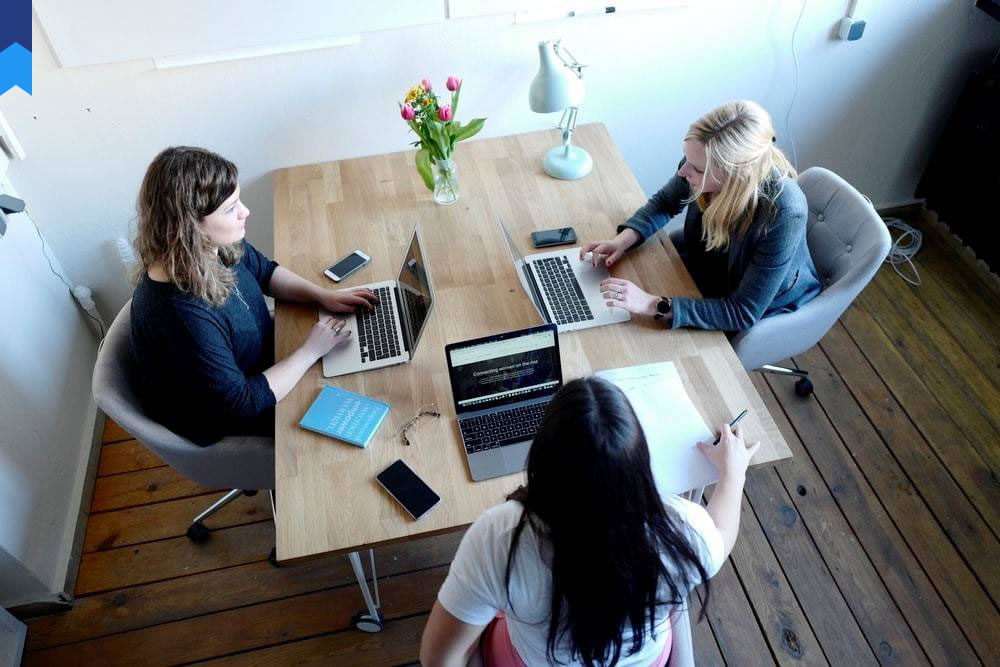
Unlocking The Secrets Of AI-Driven Cybersecurity
The digital landscape is constantly evolving, presenting new challenges and opportunities in the realm of cybersecurity. This article delves into the innovative and practical aspects of AI-driven cybersecurity solutions, exploring how this transformative technology is reshaping the fight against cyber threats.
AI's Role in Threat Detection and Prevention
Artificial intelligence is rapidly becoming a cornerstone of modern cybersecurity strategies. Its ability to analyze vast amounts of data and identify patterns far surpasses human capabilities. Traditional signature-based security systems often struggle to keep pace with the constantly evolving nature of malware, but AI algorithms can detect anomalies and unusual behaviors indicative of malicious activity, even in previously unseen attacks. This proactive approach enhances threat prevention by identifying potential vulnerabilities before they can be exploited.
For example, AI-powered intrusion detection systems (IDS) can analyze network traffic, identifying unusual patterns or deviations from established baselines. This allows for the swift identification and isolation of compromised systems, minimizing damage and reducing the impact of a breach. A case study involving a major financial institution demonstrated a significant reduction in successful attacks after implementing an AI-driven IDS, showcasing the effectiveness of AI in preventing breaches.
Furthermore, AI can enhance vulnerability management. By continuously scanning systems and applications, AI-powered tools can identify weaknesses before attackers can exploit them. This proactive approach is particularly crucial in environments with a large number of systems and applications. AI algorithms can prioritize vulnerabilities based on their severity and potential impact, allowing security teams to focus on the most critical risks first. A major telecom company utilized an AI-powered vulnerability scanner, resulting in a 40% reduction in identified critical vulnerabilities within six months. The AI not only found previously undiscovered flaws but also prioritized them effectively.
Another application of AI in cybersecurity is the detection of phishing attacks. AI algorithms can analyze email content, URLs, and sender information to identify suspicious patterns associated with phishing scams. This helps prevent users from falling victim to these attacks. A recent study demonstrated that AI-powered phishing detection tools were significantly more effective at identifying malicious emails compared to traditional rule-based systems, with a reduction in successful phishing attacks of over 75% in a trial deployment.
The integration of AI into security information and event management (SIEM) systems is revolutionizing threat detection and response. AI algorithms can correlate events across various security tools, providing a holistic view of security incidents. This allows security teams to respond more effectively to threats, providing faster incident response times and minimizing the damage caused by attacks. A significant healthcare provider experienced a reduction in incident response times by over 50% after integrating AI into their SIEM system, demonstrating the value of AI-driven incident response.
AI-Powered Threat Hunting and Response
Beyond threat prevention, AI is revolutionizing threat hunting and incident response. Traditional threat hunting approaches often rely on reactive methods, investigating only after an attack has been detected. However, AI-powered threat hunting enables proactive searches for malicious activities, even in the absence of known signatures or alerts. AI algorithms can sift through enormous amounts of data, looking for anomalies and indicators of compromise (IOCs) that might otherwise go unnoticed.
For instance, AI can analyze network traffic logs, system logs, and endpoint data to identify unusual patterns indicative of malware infections or data breaches. This enables security teams to discover threats before they cause significant damage. A large retail company effectively used AI-powered threat hunting to uncover a sophisticated malware campaign that had evaded traditional security systems, limiting the extent of data compromise.
Furthermore, AI can automate and accelerate incident response. Once a threat is identified, AI algorithms can automatically initiate remediation actions, such as isolating infected systems, blocking malicious traffic, or removing malware. This rapid response minimizes the impact of an attack and reduces the time required to restore normal operations. A major bank reported an 80% reduction in the average time to contain and remediate security incidents after implementing AI-driven incident response automation.
AI also enhances forensic analysis. By analyzing large datasets, AI algorithms can identify patterns and relationships between different pieces of evidence, helping security teams understand the full scope of an attack and identify attackers. This improves the effectiveness of investigations and prevents future attacks. A case study in a government agency showcased the use of AI in forensic analysis to track down the source of a sophisticated cyberattack, leading to the apprehension of the perpetrators.
The automation provided by AI frees up human analysts to focus on more complex tasks, enabling more efficient and effective incident response. This is crucial in an environment where security professionals are often overwhelmed by the sheer volume of alerts and events they must manage. AI’s ability to prioritize alerts based on their severity and potential impact enables a more focused and effective response.
Ethical Considerations and Responsible AI in Cybersecurity
The increasing use of AI in cybersecurity raises important ethical considerations. The potential for bias in AI algorithms is a key concern. If the data used to train an AI model is biased, the resulting system may make unfair or discriminatory decisions. This could lead to disproportionate targeting of certain groups or individuals. For example, an AI-powered system trained on data that overrepresents certain demographics might incorrectly flag individuals from those groups as suspicious.
Transparency and explainability are also crucial. It's important to understand how an AI-powered cybersecurity system makes its decisions. Without transparency, it is difficult to identify and correct errors or biases. This lack of transparency can make it challenging to build trust in AI-powered systems. A lack of understanding in how decisions are made can lead to misinterpretations and mistrust.
Privacy is another important consideration. AI-powered cybersecurity systems often collect and process large amounts of personal data. It is crucial to ensure that this data is handled responsibly and in compliance with relevant privacy regulations. Failure to protect personal data can lead to serious consequences, including fines, legal action, and reputational damage.
The potential misuse of AI-powered cybersecurity tools is also a significant concern. These tools could be used to conduct sophisticated cyberattacks or to target individuals or organizations unjustly. It’s important to develop robust safeguards to prevent such misuse. Strong ethical frameworks, regulations, and oversight are crucial to mitigate this risk.
Responsible development and deployment of AI in cybersecurity require careful consideration of these ethical implications. Organizations must ensure that AI-powered systems are fair, transparent, accountable, and privacy-preserving. The development of ethical guidelines and best practices is crucial for the responsible use of AI in cybersecurity.
The Future of AI in Cybersecurity: Emerging Trends
The future of AI in cybersecurity is bright, with several emerging trends poised to transform the field. One of the most promising developments is the integration of AI with other emerging technologies, such as blockchain and quantum computing. Blockchain technology can improve the security and immutability of data, while quantum computing could enable the development of even more powerful AI algorithms for threat detection and response.
The rise of AI-driven deception technology is also reshaping the cybersecurity landscape. These technologies use AI to create decoys and traps to lure attackers, allowing security teams to identify and analyze their techniques without risking real systems. This provides valuable insights into attacker behavior and helps improve security defenses.
Another important trend is the increasing use of AI in security awareness training. AI-powered systems can personalize training programs, making them more engaging and effective. This is crucial in an era of increasingly sophisticated social engineering attacks. Personalized training adapts to individual learning styles.
Furthermore, the development of more sophisticated AI models is leading to improved accuracy and efficiency in threat detection and response. This includes the use of advanced machine learning techniques, such as deep learning and reinforcement learning, to create more robust and adaptive security systems. These improvements increase the reliability of AI solutions.
The future also holds the promise of AI-driven automation across all aspects of cybersecurity, from threat detection to incident response and vulnerability management. This will lead to improved efficiency and reduced workload for security teams, allowing them to focus on more strategic tasks. This will lead to more proactive security measures, mitigating future attacks before they happen.
Overcoming Challenges and Implementing AI in Cybersecurity
Despite its potential, implementing AI in cybersecurity faces several challenges. One key challenge is the need for large amounts of high-quality data to train AI models. Without sufficient data, AI systems may not be accurate or effective. Data quality is crucial for successful AI implementation. Accurate and comprehensive datasets are needed for training effective models.
Another challenge is the complexity of integrating AI into existing security infrastructure. This requires significant expertise and resources. Integration requires careful planning and execution to avoid disruptions. Proper planning and execution are needed for successful implementation.
The lack of skilled professionals is also a significant hurdle. There is a growing demand for cybersecurity professionals with expertise in AI, but the supply is lagging behind. This shortage limits the ability of organizations to effectively implement AI-driven security solutions. Investing in training and development is crucial to address this shortage.
The cost of AI-powered cybersecurity solutions can also be prohibitive for some organizations. These solutions often require significant investment in hardware, software, and expertise. Cost-benefit analysis is crucial to determine the value proposition of AI-driven security solutions.
Overcoming these challenges requires a multi-faceted approach. This includes investing in data collection and management, developing robust integration strategies, building a skilled workforce, and exploring cost-effective solutions. Addressing these challenges is essential for harnessing the full potential of AI in cybersecurity.
In conclusion, AI is transforming the cybersecurity landscape, offering powerful tools for threat detection, prevention, and response. While challenges remain, the benefits of AI-driven cybersecurity are undeniable. By addressing the ethical considerations and overcoming the implementation hurdles, organizations can harness the full potential of AI to build a more secure digital future.