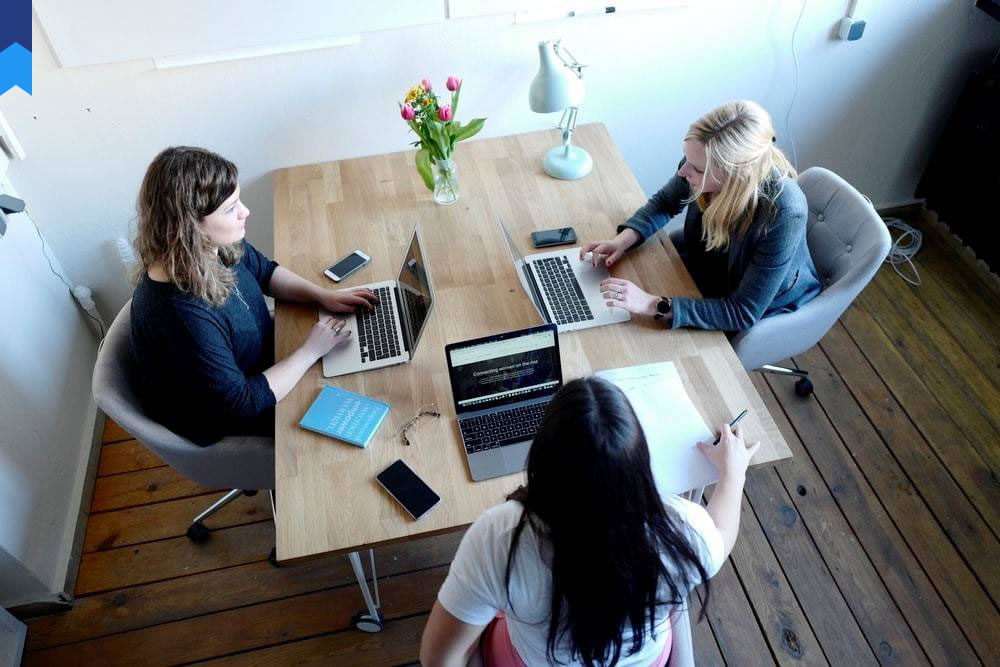
The Hidden Mechanics Of Facebook's Algorithm
Facebook's algorithm, the unseen force shaping our newsfeeds, is far more complex than most realize. It's not just a simple ranking system; it's a constantly evolving machine learning model, influenced by countless factors beyond the likes and shares we readily observe. Understanding its hidden mechanics is key to navigating the platform effectively, whether you're a user, a business, or a researcher.
Understanding the Core Principles
At its heart, Facebook's algorithm aims to show users content it believes they will find most engaging. This engagement is measured through a variety of signals, including the time spent viewing a post, whether a user clicks on a link, shares a post, comments, or reacts with an emoji. The more engagement a post receives, the higher its ranking in the newsfeed. However, the system doesn't simply prioritize popularity. It also considers factors like the relationship between the user and the poster, the type of content, and the user's past behavior. This intricate interplay of variables creates a dynamic system constantly adjusting to individual preferences. For instance, a post from a close friend might receive preferential treatment over a viral video, even if the video has significantly more engagement overall. Case study: A local bakery posting about a new cake versus a celebrity's post, despite the celebrity having millions of followers.
Furthermore, the algorithm isn't static. It undergoes continuous updates and refinements, learning from user interactions and adapting to evolving trends. This means the factors influencing newsfeed placement are constantly shifting, making it difficult to predict exactly how a post will perform. Facebook's engineers constantly tweak the parameters, introducing new signals and weighting existing ones differently, all in an attempt to optimize user experience and keep people engaged. This dynamic nature makes understanding the algorithm a continuous process of learning and adaptation. Consider the evolution from prioritizing chronological feeds to personalized ranking: a significant shift driven by algorithmic adjustments. Another case study: A niche interest group observing a sudden decrease in reach despite consistent engagement, indicating an algorithmic change affecting their specific niche.
The algorithm isn't purely driven by engagement; it also takes into account factors related to the quality of content. This involves combating the spread of misinformation and spam, prioritizing credible sources, and promoting positive interactions. Facebook employs sophisticated techniques to identify and downrank low-quality content, including posts with clickbait titles, misleading information, or hate speech. This is a constantly evolving battle, with malicious actors continually finding new ways to circumvent the algorithm. However, Facebook continues to improve its detection methods, incorporating machine learning models that learn from previous instances of misinformation. For example, an article about a false medical breakthrough could be flagged and given a lower ranking. Another example: A brand account noticing a reduction in visibility due to using excessively promotional language, highlighting the algorithm's preference for authentic engagement.
Finally, the algorithm also considers the user's network. The people you interact with regularly, the groups you're a part of, and the pages you follow all influence what appears in your newsfeed. Posts from your close friends and family are generally given higher priority than content from strangers or pages you rarely engage with. This prioritization reflects Facebook's goal of fostering meaningful connections. For example, a post from a close friend's birthday might be prioritized over news updates, even if the news update is receiving significantly more engagement. Another example: users noticing a higher prevalence of posts from their family members over more widely shared public posts.
The Role of User Preferences
User preferences, while often implicit, play a crucial role in shaping the algorithm's output. Facebook tracks user behavior meticulously, observing which types of content they interact with most frequently. This data informs the algorithm's understanding of individual interests and preferences. For instance, frequent engagement with political news articles might lead to a higher prevalence of such articles in the newsfeed, even if those articles have fewer overall likes and shares compared to other types of content. This personalized approach allows for a highly targeted newsfeed experience. Case study: A user who consistently engages with cooking videos will likely see more cooking-related content, even if those videos have less overall engagement than other types of content.
However, this personalized approach isn't without its drawbacks. Filter bubbles, where users are primarily exposed to information reinforcing their existing beliefs, can arise. This phenomenon can limit exposure to diverse perspectives and lead to echo chambers where dissenting opinions are marginalized. Facebook acknowledges this concern and is actively working to address it, but the inherent nature of personalized algorithms makes it a challenging problem to solve completely. Case study: A user primarily following news sources from one political perspective may miss out on crucial information from alternative viewpoints. Another case study: a user who only interacts with positive content might not see any content that could trigger more varied engagement.
Understanding how the algorithm interprets user preferences is vital for individuals seeking to effectively utilize Facebook. While it's impossible to fully control the algorithm, users can influence it by actively engaging with content they want to see more of. This involves liking, commenting, sharing, and reacting to posts that align with their interests. Conversely, ignoring or hiding content will signal to the algorithm that it's less relevant. For example, repeatedly liking posts from a particular page will increase the likelihood of seeing more content from that page. Another case study: ignoring posts from specific groups may eventually result in reduced visibility of content from similar groups.
Beyond active engagement, users can also leverage Facebook's features to refine their newsfeed experience. This includes using the “snooze†function to temporarily hide posts from certain sources, following pages relevant to their interests, and utilizing the "See First" option to prioritize specific friends or pages. These tools provide users with a degree of control over the algorithm's output, allowing for a more personalized and curated experience. For example, a user overwhelmed by news updates might use the “snooze†function to reduce their frequency. Another case study: A user who prefers updates from close friends and family might prioritize them using the "See First" function.
The Impact on Businesses
Facebook's algorithm has profound implications for businesses seeking to leverage the platform for marketing and advertising. Understanding its mechanics is crucial for creating effective campaigns that reach the target audience. Simply posting content isn't enough; businesses need to tailor their strategy to the algorithm's prioritization factors. For example, creating visually appealing, engaging content that encourages interaction is vital. Case study: A company posting high-quality images and videos instead of only textual updates, maximizing engagement potential.
Businesses must also consider the importance of targeting. Facebook's advertising platform allows for precise targeting based on demographics, interests, and behaviors. This allows businesses to reach their ideal customers effectively, maximizing the return on investment for their advertising spending. Effective targeting helps minimize wasted ad spend and ensures maximum reach to the desired customer segments. Case study: A clothing brand targeting ads to users interested in fashion and specific styles, reducing ad waste and improving campaign ROI. Another case study: a software company tailoring ads to users with technical backgrounds, increasing the likelihood of conversion.
Organic reach, or the visibility of unpaid content, is increasingly challenging on Facebook. The algorithm prioritizes content that generates engagement, meaning businesses must focus on creating high-quality content that encourages interaction from their followers. This requires an understanding of their target audience's preferences and tailoring content accordingly. Case study: A local restaurant posting engaging photos and videos of its food to encourage user interaction and increased organic reach. Another case study: a non-profit organization using interactive polls and questions to maximize their organic reach.
In addition to organic content, businesses utilize Facebook's paid advertising options to further enhance their reach. Paid advertising allows businesses to bypass some of the algorithm's limitations and ensure their content is seen by their target audience. However, effective paid advertising requires a thorough understanding of the algorithm's targeting capabilities and careful optimization to achieve the desired results. Case study: A small business using Facebook ads to reach potential customers in their geographic area. Another case study: a large corporation using sophisticated targeting techniques to reach diverse customer segments.
Addressing Algorithmic Bias and Transparency
The Facebook algorithm, despite its sophistication, isn't free from bias. The data used to train the algorithm can reflect existing societal biases, leading to skewed outcomes. For example, if the training data over-represents certain demographics, the algorithm might inadvertently prioritize content from those groups, further marginalizing others. Addressing algorithmic bias requires careful attention to data collection and model training, and continuous monitoring for potential disparities. Case study: Analyzing the algorithm's impact on different demographic groups to identify potential biases. Another case study: Reviewing training datasets to ensure adequate representation of diverse viewpoints and demographics.
Transparency is another key area of concern. The complexity of the algorithm makes it difficult for users to fully understand how it works, leading to a lack of accountability. While Facebook has made efforts to improve transparency, providing more information about the algorithm's inner workings is crucial for building trust and ensuring fairness. This involves clear communication of how the algorithm functions and the factors influencing content ranking. Case study: Facebook releasing more detailed documentation on algorithm functionalities and decision-making processes. Another case study: conducting public audits and evaluations of the algorithm's effectiveness and fairness.
The issue of misinformation and its spread through the algorithm also demands attention. Combating the spread of false or misleading information requires a multi-pronged approach, including improved detection mechanisms, fact-checking initiatives, and user education. Facebook's efforts to combat misinformation are ongoing, but the rapid evolution of misinformation techniques necessitates continuous adaptation. Case study: Facebook's partnership with independent fact-checking organizations. Another case study: improving the platform's ability to identify and remove misleading content.
Ultimately, ensuring fairness and accountability requires a collaborative effort involving Facebook, researchers, policymakers, and users. Open dialogue and continued research are vital for addressing the ethical challenges posed by powerful algorithms. Developing clear guidelines and regulations can promote responsible algorithm design and prevent the exacerbation of existing biases. Case study: Open forums and collaborations between Facebook and academic researchers to study and improve the algorithm's fairness. Another case study: engagement with policymakers and regulators to establish guidelines for responsible algorithm development.
Conclusion
Facebook's algorithm is a powerful and complex system that significantly shapes our online experiences. Understanding its hidden mechanics—from the core principles of engagement and user preferences to its impact on businesses and ethical considerations—is crucial for navigating the platform effectively. While the algorithm's constant evolution presents challenges, recognizing its intricacies empowers users and businesses to leverage its potential while mitigating its limitations. Increased transparency and ongoing efforts to address algorithmic bias are critical to fostering a fair and responsible online environment.
The future of Facebook’s algorithm hinges on continuous improvement and adaptation. Ongoing research into fairness, transparency, and the effective combating of misinformation will be paramount in maintaining a positive and trustworthy user experience. The evolution of the algorithm will likely involve more sophisticated machine learning techniques, increased emphasis on user privacy, and a greater focus on community well-being. Ultimately, the success of the algorithm will depend on its ability to balance personalization with broader societal goals.