monitoring to ensure equitable outcomes. Companies like Google are actively researching and developing bias mitigation techniques, demonstrating a growing awareness of this crucial challenge. The challenge lies in not only identifying these biases but also in proactively addressing the root causes in data collection and model design.
Furthermore, the volume of data needed for effective AI training is often underestimated. Many projects fail due to insufficient data, leading to inaccurate or unreliable models. Data augmentation techniques, which artificially increase dataset size, are becoming increasingly important but are not a silver bullet. A sophisticated approach combines data augmentation with careful data selection and cleaning, ensuring that the augmented data truly reflects the patterns within the original data. This process is iterative and often involves expert human oversight.
Case Study 1: A major bank experienced significant backlash after its AI-powered loan application system disproportionately rejected applications from minority groups. The issue stemmed from biased training data that reflected historical lending patterns. This case highlights the critical importance of addressing bias in data before deploying AI systems. Case Study 2: A facial recognition system implemented by a law enforcement agency was found to misidentify individuals with darker skin tones at a significantly higher rate than those with lighter skin tones, underscoring the need for rigorous testing and bias mitigation strategies in sensitive applications.
Model Deployment: Navigating the Unexpected
Deploying an AI model successfully involves more than just training the model; it requires careful planning, execution, and monitoring. Unexpected challenges frequently arise, such as unexpected data drift, where the characteristics of the data change over time, impacting the model's accuracy. Consider a model predicting customer churn: if the company's pricing strategy changes, the model may become obsolete unless continuously updated to reflect these shifts. This necessitates mechanisms for ongoing model monitoring and retraining.
Another challenge involves the integration of AI models into existing systems. This process can be complex and time-consuming, requiring careful consideration of data flow, security, and scalability. A well-structured approach involves a phased rollout, allowing for adjustments based on real-world feedback. Ignoring the integration complexities can lead to significant delays and failures. Robust monitoring systems are essential for detecting anomalies and potential problems during model deployment and operation.
Furthermore, the choice of deployment platform is crucial. Cloud-based solutions offer scalability and flexibility, while on-premise solutions provide greater control over data security. The optimal choice depends on the specific needs of the application and organization. A hybrid approach, combining cloud and on-premise resources, often provides the best balance of flexibility and control. Careful consideration of infrastructure and resource management is critical for efficient and cost-effective AI deployment.
Case Study 1: A retail company experienced significant disruptions after deploying a new AI-powered recommendation engine without properly addressing the integration challenges, resulting in system instability and decreased sales. Case Study 2: A healthcare provider successfully deployed an AI-powered diagnostic tool by adopting a phased rollout approach, gradually expanding its use based on the results of initial trials and feedback from medical professionals.
The Human Element: Beyond the Algorithm
The success of AI isn't solely dependent on sophisticated algorithms; the human element plays a vital role. Effective AI deployment necessitates collaboration between data scientists, engineers, and domain experts. Each team member brings unique perspectives and expertise, contributing to a holistic approach to problem-solving. This collaborative process helps to identify potential biases, address integration challenges, and ensure that the AI system aligns with the organization's goals.
Furthermore, user acceptance is crucial. AI systems must be designed to be intuitive and user-friendly, ensuring seamless integration into workflows. Poor user experience can lead to resistance and ultimately undermine the adoption of the AI system. Considerable attention must be paid to the human-computer interaction aspects of AI deployment, encompassing training, support, and feedback mechanisms. This focus on usability enhances the chances of successful integration.
Ethical considerations are also paramount. AI systems must be developed and deployed responsibly, ensuring that they adhere to ethical guidelines and avoid perpetuating biases or causing harm. Transparency and explainability are essential, allowing users to understand how the AI system makes decisions. This transparency builds trust and encourages responsible use. A strong ethical framework ensures accountability and mitigates potential risks associated with AI deployment.
Case Study 1: A manufacturing company successfully integrated an AI-powered quality control system by working closely with its production team, ensuring that the system aligned with their workflows and addressing their concerns. Case Study 2: A healthcare organization faced challenges deploying an AI-powered diagnostic tool due to a lack of user training and support, highlighting the importance of considering the human element in AI adoption.
The Ever-Evolving Landscape: Adaptability and Future Trends
The field of AI is constantly evolving, with new algorithms, techniques, and technologies emerging regularly. Staying abreast of these advancements is essential for maintaining the competitiveness of AI systems. Continuous learning and adaptation are key to successfully navigating the dynamic landscape. Organizations must invest in training and development programs to ensure their teams have the necessary skills to adapt to new developments.
The increasing use of federated learning, which allows for the training of AI models on decentralized data sources without sharing sensitive information, is reshaping the way AI is developed and deployed. This approach enhances data privacy and security while simultaneously enabling the creation of more robust and accurate models. Federated learning opens up new possibilities for collaboration and data sharing, leading to significant advancements in AI capabilities.
Another important trend is the growing focus on explainable AI (XAI), which aims to make AI systems more transparent and understandable. As AI systems become more complex, the ability to explain their decisions becomes increasingly crucial, especially in sensitive applications such as healthcare and finance. XAI methods improve trust and accountability, ensuring responsible AI deployment. This area is rapidly developing and continues to be a focus of extensive research.
Case Study 1: A financial institution leveraged federated learning to train a fraud detection model across multiple branches, enhancing privacy while improving detection accuracy. Case Study 2: A research team developed an XAI method to explain the decisions of an AI-powered diagnostic tool, improving transparency and facilitating user acceptance.
Maintenance and Monitoring: The Ongoing Commitment
Implementing an AI system is not a one-time event. Successful AI deployment necessitates a commitment to ongoing maintenance and monitoring. Regular updates are essential to address bugs, enhance performance, and adapt to changes in the data or environment. Effective monitoring systems help to identify potential problems and ensure that the AI system continues to function as expected. Neglecting these ongoing tasks can lead to decreased performance, inaccuracies, and even system failures.
Regular model retraining is crucial to maintain accuracy over time. As data changes, models can become outdated, leading to decreased performance. A robust retraining schedule, incorporating new data and addressing data drift, is vital for sustained accuracy. Continuous monitoring allows for timely intervention and adjustments, ensuring the longevity of the AI system. This proactive approach is essential for maximizing the return on investment in AI.
Additionally, security considerations are paramount. AI systems are vulnerable to various security threats, and robust security measures are essential to protect sensitive data and prevent unauthorized access. Regular security audits and updates are essential to mitigate risks and maintain data integrity. A comprehensive security strategy is vital for ensuring the ongoing safety and reliability of the AI system.
Case Study 1: A logistics company experienced significant delays after failing to update their AI-powered route optimization system, highlighting the importance of regular maintenance and updates. Case Study 2: A cybersecurity firm successfully defended against an attack on an AI system by implementing robust security measures and regularly monitoring for suspicious activity.
In conclusion, mastering the art of AI deployment involves much more than simply following basic tutorials. The "hidden truths" discussed—the challenges of biased data, the complexities of model deployment, the importance of the human element, the ever-evolving AI landscape, and the need for ongoing maintenance—must be carefully considered for true success. By addressing these often-overlooked aspects, organizations can unlock the true transformative potential of AI and build truly impactful and reliable AI systems.