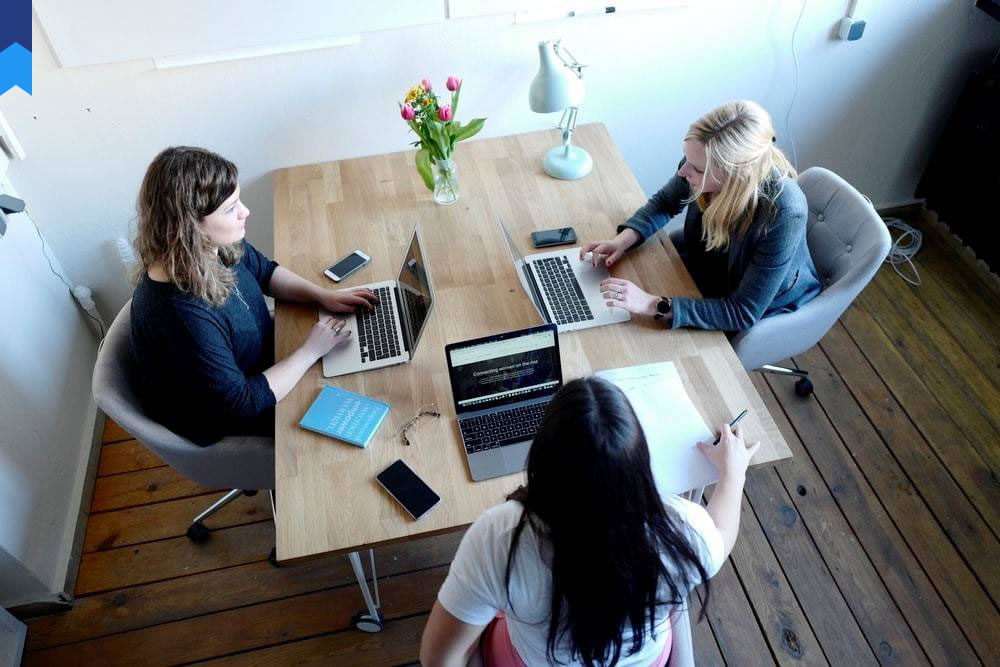
Strategic Approaches To AI-Powered Customer Service
The landscape of customer service is undergoing a dramatic transformation, driven by the rapid advancements in artificial intelligence (AI). No longer a futuristic concept, AI is becoming an indispensable tool for businesses seeking to enhance customer experiences, optimize operational efficiency, and gain a competitive edge. This article explores strategic approaches to leveraging AI in customer service, moving beyond basic implementations to delve into innovative and impactful strategies that redefine how businesses interact with their customers.
AI-Driven Personalization: Crafting Seamless Customer Journeys
Personalization is paramount in today's customer-centric world. AI empowers businesses to deliver hyper-personalized experiences at scale. Natural Language Processing (NLP) allows for the analysis of customer data, including past interactions, purchase history, and preferences, to anticipate needs and proactively offer tailored solutions. For example, an e-commerce platform can use AI to recommend products based on browsing history and purchase patterns. This approach significantly improves customer satisfaction and drives sales conversion.
Case Study 1: Amazon's recommendation engine leverages AI to suggest products based on individual customer profiles, leading to increased sales and enhanced customer engagement. Case Study 2: Netflix utilizes AI to personalize movie and show recommendations, optimizing user experience and driving subscription retention.
Furthermore, AI-powered chatbots can engage in personalized conversations, addressing customer queries with context-aware responses. This approach not only improves response times but also enhances customer satisfaction by providing tailored solutions to specific issues. AI can be used to segment customers based on various factors (demographics, purchase behaviour, engagement level etc.) enabling targeted communication strategies that resonate with specific customer groups.
By incorporating sentiment analysis, businesses gain valuable insights into customer emotions, allowing for proactive issue resolution and the identification of areas for improvement. For instance, AI can detect negative sentiment in customer feedback, prompting immediate intervention to prevent escalation. The ability to predict customer churn through AI analysis allows businesses to implement proactive retention strategies. This data-driven approach is critical in building customer loyalty and reducing churn rates.
Incorporating AI-driven personalization involves more than just basic data analysis; it necessitates a deep understanding of customer behaviours and preferences to create truly seamless and engaging customer journeys. A successful strategy requires robust data infrastructure and advanced AI algorithms capable of handling massive volumes of data. The combination of these elements helps businesses deliver consistently personalized interactions throughout the customer lifecycle. This is more than just efficiency; it's about building stronger relationships and creating loyalty.
AI-Powered Chatbots: Revolutionizing Instant Support
AI-powered chatbots have emerged as a game-changer in customer service, offering instant support and resolving common queries efficiently. These chatbots leverage NLP and machine learning to understand and respond to customer inquiries, reducing the workload on human agents. For example, a bank can use an AI chatbot to guide customers through account balance inquiries, transaction history review, and simple problem-solving tasks. This automated approach minimizes wait times and enhances customer experience.
Case Study 1: Many banks use AI chatbots to handle routine inquiries, improving customer satisfaction and reducing costs. Case Study 2: E-commerce sites employ AI chatbots for order tracking, delivery information, and product inquiries, enhancing convenience for online shoppers.
However, the effectiveness of AI chatbots depends on meticulous design and development. Chatbots should be intuitive, easy to use, and capable of handling a wide range of customer inquiries. Proper training is vital for ensuring accuracy and relevance of responses. If a chatbot is unable to solve a customer's query, it should seamlessly transfer the conversation to a human agent. This ensures that no customer is left unattended.
Beyond basic query resolution, advanced AI chatbots can analyze customer interactions to identify trends and patterns. This data provides valuable insights into common customer issues and areas for improvement in products or services. Continuous improvement and refinement of chatbots based on customer feedback and interaction data are crucial for optimal performance.
Furthermore, integrating AI chatbots with other customer service channels, such as email or phone, creates a holistic and seamless customer experience. The transition between channels should be smooth and effortless, ensuring consistency in interaction and brand identity. Investing in sophisticated AI chatbots presents a significant opportunity to enhance operational efficiency while improving the overall customer experience.
Predictive Analytics: Proactive Problem Solving and Enhanced Efficiency
Predictive analytics, a subset of AI, enables businesses to anticipate customer needs and proactively address potential issues. By analyzing historical data and identifying patterns, businesses can predict customer churn, identify potential service failures, and optimize resource allocation. For instance, a telecommunications company can use predictive analytics to identify customers at high risk of churning and implement targeted retention strategies. This proactive approach can significantly reduce churn rates and improve customer loyalty.
Case Study 1: A major airline uses predictive analytics to anticipate flight delays and proactively communicate with affected passengers, minimizing disruption and improving customer satisfaction. Case Study 2: A retail company utilizes predictive analytics to forecast demand and optimize inventory management, reducing waste and improving efficiency.
Predictive analytics also plays a crucial role in optimizing resource allocation. By analyzing historical data on call center volume, businesses can predict peak demand periods and staff accordingly, ensuring adequate support to handle customer inquiries efficiently. This approach minimizes wait times and improves overall customer satisfaction. Properly implemented, this system significantly reduces customer frustration associated with long wait times.
The use of predictive analytics is not limited to customer service; it extends to other areas of the business. By anticipating potential problems and addressing them proactively, businesses can prevent costly disruptions and enhance operational efficiency. The integration of predictive analytics with other AI technologies, such as machine learning, enhances the accuracy and effectiveness of predictions. This combination creates a powerful tool for businesses aiming to optimize customer service and operational performance.
The value of predictive analytics lies in its ability to transform reactive customer service into a proactive approach. By focusing on preventing problems before they arise, businesses can significantly improve customer satisfaction, reduce operational costs, and enhance their overall efficiency.
Sentiment Analysis: Understanding Customer Emotions and Improving Engagement
Sentiment analysis is a powerful tool for understanding customer emotions and improving engagement. By analyzing customer feedback, including reviews, social media posts, and survey responses, businesses can gain valuable insights into customer sentiment towards their products, services, and brand. For example, a restaurant can use sentiment analysis to monitor online reviews and identify areas for improvement in its food, service, or ambiance. This data-driven approach allows for targeted improvements that enhance customer satisfaction.
Case Study 1: A social media company uses sentiment analysis to track brand perception and address negative feedback, mitigating reputational damage. Case Study 2: An online retailer uses sentiment analysis to understand customer feedback on new products, informing product development and improvement strategies.
Beyond basic sentiment classification (positive, negative, neutral), advanced sentiment analysis techniques can identify specific emotions and nuances in customer feedback. This allows businesses to address customer concerns with empathy and personalized solutions. Moreover, sentiment analysis can help in identifying unmet customer needs or areas where the customer experience falls short of expectations. This information is critical for continuous improvement and enhancing customer satisfaction. Identifying and addressing unmet needs proactively builds a stronger connection with customers.
The integration of sentiment analysis with other AI technologies, such as NLP and machine learning, enhances the accuracy and effectiveness of analysis. The combined analysis provides a comprehensive understanding of customer sentiment and guides strategic decision-making. By understanding customer emotions, businesses can tailor their communications and interactions to foster stronger relationships and improve customer loyalty.
Sentiment analysis is not just about identifying negative feedback; it's about understanding the "why" behind customer emotions. By understanding the underlying reasons for positive or negative sentiment, businesses can improve their products, services, and overall customer experience. This data-driven approach is critical for continuous improvement and staying ahead in a competitive market.
Ethical Considerations and Data Privacy in AI-Powered Customer Service
As AI becomes increasingly integrated into customer service, ethical considerations and data privacy become paramount. Businesses must ensure responsible use of customer data, adhering to relevant regulations and guidelines. Transparency is crucial, informing customers about the use of AI in their interactions and providing mechanisms for data access and control. For example, businesses should clearly state how customer data is used for personalization and provide options for opting out of data-driven personalization. Transparency builds trust and enhances customer confidence in the business.
Case Study 1: A company suffered reputational damage due to a data breach, highlighting the importance of robust data security measures. Case Study 2: A company implemented robust data privacy measures, building customer trust and enhancing brand reputation.
Data security is critical in protecting sensitive customer information. Businesses must invest in robust security measures to prevent data breaches and unauthorized access. AI algorithms should be designed to minimize bias and ensure fairness in customer interactions. AI systems should be regularly audited to identify and address any potential biases that could lead to unfair or discriminatory outcomes. This ongoing monitoring ensures ethical operations and prevents any negative impacts on customers.
Moreover, businesses should establish clear guidelines for the use of AI in customer service, ensuring that AI systems are used ethically and responsibly. This involves considering the potential impact of AI on jobs and ensuring that human oversight remains integral to the customer service process. AI is a tool meant to assist human agents, not replace them entirely. A balance must be found.
Addressing ethical considerations and data privacy is not merely a compliance issue; it's a matter of building trust and maintaining customer loyalty. Businesses that prioritize ethical AI practices will build stronger relationships with their customers and gain a competitive advantage in the long term. This proactive approach is critical in the current climate of increased scrutiny and heightened customer awareness regarding data privacy.
Conclusion
The strategic integration of AI into customer service is no longer a luxury but a necessity for businesses seeking to thrive in today's competitive landscape. By embracing innovative approaches to AI-powered personalization, chatbots, predictive analytics, and sentiment analysis, while adhering to the highest ethical standards and data privacy regulations, businesses can create truly exceptional customer experiences. This data-driven approach allows for continuous improvement, driving customer loyalty and operational efficiency. The future of customer service lies in the seamless integration of human expertise with the capabilities of AI, creating a powerful synergy that benefits both businesses and their customers.
The journey towards AI-driven excellence in customer service is an ongoing process, requiring continuous adaptation and refinement. By staying abreast of emerging trends and best practices, businesses can leverage the full potential of AI to enhance customer satisfaction and achieve lasting success. The key lies in a thoughtful, strategic approach, balancing technological advancements with human-centered values. This approach positions businesses to create meaningful connections with their customers, fostering long-term loyalty and driving sustainable growth.