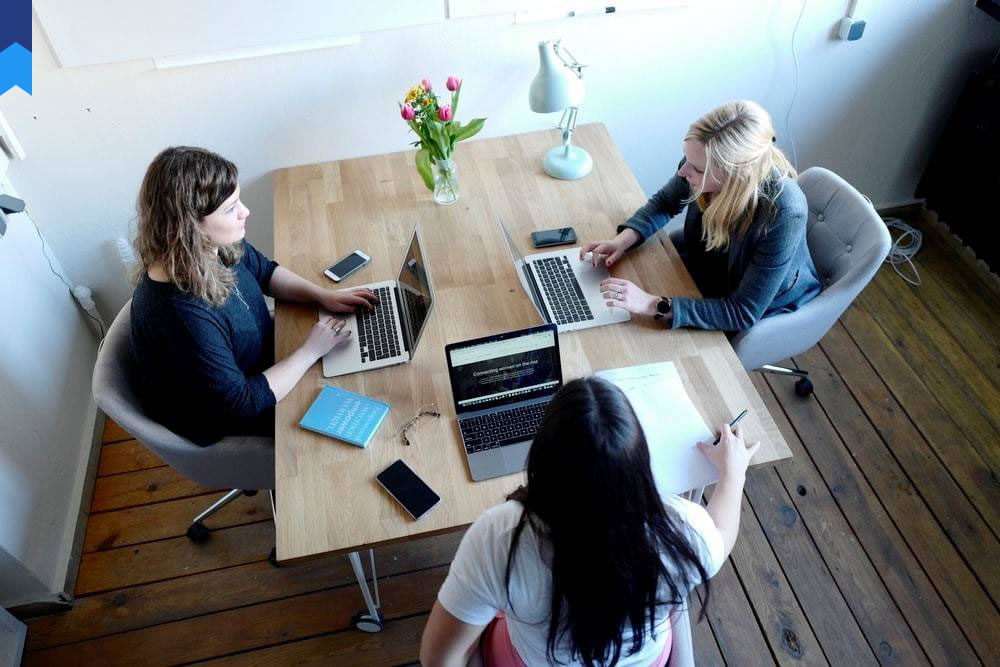
What Tech Experts Don't Tell You About AI Integration
What Tech Experts Don't Tell You About AI Integration
Introduction
The rapid integration of artificial intelligence (AI) across industries is reshaping the technological landscape at an unprecedented pace. While the potential benefits are widely touted – increased efficiency, improved decision-making, and innovative product development – a critical gap exists in the narrative. Experts often gloss over the complex challenges, unforeseen consequences, and hidden costs associated with successful AI implementation. This article delves into those often-overlooked aspects, providing a more realistic and nuanced perspective on the realities of AI integration.
The Data Deluge: Challenges in Data Acquisition and Preparation
AI models thrive on data; the more data, the better the model's performance. However, acquiring sufficient high-quality data is a significant hurdle. Data may be scattered across various sources, formats, and systems, requiring substantial cleaning, transformation, and integration efforts. This process, often underestimated, can consume significant time and resources. Consider the case of a healthcare provider attempting to integrate AI for diagnostics. Gathering patient data from diverse sources, ensuring data privacy compliance (HIPAA, GDPR), and standardizing disparate formats are monumental tasks. Furthermore, data bias, a critical issue, can lead to inaccurate or unfair outcomes if not meticulously addressed. For instance, a facial recognition system trained primarily on images of one ethnicity may perform poorly on others, leading to biased results.
Another challenge lies in data security. Protecting sensitive data used to train AI models is paramount. Breaches can lead to significant financial and reputational damage. Companies investing in AI must invest equally in robust cybersecurity measures. Consider the example of a financial institution employing AI for fraud detection. A data breach exposing customer transaction details could have catastrophic consequences, impacting both the institution and its clients. The preparation and integration of data, including addressing bias and security concerns, can often represent 70% to 80% of the total AI implementation project time and cost, a fact often overlooked in initial projections.
The cost of data acquisition and preparation can be exorbitant, especially for specialized datasets. This is particularly true for industries such as medicine, where obtaining and annotating medical images for AI training may require the expertise of medical professionals, driving up costs. Furthermore, the ongoing maintenance and updates of data are crucial for ensuring that AI models remain accurate and relevant over time. This continuous data refresh is an often-overlooked operational expense. A recent study showed that 40% of AI projects fail due to inadequate data management.
Best practices in this realm involve establishing clear data governance policies, implementing robust data quality checks, and investing in tools and techniques for data cleaning, transformation, and integration. Organizations should also adopt a proactive approach to data security, implementing encryption and access controls to minimize risks.
The Skills Gap: Finding and Retaining AI Expertise
Successfully implementing AI requires a skilled workforce capable of designing, building, deploying, and maintaining AI systems. There's a significant global shortage of AI professionals with the necessary expertise in machine learning, deep learning, data science, and related fields. This skills gap makes it challenging to find and retain qualified personnel, driving up salaries and increasing competition for talent. For example, a large technology company might find themselves competing with numerous other companies for the same limited pool of experienced AI engineers and data scientists.
The complexity of AI systems also demands a multidisciplinary team. Besides technical expertise, organizations need professionals with business acumen, domain knowledge, and ethical considerations for responsible AI implementation. Finding individuals who possess a blend of technical, business, and ethical understanding is even more challenging. A healthcare organization integrating AI for patient care, for example, needs a team encompassing not only data scientists and AI engineers but also clinicians, ethicists, and legal experts to address regulatory and ethical concerns. This interdisciplinary requirement significantly expands the complexity of talent acquisition and management.
To address this, companies are investing heavily in AI training programs and upskilling existing employees. However, the sheer scale of the skills gap necessitates a more holistic approach. Educational institutions are responding by expanding AI-related curricula, while governments are implementing policies to support AI education and workforce development. Yet, the demand continues to outpace supply, creating an ongoing challenge for organizations looking to leverage AI effectively. A recent survey indicated that 85% of organizations reported difficulty finding skilled AI professionals.
Effective strategies for navigating this challenge include targeted recruitment strategies, competitive compensation packages, comprehensive training programs, and fostering a culture that attracts and retains top talent. Building internal expertise through training programs and apprenticeships is a crucial long-term solution.
Ethical Considerations and Responsible AI
The ethical implications of AI are increasingly becoming central to discussions surrounding its integration. Bias in algorithms, data privacy concerns, job displacement, and the potential for misuse are all significant considerations that need to be addressed proactively. For instance, an AI system used for loan applications could perpetuate existing biases if the training data reflects historical societal inequalities, potentially leading to unfair lending practices. Addressing bias requires careful curation of training data and rigorous testing of AI models for fairness and equity. Similarly, using AI for facial recognition raises concerns about privacy and potential for surveillance, necessitating strict regulations and transparent deployment strategies.
Transparency in AI development and deployment is crucial to building trust. Organizations must be clear about how their AI systems work, what data they use, and how decisions are made. This transparency fosters accountability and allows for scrutiny of potential biases or unintended consequences. Consider a case where an AI system is used in a hiring process; transparency allows for auditing and ensures that the system is not unfairly discriminating against certain groups. Without transparency, such issues might go unnoticed.
Establishing ethical guidelines and frameworks for AI development and deployment is paramount. Organizations must adopt ethical principles throughout the AI lifecycle, from data collection to model deployment and monitoring. This includes regular audits and reviews to ensure that AI systems align with ethical standards. The lack of clear guidelines and enforcement mechanisms often leaves companies operating in a regulatory grey area, leading to potential legal and reputational risks. Best practices in this field include establishing ethical review boards, implementing bias detection tools, and fostering a culture of responsible AI within the organization.
The development of robust regulatory frameworks is crucial. Governments are actively working on establishing guidelines and regulations to ensure responsible AI development and deployment. These efforts include addressing data privacy, algorithmic accountability, and the potential for job displacement. However, the rapid pace of AI innovation poses a continuous challenge for regulators.
Integration Challenges: Legacy Systems and Organizational Change
Integrating AI into existing organizational structures and legacy systems presents significant challenges. Many companies operate with outdated infrastructure and processes that are not easily compatible with modern AI technologies. This incompatibility can create integration bottlenecks, slow down deployment, and increase costs. For example, a manufacturing company attempting to integrate AI-powered predictive maintenance might find its existing sensor network inadequate, requiring significant investment in upgrading the infrastructure.
Organizations need to assess their existing infrastructure and processes to determine their readiness for AI integration. This requires a thorough evaluation of data systems, IT infrastructure, and business workflows. A detailed roadmap outlining the necessary changes and upgrades is essential for successful implementation. The lack of a clear roadmap can lead to significant delays and cost overruns. Consider the case of a retail company implementing AI-powered recommendation systems. Without properly planning the integration with its existing e-commerce platform, the company might face delays in launch and difficulties in managing customer data.
Organizational change management is critical for successful AI integration. Employees need to be adequately trained and supported throughout the transition. Resistance to change is a common barrier, and addressing this requires a well-defined communication strategy and clear articulation of the benefits of AI implementation. A lack of employee buy-in can significantly hinder the success of AI initiatives. A survey revealed that 60% of failed AI projects were attributed to insufficient change management efforts. Successful AI integration requires not just technical expertise but also careful consideration of the human element.
Best practices include developing a detailed integration plan, investing in training programs for employees, and establishing clear communication channels to address concerns and promote collaboration. Organizations should also foster a culture of experimentation and continuous improvement to facilitate successful AI adaptation.
Conclusion
While the promise of AI is undeniable, the path to successful integration is fraught with challenges that extend far beyond simple technological hurdles. Overlooking the complexities of data management, the skills gap, ethical considerations, and the necessary organizational changes can lead to costly delays, disappointing results, and even reputational damage. A holistic and nuanced understanding of these challenges, coupled with proactive planning and a commitment to responsible AI development, is crucial for reaping the true benefits of this transformative technology. The future of successful AI implementation lies in addressing these often-overlooked aspects, fostering collaboration between technical experts, business leaders, and ethicists, and prioritizing a strategic approach that balances technological advancements with organizational realities and ethical considerations.