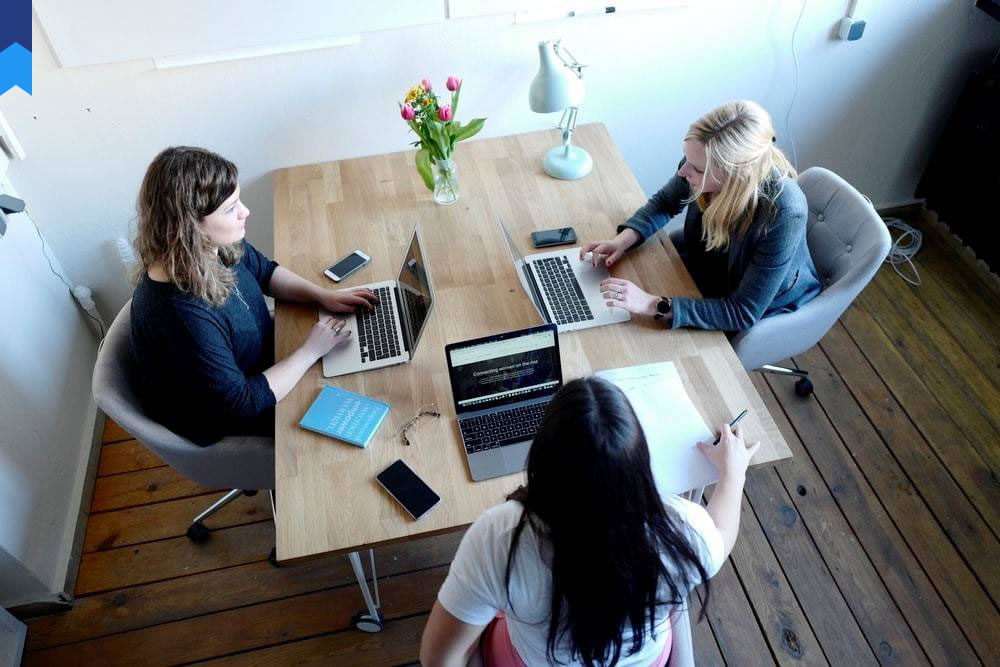
The Hidden Mechanics Of Video Compression
Video compression: a silent powerhouse behind every streamed movie, every viral clip, and every video call. We interact with it daily, yet rarely understand its intricate workings. This article delves into the hidden mechanics of video compression, revealing the surprising science and engineering that allows us to seamlessly consume vast amounts of visual data.
Understanding the Fundamentals of Video Compression
At its core, video compression is about reducing the size of video files without significantly impacting their visual quality. This is achieved by exploiting redundancies and irrelevancies within the video data. Consider a scene with a static background: Why transmit the same background information frame after frame? This is where compression techniques excel. They identify these redundancies and cleverly represent them using fewer bits of data. Lossy and lossless compression methods exist. Lossless compression, such as PNG for images, guarantees perfect reconstruction of the original data, but achieves less compression. Lossy compression, like JPEG or MP4 for video, discards some data to achieve a significantly smaller file size, resulting in some loss of quality. This trade-off between compression ratio and quality is a central challenge in video compression.
Several key concepts drive efficient compression. Spatial compression reduces redundancy within individual frames. Techniques like Discrete Cosine Transform (DCT) decompose images into frequency components, allowing the elimination of less important high-frequency details. Temporal compression exploits redundancies between successive frames. Techniques like motion estimation and compensation track moving objects and only encode the changes between frames. This dramatically reduces the data required. Predictive coding uses previous data to predict future data, efficiently encoding the difference (residual) between prediction and actual data. This significantly lowers the bitrate.
Case Study 1: The evolution of codecs. Early codecs like MPEG-1 were relatively inefficient. Subsequent codecs, like H.264/AVC, used more sophisticated algorithms like improved motion estimation and more efficient quantization, leading to significantly smaller file sizes for the same quality. Case Study 2: The impact of codec choice on streaming services. Netflix, for example, uses sophisticated algorithms to select the optimal codec and bitrate based on network conditions and device capabilities, ensuring a high-quality viewing experience while minimizing bandwidth consumption.
The choice between different compression algorithms impacts the final quality and file size. H.264, once the dominant standard, offers a good balance between compression efficiency and computational complexity. However, newer codecs like H.265 (HEVC) and VP9 achieve significantly higher compression ratios at the same quality level, although they may require more processing power to decode. This constant evolution reflects the ongoing research and technological advances in this field.
The Role of Codecs and Standards
Codecs (coder-decoders) are the software and hardware implementations of compression and decompression algorithms. They are crucial for translating raw video data into compressed files and back again. The choice of codec significantly impacts the final file size, quality, and computational demands. Different codecs employ various algorithms to achieve compression, resulting in varying degrees of efficiency and visual fidelity. Some codecs prioritize compression efficiency, potentially sacrificing some quality, while others focus on preserving visual quality at the cost of larger file sizes.
Standardization plays a critical role in ensuring interoperability between different devices and platforms. Standardized codecs, like H.264 and H.265, guarantee that compressed videos can be played across a wide range of devices. Without standardization, each device would require its own unique codec, leading to incompatibility issues. The ongoing development and refinement of standards reflect the constantly evolving needs of the industry.
Case Study 1: The impact of AV1. AV1, a royalty-free codec, is emerging as a strong competitor to existing standards, offering superior compression efficiency. Its open nature fosters collaboration and innovation, potentially leading to further improvements in video compression technology. Case Study 2: The challenges of codec adoption. The transition to new codecs requires substantial investment in hardware and software updates. This can be a significant barrier to wider adoption, especially in older devices.
The future of codecs involves exploring more sophisticated compression algorithms, incorporating artificial intelligence (AI) to improve efficiency, and adapting to the ever-growing demands of higher resolutions and frame rates. Advanced techniques like deep learning are being investigated to further optimize compression and enhance the quality of compressed video.
Advanced Compression Techniques
Beyond the foundational methods, advanced techniques push the boundaries of video compression. These leverage sophisticated mathematical models and algorithms to achieve unprecedented efficiency. One such technique is perceptual coding, which aims to exploit the limitations of the human visual system. Since humans aren't equally sensitive to all visual details, perceptually-based codecs can selectively discard less noticeable information without significantly impacting perceived quality. This allows for higher compression ratios with minimal impact on visual fidelity.
Another significant advancement involves incorporating AI and machine learning. AI-powered codecs can learn complex patterns and redundancies within video data and tailor compression strategies accordingly. This can lead to significant improvements in both compression ratio and quality compared to traditional approaches. Deep learning models can be trained on vast datasets of video to optimize various aspects of compression, such as motion estimation, quantization, and rate control.
Case Study 1: The application of AI in HEVC encoding. Researchers have developed AI-based techniques to optimize rate control in HEVC encoding, improving the bitrate efficiency. Case Study 2: Perceptual coding applied to 360-degree video. Perceptual coding techniques prove particularly useful in compressing 360-degree videos, where the spatial redundancy is less pronounced compared to standard videos.
The integration of these technologies poses significant challenges. AI-powered codecs typically require extensive computational resources for both encoding and decoding, limiting their applicability on resource-constrained devices. Further research is essential to balance efficiency and computational complexity.
The Impact on Bandwidth and Streaming
Video compression's impact on bandwidth consumption is monumental. Without efficient compression, streaming high-definition video would be impossible for most users. The rise of streaming services like Netflix, YouTube, and others is heavily reliant on advancements in video compression. As video resolution and frame rates increase, the demand for efficient compression grows exponentially. Compression techniques allow streaming services to deliver high-quality video to a global audience while managing bandwidth costs and maintaining service quality.
Adaptive bitrate streaming (ABR) dynamically adjusts the video quality based on network conditions. This ensures smooth playback even with fluctuating network bandwidth. ABR leverages video compression by selecting the appropriate compressed version of the video based on available bandwidth. This approach allows for a seamless user experience, ensuring that viewers consistently get the best possible viewing experience regardless of their network connection.
Case Study 1: Netflix's use of ABR. Netflix is a prime example of a platform that has successfully leveraged ABR and sophisticated video compression techniques to deliver high-quality video across a wide range of network conditions. Case Study 2: YouTube's approach to video encoding and delivery. YouTube optimizes video encoding parameters based on the anticipated viewership and network conditions, balancing quality and bandwidth efficiency.
Future trends in bandwidth management will likely involve further advances in compression technologies, integration of edge computing, and the use of more sophisticated network management techniques. The challenge lies in keeping pace with the increasing demand for higher resolutions and more immersive video experiences while maintaining network efficiency.
The Future of Video Compression
The future of video compression is a dynamic landscape driven by several key factors. The relentless pursuit of higher resolutions (4K, 8K, and beyond), higher frame rates, and more immersive video experiences necessitates continuous innovation in compression techniques. The rise of virtual reality (VR) and augmented reality (AR) applications further increases the demands on compression technology. These applications require significantly higher data rates compared to traditional video, making efficient compression even more crucial.
The integration of artificial intelligence (AI) and machine learning is expected to play a prominent role. AI-powered codecs can adapt to varying content types and network conditions, optimizing compression dynamically. This dynamic adaptation will be crucial for managing the diverse and ever-evolving landscape of video content and network conditions.
Case Study 1: Exploration of neural network-based codecs. Researchers are actively investigating the use of neural networks to design more efficient and versatile codecs. Case Study 2: Development of codecs for high dynamic range (HDR) video. HDR video requires greater bit depths and wider color gamuts, posing new challenges for compression technologies.
The ongoing research and development in this field promise further advancements in compression efficiency and quality. The collaboration between researchers, industry players, and standardization bodies will be essential in shaping the future of video compression and its impact on the broader digital world.
In conclusion, the hidden mechanics of video compression are far from simple. It's a complex interplay of algorithms, standards, and evolving technologies. Understanding these mechanics is not just intellectually stimulating but also crucial for appreciating the technological marvel that enables our seamless consumption of vast amounts of visual data. As technology advances, so will the methods of compression, continuously pushing the boundaries of what's possible in the world of video.