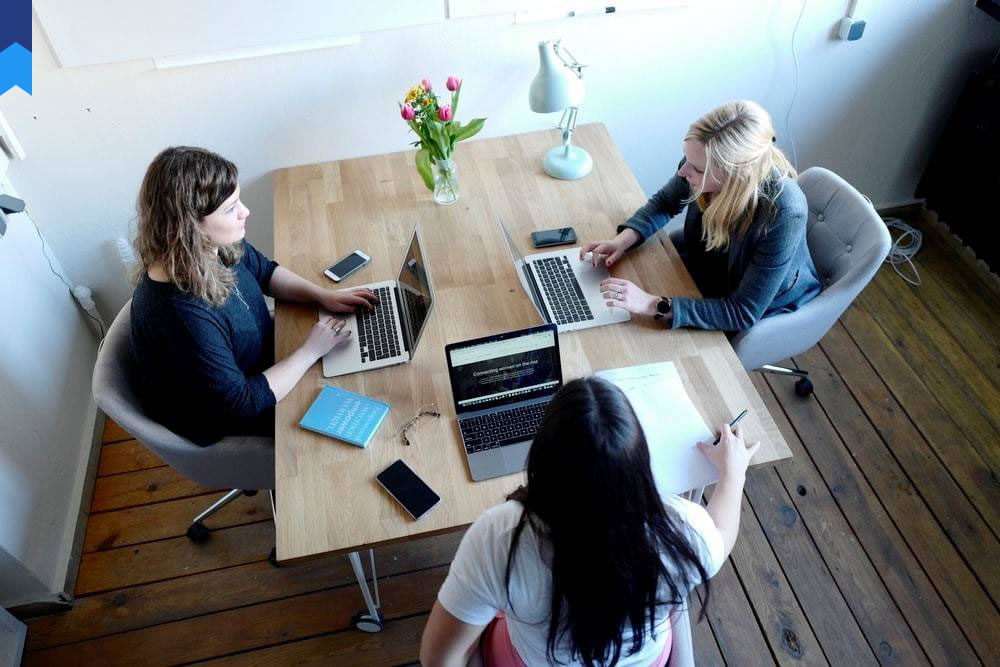
The Hidden Mechanics Of AI-Driven Cybersecurity
The digital landscape is a battleground. Cybersecurity isn't just about firewalls and antivirus software anymore; it's a complex interplay of artificial intelligence, machine learning, and human ingenuity. This article delves into the unseen mechanisms driving this technological arms race, exploring the innovative ways AI is reshaping our defenses against ever-evolving cyber threats.
AI-Powered Threat Detection: Beyond the Signatures
Traditional cybersecurity relied heavily on signature-based detection, identifying known malware through predefined patterns. This approach is inherently reactive, always playing catch-up with sophisticated attackers. AI, however, offers proactive threat detection. Machine learning algorithms can analyze network traffic, user behavior, and system logs, identifying anomalies that deviate from established baselines. These anomalies, often invisible to human analysts, can be indicative of malicious activity. For instance, a sudden spike in unusual network requests from a specific IP address might signal a Distributed Denial of Service (DDoS) attack. AI algorithms can detect these subtle changes in real time, allowing security teams to respond before significant damage occurs.
Case Study 1: A major financial institution implemented an AI-driven security system that detected and neutralized a sophisticated phishing campaign targeting its employees before any significant data breach occurred. The AI system identified subtle inconsistencies in email headers and sender behavior that human analysts had overlooked. Case Study 2: A global e-commerce company used AI-powered threat intelligence to predict and mitigate a series of ransomware attacks. By analyzing past attack patterns and correlating them with real-time data, the system accurately predicted the timing and target of the attacks, allowing the company to proactively strengthen its defenses.
Furthermore, AI can enhance the effectiveness of existing security tools. Intrusion detection systems (IDS) and intrusion prevention systems (IPS) can be augmented with AI to improve accuracy and reduce false positives. AI-powered sandboxing techniques can safely analyze suspicious files and applications, identifying malicious code without the risk of infection. This proactive approach helps to shift the focus from reactive threat mitigation to preventative measures, significantly reducing the impact of potential cyberattacks.
Moreover, AI algorithms constantly learn and adapt, refining their threat detection capabilities over time. The more data they process, the better they become at identifying subtle patterns and predicting future attacks. This continuous learning process is crucial in the ever-evolving world of cybersecurity, where attackers constantly develop new techniques to evade detection.
Automated Incident Response: Speed and Efficiency
Responding effectively to a cyberattack requires speed and precision. Manual incident response processes can be slow and cumbersome, allowing attackers to cause significant damage before containment measures can be implemented. AI can automate many aspects of incident response, accelerating the process and minimizing the impact of attacks. AI-powered security information and event management (SIEM) systems can automatically detect and triage security alerts, prioritizing critical incidents and routing them to the appropriate security teams. AI can also automate the process of isolating infected systems, preventing the spread of malware, and restoring compromised data.
Case Study 1: A large healthcare provider leveraged AI-powered incident response automation to contain a ransomware attack within minutes. The system automatically identified the source of the infection, isolated the affected systems, and initiated a data recovery process, minimizing patient data exposure and service disruption. Case Study 2: A multinational corporation used AI to automatically remediate thousands of low-level security vulnerabilities across its global network. This automated patching system reduced the risk of exploitation and significantly streamlined the security operations team's workload. This automation not only speeds up responses but also reduces human error, a critical factor in successful incident management.
AI-driven incident response solutions are not merely about automating routine tasks. They also allow security teams to focus on more complex and strategic challenges. By automating the handling of routine incidents, AI frees up human analysts to investigate more sophisticated threats and develop more robust security strategies. This synergy between human expertise and artificial intelligence is key to building a truly effective cybersecurity posture.
The efficiency gains from automated response are substantial. Statistics show that AI-powered systems can reduce mean time to resolution (MTTR) for security incidents by up to 70%, significantly reducing the overall cost of a breach.
Predictive Threat Intelligence: Anticipating the Next Attack
Predictive threat intelligence is arguably the most significant advancement in cybersecurity enabled by AI. By analyzing vast quantities of data from diverse sources, AI can identify patterns and predict future attacks with remarkable accuracy. This allows organizations to proactively strengthen their defenses before attacks occur, significantly reducing their vulnerability to cyber threats. AI algorithms can analyze various datasets, including dark web activity, open-source intelligence, and threat feeds, to identify emerging threats and predict attack vectors.
Case Study 1: A major telecommunications company used AI-powered predictive threat intelligence to anticipate and mitigate a sophisticated social engineering campaign targeting its customer base. The AI system identified a pattern of malicious activity on social media platforms, indicating that attackers were planning to exploit customer data. Case Study 2: A large financial institution used AI to predict and prevent a series of account takeover attacks by analyzing user behavior and identifying anomalies in login attempts.
This predictive capability allows security teams to prioritize resources effectively, focusing on the most likely threats. Predictive threat intelligence helps organizations proactively patch vulnerabilities, train employees on emerging threats, and adjust their security strategies based on forecasted risks. The accuracy of predictive models improves over time as the AI system learns from past attacks and incorporates new data sources.
Experts suggest that predictive threat intelligence will become increasingly crucial in a world of ever-evolving cyber threats. The ability to anticipate and mitigate attacks before they occur is no longer a luxury but a necessity for organizations of all sizes.
AI-Enhanced Vulnerability Management: Proactive Patching and Remediation
Vulnerability management is a critical aspect of cybersecurity, involving the identification, assessment, and remediation of software and hardware vulnerabilities. AI can significantly enhance vulnerability management by automating the process of identifying vulnerabilities, prioritizing them based on risk, and recommending appropriate remediation strategies. AI-powered vulnerability scanners can analyze code bases and systems to identify weaknesses that might be overlooked by human analysts. They can also correlate vulnerabilities with threat intelligence data, assessing the likelihood of exploitation and prioritizing the most critical vulnerabilities for immediate remediation. This proactive approach to vulnerability management is crucial in reducing the attack surface and minimizing the risk of exploitation.
Case Study 1: A global technology company used AI-powered vulnerability scanning to identify and remediate hundreds of critical vulnerabilities across its software development lifecycle. The AI system reduced the time it takes to identify and fix vulnerabilities, significantly improving its overall security posture. Case Study 2: A large financial institution integrated AI-powered vulnerability management into its DevOps pipeline, ensuring that security vulnerabilities were addressed before code was deployed to production environments.
AI helps prioritize patching efforts based on the likelihood of exploitation and the potential impact of a successful attack. This allows organizations to focus on the most critical vulnerabilities, optimizing their security resources and reducing the overall risk profile. Moreover, AI can automate the process of deploying security patches, reducing the manual effort required for remediation.
The integration of AI into vulnerability management is a significant step towards a more proactive and efficient approach to cybersecurity. It enables organizations to stay ahead of the curve, reducing the time and resources required to secure their systems.
Ethical Considerations and the Human Factor
While AI offers significant advantages in cybersecurity, it’s crucial to acknowledge the ethical considerations and limitations. AI systems are only as good as the data they are trained on; biased or incomplete data can lead to inaccurate results. Moreover, the reliance on AI can lead to complacency, creating a false sense of security and potentially overlooking critical threats. Human oversight is essential to ensure that AI systems are used responsibly and effectively.
Case Study 1: A company experienced a significant data breach due to an AI-driven security system failing to identify a previously unknown type of malware because the training data lacked similar samples. Case Study 2: Another organization saw a delayed response to a cyberattack because security personnel over-relied on the AI system's analysis and failed to investigate anomalies flagged by human analysts.
The human element remains vital. Security professionals need to understand how AI systems work, interpret their output critically, and maintain oversight of the overall security posture. The effectiveness of AI in cybersecurity is not simply about replacing human analysts but enhancing their capabilities and empowering them to make better-informed decisions. Continuous training and education are crucial to bridging the gap between human expertise and artificial intelligence in a responsible and ethical way.
Furthermore, transparency and explainability in AI-driven security tools are essential for building trust and ensuring accountability. Understanding how an AI system arrives at its conclusions is crucial for building confidence in its decisions and mitigating potential biases. Striking a balance between leveraging AI's power and preserving the human element is essential for building a resilient and ethical cybersecurity strategy.
Conclusion
The integration of AI into cybersecurity is not just a technological advancement; it's a fundamental shift in how we approach the digital arms race. AI-powered tools offer unparalleled capabilities in threat detection, incident response, predictive analysis, and vulnerability management. However, success hinges on a balanced approach, combining the power of AI with the critical thinking and ethical considerations of human expertise. The future of cybersecurity will likely depend on a symbiotic relationship between human analysts and intelligent machines, ensuring that organizations are equipped to proactively address the increasingly sophisticated challenges of the digital age.
As technology continues to evolve, so too will the cyber threats it faces. A holistic approach is necessary, one that embraces innovation while addressing the ethical and practical implications of deploying AI in a security context. This will involve continuous learning, adaptation, and a robust understanding of both the technological and human components of cybersecurity.