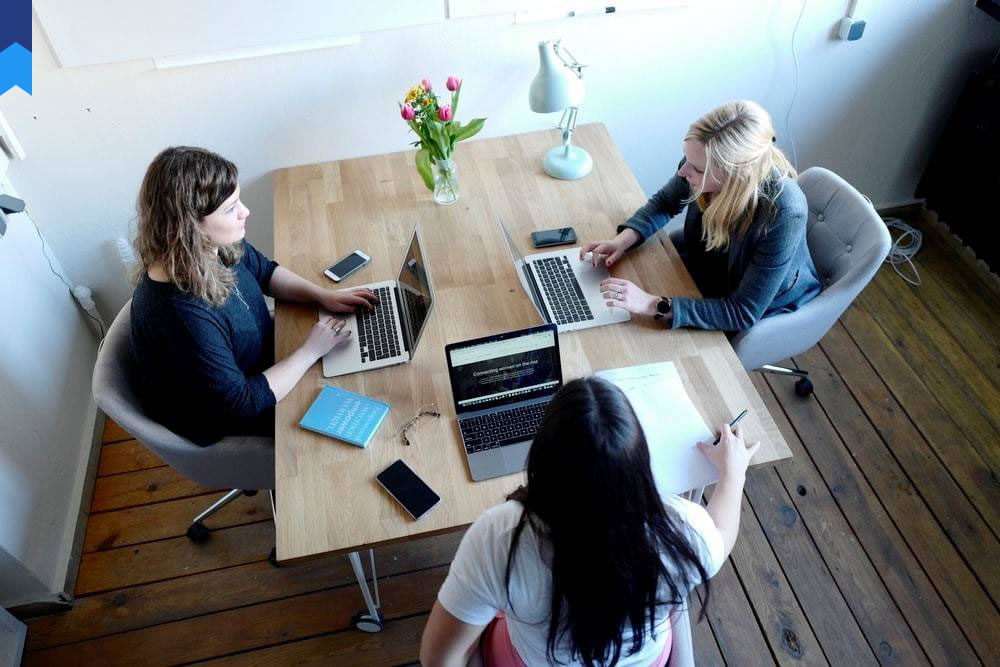
The Science Behind The AI Revolution
The rapid advancement of artificial intelligence (AI) is reshaping industries and daily life at an unprecedented pace. This exploration delves beyond the surface-level hype, examining the fundamental scientific principles fueling this technological revolution and its profound implications.
The Algorithmic Heart of AI
At the core of AI lies the intricate dance of algorithms. These are not mere lines of code; they are sophisticated mathematical formulas designed to mimic human cognitive functions like learning, problem-solving, and decision-making. Machine learning (ML), a subset of AI, empowers algorithms to learn from data without explicit programming. This learning occurs through statistical methods, analyzing vast datasets to identify patterns and make predictions. Deep learning (DL), a further advancement, uses artificial neural networks with multiple layers to process information hierarchically, enabling far greater complexity and accuracy. For example, image recognition systems, such as those used in self-driving cars, rely on deep learning to differentiate objects with remarkable precision. Case study: Google's AlphaGo, which defeated a world champion Go player, showcases the power of deep reinforcement learning, a sophisticated type of ML. Another case study focuses on IBM's Watson, a cognitive computing system capable of processing and analyzing natural language, demonstrating the capabilities of natural language processing (NLP). The sophistication of these algorithms hinges on the capacity for complex mathematical operations and the power of modern computing infrastructure. Improvements in both areas are continuously pushing the boundaries of what's possible.
Data: The Fuel of Intelligent Machines
AI systems are voracious consumers of data. The more data they process, the more accurate and effective they become. This data can range from structured information in databases to unstructured data like text, images, and videos. The quality, quantity, and diversity of data significantly impact the performance of AI models. Bias in data can lead to biased outputs, a critical concern in applications like facial recognition and loan applications. Data collection methods, data cleaning, and data preprocessing are crucial steps in the AI development pipeline. Case study: The success of recommendation systems on platforms like Netflix and Amazon relies heavily on the vast amounts of user data collected. Analyzing viewing habits and purchase histories allows these systems to predict preferences with remarkable accuracy. Another example is the use of data in healthcare, where AI is used to diagnose diseases based on medical images or patient records. The ethical implications of data collection and usage are also crucial to address, prompting concerns around data privacy and security. Data scientists are continuously exploring techniques to improve data quality, reduce bias, and ensure responsible data management. The development of synthetic data generation techniques is also allowing researchers to tackle situations where access to real-world data is limited or where privacy concerns need to be addressed.
The Hardware Revolution: Powering AI's Potential
The capabilities of AI are inextricably linked to advancements in hardware. Specialized processors like GPUs (Graphics Processing Units) and TPUs (Tensor Processing Units) are crucial for accelerating the computationally intensive tasks involved in training and running AI models. The development of more energy-efficient and powerful chips is essential for making AI more accessible and sustainable. Quantum computing holds the potential to revolutionize AI by offering exponential increases in processing power, enabling the solution of problems currently intractable for classical computers. Case study: NVIDIA's GPUs have become essential for deep learning applications, providing the computational muscle needed to train complex neural networks. Another crucial development is the advancement of specialized hardware like Intel's Loihi chip, designed for neuromorphic computing. This hardware design aims to create computers that mimic the function of the human brain, allowing for more energy-efficient processing of complex information. The evolution of hardware directly influences the scalability and efficiency of AI systems, constantly pushing forward the limits of their capabilities. The demand for specialized hardware is driving further innovation in chip design and manufacturing, leading to increased performance and reduced power consumption.
Ethical Considerations and Societal Impact
The rise of AI presents significant ethical challenges. Concerns around job displacement, algorithmic bias, and the potential misuse of AI technologies require careful consideration. The development and deployment of AI systems must adhere to ethical guidelines and regulations to ensure fairness, transparency, and accountability. The societal impact of AI is far-reaching, impacting everything from healthcare and education to transportation and security. Case study: The use of AI in hiring processes raises concerns about potential bias against certain demographic groups if the training data reflects existing societal biases. Another example focuses on the use of AI-powered surveillance systems and the potential for privacy violations. The need for responsible AI development, including strategies for mitigating bias and ensuring transparency, is of paramount importance. This includes promoting collaboration between researchers, policymakers, and the public to establish ethical frameworks and regulations that guide the development and deployment of AI technologies. Regular audits and independent evaluations of AI systems are also necessary to identify and address potential biases and risks.
The Future of AI: A Glimpse into Tomorrow
The future of AI is brimming with possibilities. Advancements in areas like explainable AI (XAI), which aims to make AI decision-making more transparent and understandable, are crucial for building trust and ensuring responsible use. The integration of AI with other emerging technologies, such as the Internet of Things (IoT) and blockchain, will lead to even more innovative applications. The development of more robust and resilient AI systems, capable of handling unexpected situations and adapting to changing environments, will be essential. Case study: XAI techniques are being developed to improve the transparency of medical diagnosis systems, providing doctors with insights into how the AI arrives at its conclusions. Another interesting area of research is the integration of AI with robotics to create more intelligent and adaptable robots. The development of more powerful and efficient AI algorithms, combined with advancements in hardware, will further accelerate the pace of innovation. This ongoing evolution necessitates a continuous reassessment of ethical guidelines and a proactive approach to addressing the societal implications of these advancements.
In conclusion, the AI revolution is not merely a technological advancement; it's a fundamental shift in how we interact with the world. Understanding the science behind AI, from its algorithmic foundations to its ethical considerations, is crucial for harnessing its potential while mitigating its risks. The future of AI will be shaped by our collective choices, requiring a concerted effort to ensure its responsible development and deployment for the benefit of humanity.