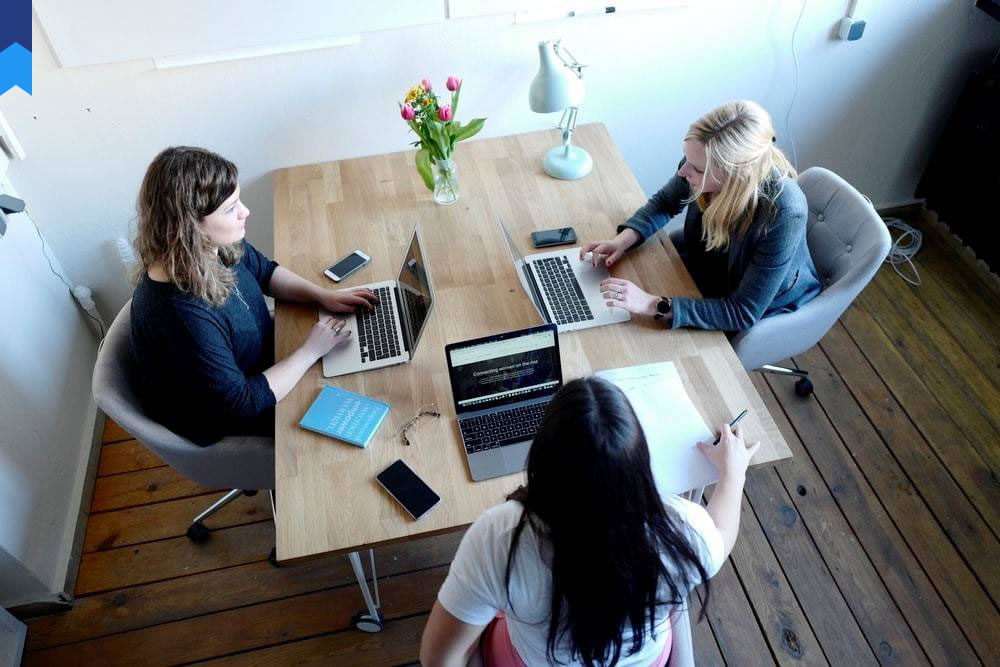
Hidden Truths About AI Integration In Business
The seamless integration of artificial intelligence (AI) into business operations is often portrayed as a straightforward path to increased efficiency and profitability. However, beneath the surface of slick marketing materials and optimistic predictions lie complex challenges, unforeseen consequences, and ethical dilemmas that demand careful consideration. This article delves into the hidden truths of AI integration, exploring the realities beyond the hype and offering practical insights for successful implementation.
The Data Deluge: Unseen Costs and Challenges
The promise of AI is predicated on data – vast quantities of it. Gathering, cleaning, and preparing this data for AI algorithms is a time-consuming and expensive process. Many businesses underestimate the sheer scale of this undertaking. For example, a retail company aiming to implement AI-powered personalized recommendations needs to collect and process transactional data, customer profiles, browsing history, and potentially even social media interactions. This requires substantial investment in data infrastructure, skilled personnel, and data governance frameworks. Failure to adequately address these preliminary steps can lead to inaccurate predictions, biased outcomes, and ultimately, project failure. Case study 1: A major e-commerce retailer invested heavily in AI-powered personalized marketing but failed to account for the significant costs associated with data cleaning and management, resulting in budget overruns and underwhelming ROI. Case study 2: A financial institution underestimated the effort needed to comply with data privacy regulations while building its AI-powered fraud detection system. This caused delays and increased costs substantially.
Furthermore, data quality is paramount. Inaccurate, incomplete, or biased data will lead to flawed AI models, producing unreliable results. This issue is particularly acute in sectors with complex or unstructured data, such as healthcare or legal services. This necessitates rigorous data validation processes, robust data governance frameworks and ongoing monitoring to ensure data quality and prevent biases from perpetuating existing inequalities. This continuous quality check might require retraining the AI periodically, another expense that may not be factored in the initial planning. This emphasizes the crucial role of data scientists, ensuring the data fed into the system is credible and reliable.
Consider the case of a healthcare provider attempting to use AI to predict patient readmission rates. Inaccurate or missing data on patient demographics, medical history, or social determinants of health could lead to unreliable predictions, potentially impacting patient care and resource allocation. The lack of comprehensive and reliable data often renders AI implementations less effective than anticipated, creating a significant obstacle to successful integration.
The cost of maintaining and updating AI systems is another hidden expense. AI models are not static; they require continuous monitoring, retraining, and adaptation to maintain their accuracy and relevance. This ongoing investment is often overlooked in initial cost projections, leading to unexpected budget shortfalls and frustration. In many instances, this requires investment in highly skilled personnel, increasing the overall cost of integration and maintenance.
The Skills Gap: Finding and Retaining AI Talent
Successfully integrating AI requires a skilled workforce capable of designing, implementing, and managing AI systems. This creates a significant challenge for many businesses, as there is a global shortage of AI specialists. Competition for talent is fierce, driving up salaries and making it difficult for companies to find and retain the expertise they need. Furthermore, the skills required extend beyond just data scientists and AI engineers. Businesses also need professionals with expertise in areas such as data management, cybersecurity, and ethical AI.
Case study 1: A technology firm struggled to fill crucial AI engineering roles, delaying its AI-driven product launch and losing market share to competitors who had successfully secured the necessary talent. Case study 2: A manufacturing company invested heavily in AI-powered automation but lacked the necessary in-house expertise to manage the system effectively, resulting in downtime and reduced productivity.
This skills gap is amplified by the rapid pace of technological advancement. New AI techniques and tools are constantly emerging, requiring continuous learning and upskilling of existing staff. Companies need to invest in employee training and development programs to keep their workforce up-to-date with the latest advancements. Otherwise, they risk becoming technologically obsolete and losing their competitive edge.
Beyond technical skills, businesses also need individuals who understand the ethical implications of AI. Bias in algorithms, data privacy concerns, and the potential for job displacement are just some of the ethical considerations that must be addressed. Companies need to cultivate a culture of responsible AI development and deployment to ensure that AI systems are used ethically and responsibly.
Addressing the skills gap requires a multifaceted approach, including investments in education and training, partnerships with universities and research institutions, and initiatives to attract and retain talent. This also includes creating a supportive environment for employees to upskill and learn new technologies. Without addressing this shortage, effective AI implementation and integration remains challenging.
The Ethical Tightrope: Navigating Bias and Responsibility
AI systems are trained on data, and if that data reflects existing societal biases, the resulting AI will likely perpetuate and even amplify those biases. This can lead to unfair or discriminatory outcomes, particularly in areas such as loan applications, hiring processes, and criminal justice. For instance, facial recognition technology has been shown to be less accurate in identifying individuals with darker skin tones, raising concerns about its use in law enforcement. This lack of accuracy can have serious consequences, resulting in misidentification and wrongful accusations.
Case study 1: A loan application algorithm was found to disproportionately deny loans to applicants from certain demographic groups, highlighting the risk of perpetuating existing biases through AI systems. Case study 2: A recruiting tool used by a major technology company was shown to favor male candidates over female candidates, demonstrating the importance of careful oversight and bias mitigation in AI systems.
Moreover, the use of AI raises significant data privacy concerns. AI systems often require access to vast amounts of personal data, raising questions about data security and the potential for misuse. Companies must implement robust data protection measures to ensure the privacy and security of sensitive information. Failure to do so can lead to reputational damage, financial penalties, and legal action. The General Data Protection Regulation (GDPR) in Europe, for example, sets strict standards for data privacy, highlighting the importance of compliance.
The ethical considerations surrounding AI are complex and require careful attention. Companies need to develop ethical guidelines and frameworks for AI development and deployment. These guidelines should address issues such as bias mitigation, data privacy, transparency, and accountability. Companies must also engage with stakeholders, including employees, customers, and regulators, to ensure that their AI systems are used ethically and responsibly. This open dialogue is vital for fostering trust and building public confidence in AI technology.
Regular audits and ethical reviews of AI systems are crucial for ensuring they align with ethical principles. These reviews should be conducted by independent experts and should include assessments of the potential for bias, fairness, and transparency. Continuous monitoring and improvement in AI ethical guidelines are necessary to address emerging challenges and concerns.
Integration Hurdles: Overcoming Technical and Organizational Barriers
Integrating AI into existing business processes can be challenging, requiring significant changes to workflows, systems, and organizational structures. Many companies struggle to effectively integrate AI into their existing infrastructure, leading to incompatibility issues and integration difficulties. For instance, integrating AI-powered analytics tools with legacy systems may require substantial modifications or even replacements of existing infrastructure, a costly and time-consuming process. This often requires significant investments in new hardware and software, as well as retraining of personnel.
Case study 1: A financial institution struggled to integrate its AI-powered fraud detection system with its existing legacy systems, leading to delays and increased costs. Case study 2: A manufacturing company experienced significant downtime and reduced productivity due to integration challenges with its AI-powered automation systems.
Furthermore, integrating AI requires a fundamental shift in organizational culture and mindset. Companies need to create a data-driven culture where data is valued as a strategic asset and where employees are empowered to use data to make informed decisions. This requires investment in training and development, as well as changes to organizational processes and reward systems. A lack of collaboration among departments can impede the integration process, highlighting the importance of a unified approach and clear communication channels.
Many businesses underestimate the time and resources required to successfully integrate AI into their operations. This is often due to a lack of clear understanding of the integration process and the associated challenges. This underestimation frequently results in project delays, budget overruns, and ultimately, project failure. A phased approach to implementation, starting with pilot projects and gradually expanding to larger-scale deployments, can help mitigate these risks. This phased approach helps identify and address potential problems early on, thereby minimizing disruptions and maximizing the chances of successful integration.
Effective communication is crucial throughout the integration process. Companies need to clearly communicate the goals and benefits of AI integration to all stakeholders. This includes explaining the changes that will be required and addressing any concerns or anxieties that employees may have. This clear and consistent communication is vital for building support and buy-in from all stakeholders involved in the integration process, which is essential for success.
Measuring Success: Beyond the Hype and Towards Tangible Results
The true measure of success in AI integration lies not in the technology itself but in its impact on business outcomes. Many companies focus on implementing AI without clearly defining their goals and metrics for success. This lack of clear objectives makes it difficult to evaluate the effectiveness of AI initiatives and demonstrate a clear return on investment. Clear, measurable goals and Key Performance Indicators (KPIs) are crucial for assessing the success of AI initiatives.
Case study 1: A marketing company implemented an AI-powered advertising platform but failed to track its impact on sales conversions, making it difficult to assess the ROI of the investment. Case study 2: A manufacturing company deployed AI-powered predictive maintenance but did not track its impact on equipment downtime, preventing a proper evaluation of the benefits.
Measuring the success of AI integration requires a holistic approach that goes beyond simple metrics such as accuracy or efficiency. Companies need to consider the broader impact of AI on business processes, customer experience, and employee productivity. This broader perspective ensures that the evaluation captures the full range of benefits and challenges associated with AI implementation.
Regular monitoring and evaluation are crucial for ensuring that AI systems are achieving their intended outcomes. Companies need to track key metrics and make adjustments as needed to optimize the performance of their AI systems. This iterative approach to improvement helps ensure that AI systems continuously adapt and improve over time, constantly refining their performance and efficiency.
Finally, communicating the results of AI initiatives is critical for building stakeholder confidence and securing future investment. Companies need to clearly communicate the successes and challenges of their AI initiatives to all stakeholders, highlighting both positive and negative aspects transparently. This transparency helps build trust and ensures accountability, essential elements for maintaining successful long-term AI integration.
In conclusion, the successful integration of AI into business operations requires a multifaceted approach that goes beyond simply adopting the latest technology. Businesses must carefully consider the practical challenges, ethical implications, and organizational changes required for effective AI implementation. By proactively addressing these hidden truths, companies can increase their chances of realizing the true potential of AI and achieving tangible business results.