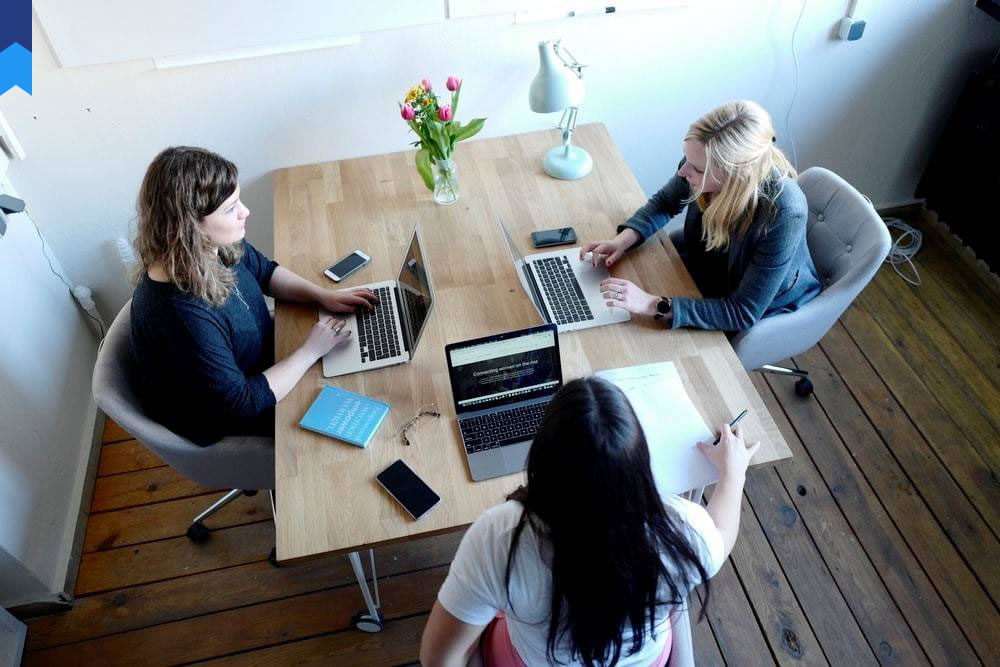
The Counterintuitive Guide To Data Analytics Success
Data analytics is often portrayed as a straightforward path to insights. However, true mastery requires embracing counterintuitive strategies. This guide unravels unconventional approaches to achieve data-driven success, challenging common assumptions and revealing hidden pathways to impactful results.
Embrace the Messy Data First
Contrary to popular belief, the pursuit of perfectly clean data can be a significant time sink, often delaying actionable insights. Instead, prioritize getting started with the data you have, even if it's messy. Iterative cleaning and refinement, alongside quick analyses, can be more efficient than aiming for perfection upfront. This approach allows for the rapid identification of valuable patterns and trends, informing further data cleansing efforts. Case study 1: A startup successfully launched a new product line by analyzing initially messy customer feedback data, revealing unmet needs despite data inconsistencies. Case study 2: A large retailer utilized a quick initial analysis of incomplete sales data to identify a sudden surge in demand for a specific product, allowing for timely restocking and increased sales.
Begin by focusing on the core questions you are trying to answer. Prioritize data elements directly relevant to these questions. Employ automated data cleaning tools to initially address superficial issues such as inconsistencies in formatting or missing values. Remember, the goal is to gain initial insights, not to achieve perfect data hygiene immediately. This agile approach enables faster iterations and reduces project delays.
Furthermore, embrace the opportunity presented by incomplete data. In many scenarios, missing information can itself be a source of insights. For instance, a high number of missing values for a particular field may indicate a problem in data collection or a gap in understanding customer preferences. Addressing these gaps can significantly enhance data quality and lead to more effective analyses. The ability to efficiently utilize imperfect data allows for more expedient generation of insights.
It’s crucial to document your data cleaning decisions. This is more than simply recording the steps taken; it is about creating a clear understanding of how data transformations and choices affected the findings. This documentation is essential for ensuring that the data is reproducible and verifiable. By prioritizing early analysis over perfect data, you can significantly accelerate the value obtained.
Prioritize Intuition Before Automation
While automation is essential in modern data analytics, it should not replace human intuition. The initial stages of data exploration often require a keen understanding of the data’s context, and this is best achieved through hands-on exploration and insightful questioning. Relying solely on automated processes can obscure valuable patterns and insights. Case study 1: A financial analyst, using intuition, identified an unusual pattern in transaction data that automated fraud detection systems missed. Case study 2: A marketing team used their intuition to segment customers based on purchasing behavior, outperforming algorithmic segmentation approaches.
Humans possess a unique ability to detect subtle patterns and anomalies, often overlooked by automated algorithms. This innate ability is crucial in identifying potential biases, inconsistencies, or unexpected relationships within data. By prioritizing intuition, data analysts can often uncover valuable insights that would otherwise remain hidden. A crucial aspect of intuition is experience, which builds an analyst's capacity to make swift, effective judgements based on a large amount of prior data evaluation.
The integration of intuition and automation is crucial to successful data analysis. Automation handles repetitive tasks, enabling analysts to focus on more complex tasks which require critical thinking and nuanced interpretation. By blending these two approaches, analysts harness the power of both human insight and computational speed.
Effective visualization aids intuition. Data visualization is not simply about presenting information; it is about creating a framework for analysts to recognize patterns and to discover areas deserving of deeper investigation. Creating meaningful visuals aids in rapid intuition and the generation of new ideas.
Fail Fast, Fail Often
In the world of data analytics, failure is not the opposite of success; it's a stepping stone. Experimentation with different methodologies, and even calculated risks, is vital for achieving significant breakthroughs. Fearing failure can stifle innovation and limit the potential for significant discoveries. Case study 1: A research team’s initial hypothesis failed, but their findings led to a completely new and more successful approach. Case study 2: A marketing team’s A/B testing campaign, despite encountering several “failed†variations, ultimately resulted in a significant improvement in conversion rates.
The iterative process of data analysis is predicated upon learning from failures. Each failed attempt provides valuable feedback that can be used to refine methods and improve future outcomes. By embracing a culture of experimentation, data analysts can significantly improve the likelihood of discovering impactful insights.
This perspective promotes a culture of continuous learning. Analysts must be comfortable with the possibility of mistakes; they should understand that failure is often an integral part of the learning process. Moreover, the ability to learn from past errors is essential for continuous development.
It’s crucial to establish clear metrics for success and failure. This means defining specific, measurable, achievable, relevant, and time-bound (SMART) goals at the outset of a project. This process allows analysts to evaluate their work more objectively and learn from any deficiencies.
Communicate Visually, Not Just Verbally
Data analytics is not just about crunching numbers; it's about communicating insights effectively. While technical expertise is essential, the ability to convey complex information in a clear, concise, and engaging manner is equally crucial. This requires mastering visual communication techniques, which often surpasses verbal communication in its impact. Case study 1: A company using compelling data visualizations increased investor confidence and secured funding. Case study 2: A marketing team, through effective data visualizations, successfully persuaded management to change strategy.
Visualizations can transform complex data into easily understandable stories. They allow stakeholders to grasp key trends and patterns without needing extensive data analysis expertise. By visually presenting insights, analysts can increase the adoption and impact of their findings, leading to more effective decision-making.
The most effective visualizations are tailored to the audience. For example, a technical audience may appreciate detailed charts and graphs, whereas a non-technical audience might benefit from simpler, more narrative-driven visuals. The presentation method should suit the audience’s level of understanding and their needs.
Remember that visual communication is not just about aesthetics; it’s about clarity and accuracy. Visually communicating results should not distort or misrepresent data. It is paramount that the visuals accurately reflect the underlying data while improving understanding.
Focus on Impact, Not Just Analysis
The ultimate goal of data analytics is not simply to generate reports; it's to drive action and achieve tangible results. This requires shifting the focus from the technical aspects of analysis to the practical implications of findings. Prioritizing impact ensures that analytical efforts directly contribute to organizational objectives. Case study 1: A retail company, through data analysis, optimized its supply chain, leading to significant cost savings. Case study 2: A healthcare provider, using data analysis, improved patient care, reducing readmission rates.
Align data analysis efforts with strategic business goals. This means setting clear objectives from the beginning, ensuring that the questions being answered are directly relevant to the organization's success. It's critical to consistently evaluate the value derived from data projects, and modify direction if it deviates from intended outcomes.
Data-driven decision making is about empowering stakeholders with information. It’s not about analysts making decisions for others; it’s about providing stakeholders with the tools to make informed choices. This requires clearly communicating findings and highlighting their practical implications.
Continuous monitoring and evaluation are critical to ensuring that data-driven decisions are effective. It is vital to assess the results, make adjustments as needed, and ensure that data analysis activities create tangible improvements.
Conclusion
Mastering data analytics requires embracing a counterintuitive approach. By prioritizing the messy data first, valuing intuition alongside automation, embracing failure, communicating visually, and focusing on impact, data analysts can unlock the true potential of their work and drive substantial organizational success. This unconventional guide offers a pathway to achieve data-driven success, challenging conventional wisdom and revealing unexpected routes to impactful results. The journey towards becoming a truly effective data analyst is an iterative process of learning, adapting, and refining techniques—a continuous cycle of improvement guided by both experience and insight.