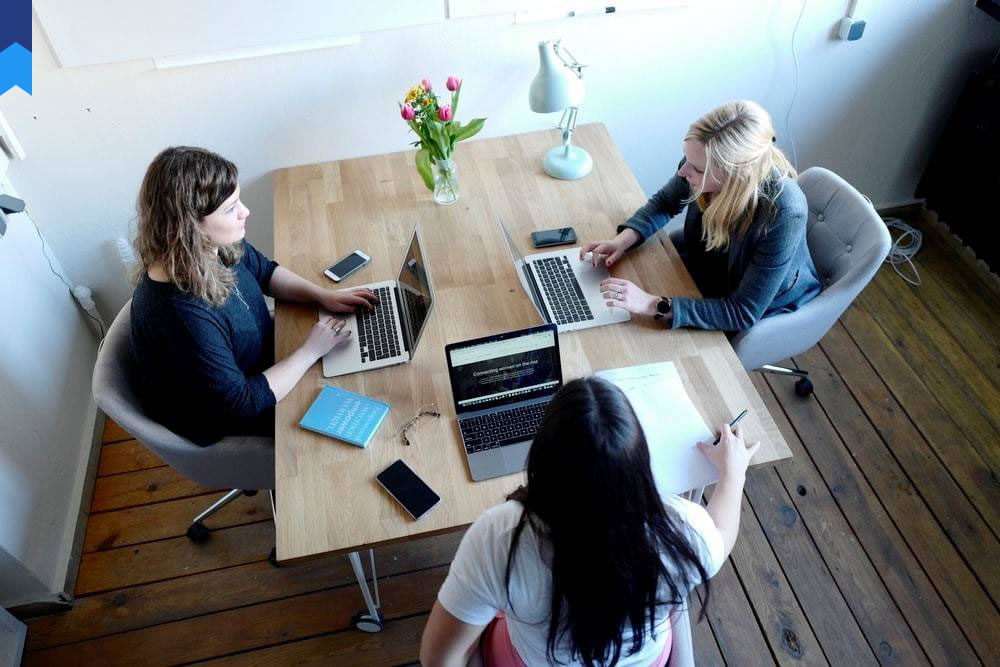
What Tech Experts Don't Tell You About AI's Real-World Impact
The rapid advancement of artificial intelligence (AI) has captured the world's imagination, promising a future brimming with technological marvels. However, the narrative often focuses on futuristic scenarios, overlooking the immediate, tangible impacts AI is having on our lives. This article delves into the often-overlooked aspects of AI's real-world influence, exploring its subtle yet profound effects on various sectors.
The Unseen Bias in AI Algorithms
AI systems, particularly those trained on large datasets, often inherit and amplify existing societal biases. These biases, embedded in the data used for training, manifest as discriminatory outcomes in areas like loan applications, hiring processes, and even criminal justice. For instance, facial recognition technology has demonstrated a higher error rate in identifying individuals with darker skin tones, highlighting the critical need for algorithmic fairness and accountability. A study by the National Institute of Standards and Technology (NIST) found significant disparities in accuracy across different demographic groups, underscoring the pervasive nature of this issue. Addressing this requires careful data curation, algorithmic auditing, and a greater focus on diversity and inclusion in the development process. A compelling case study is the use of AI in healthcare, where biased algorithms could lead to misdiagnosis or unequal access to treatment. Another example lies in the recruitment industry, where AI-powered systems may inadvertently favor candidates from specific backgrounds, perpetuating existing inequalities. The development of bias mitigation techniques, such as adversarial debiasing and fairness-aware learning, represents crucial steps toward mitigating these biases.
Furthermore, the lack of transparency in many AI algorithms, often referred to as the "black box" problem, makes it difficult to identify and address these biases effectively. Explainable AI (XAI) is emerging as a solution, aiming to create more interpretable models that reveal the reasoning behind their decisions. However, the transition to XAI requires significant advancements in both theoretical understanding and practical implementation. The implementation of robust regulatory frameworks, similar to those governing data privacy, will be crucial in ensuring responsible AI development and deployment. This requires collaboration between researchers, policymakers, and industry leaders to establish guidelines and best practices for fair and ethical AI development. Continuous monitoring and evaluation of AI systems in real-world applications are necessary to identify and mitigate potential biases over time. The challenge lies in balancing the drive for innovation with the need for ethical and responsible use of this powerful technology.
The increasing reliance on AI in decision-making processes necessitates a deeper understanding of the potential for bias amplification. This requires a multi-faceted approach involving diverse teams, rigorous testing, and ongoing monitoring of AI systems. Failure to address these biases could lead to significant social and economic inequalities, further exacerbating existing societal divisions. The development and implementation of bias detection and mitigation techniques represent a crucial step towards ensuring fairness and accountability in the age of AI. This is not merely a technical challenge, but also a social and ethical imperative.
Case studies involving biased AI systems in hiring practices demonstrate the real-world consequences of neglecting fairness considerations. Companies are increasingly using AI-powered tools to screen resumes and shortlist candidates, yet these systems may inadvertently discriminate against certain demographic groups if the training data is not carefully vetted. Similarly, loan application processes powered by AI algorithms may disproportionately reject applications from specific communities due to biases in the underlying data. Addressing these biases requires a comprehensive strategy that involves data auditing, algorithmic transparency, and the development of fairness-aware AI systems. The legal and ethical implications of biased AI systems are also significant, necessitating the development of robust regulatory frameworks and ethical guidelines to guide the responsible deployment of this technology.
The Job Displacement Myth (and the Reality)
While fears of widespread job displacement due to AI are prevalent, the reality is more nuanced. AI is not simply replacing jobs; it's transforming them. Many roles will be augmented by AI, requiring workers to adapt and acquire new skills. For instance, while some manufacturing jobs may be automated, there's a growing demand for AI specialists to maintain and improve these systems. The World Economic Forum estimates that while some jobs will be lost, many more will be created, necessitating a shift in workforce skills and education. The focus should be on reskilling and upskilling the workforce to meet the evolving demands of the AI-driven economy. This requires significant investment in education and training programs, as well as collaboration between industry and educational institutions to develop relevant curricula. However, the transition won't be seamless; some workers may face temporary unemployment or require significant retraining. The challenge lies in managing this transition effectively, providing support for displaced workers, and fostering a culture of lifelong learning.
The impact of AI on various sectors, such as healthcare, finance, and transportation, varies considerably. In healthcare, AI is assisting doctors with diagnosis and treatment, leading to improved efficiency and accuracy. However, this also requires healthcare professionals to adapt to new technologies and workflows. In finance, AI is being used for fraud detection and risk management, while in transportation, self-driving cars are slowly transforming the industry. These advancements create new job opportunities in AI development, data science, and AI-related services, but also require retraining and upskilling for existing workers. Moreover, the ethical considerations of using AI in these sectors must be addressed, including data privacy, algorithmic bias, and accountability.
A case study of a manufacturing company that implemented robotic process automation (RPA) showcases both the opportunities and challenges of AI-driven job transformation. While some repetitive tasks were automated, leading to increased efficiency, the company also invested heavily in retraining its workforce to manage and maintain the new robotic systems. This involved partnerships with local educational institutions and customized training programs. Another example is the adoption of AI-powered chatbots in customer service, which automated routine inquiries, freeing up human agents to handle more complex issues. This required a shift in the role of customer service representatives, focusing on more sophisticated problem-solving and relationship management. The successful integration of AI in the workplace requires careful planning, proactive workforce development, and a clear understanding of the evolving nature of work.
The future of work in an AI-driven world will require a proactive and adaptive approach. Government policies, industry initiatives, and individual efforts are all crucial in navigating this transition effectively. Investing in education, fostering collaboration between industry and education, and providing support for displaced workers will be essential in ensuring a smooth and equitable transition to the AI-driven economy. The focus should be on creating a workforce that is adaptable, resilient, and equipped with the skills needed to thrive in this evolving landscape.
The Data Security Dilemma
The increasing reliance on AI systems necessitates the collection and processing of vast amounts of data. This raises critical concerns about data security and privacy. Data breaches involving sensitive personal information can have devastating consequences, both for individuals and organizations. Robust security measures are crucial to protect this valuable data from unauthorized access and misuse. The implementation of strong encryption techniques, access control mechanisms, and regular security audits is essential. However, the ever-evolving nature of cyber threats requires a proactive and adaptable security strategy. Staying ahead of emerging threats necessitates continuous investment in security infrastructure and personnel training. The development of AI-powered security tools can help in detecting and responding to cyber threats more effectively, but these tools themselves need to be secure and resistant to attacks.
The use of AI in data security raises several complex issues, including the potential for AI-powered attacks. Adversaries could use AI to create sophisticated phishing scams or bypass existing security systems. This calls for the development of countermeasures that leverage AI to detect and defend against these advanced threats. Moreover, the ethical implications of using AI for surveillance and monitoring must be carefully considered, balancing the need for security with the protection of individual privacy. Regulatory frameworks are essential to guide the responsible use of AI in data security, ensuring accountability and transparency. A balanced approach is needed that prioritizes security without compromising individual rights and freedoms.
A recent case study highlights the vulnerability of AI systems to adversarial attacks. Researchers demonstrated how small, imperceptible changes to input data could cause AI-powered image recognition systems to misclassify objects, demonstrating the potential for malicious actors to exploit vulnerabilities in AI systems. Another case study explores the challenges of securing sensitive medical data used to train AI algorithms in healthcare. The need to protect patient privacy while leveraging the power of AI for improved diagnostics and treatment requires stringent security protocols and robust data anonymization techniques. Addressing these challenges requires a multi-layered approach involving technical solutions, legal frameworks, and ethical considerations.
The future of data security in an AI-driven world necessitates a proactive and holistic approach. Collaboration between researchers, industry leaders, and policymakers is crucial in developing effective security measures, ethical guidelines, and regulatory frameworks. This includes continuous investment in cybersecurity research, development of advanced security technologies, and education and training of cybersecurity professionals. Addressing the challenges of data security is not merely a technical imperative, but also a social and ethical responsibility.
The Ethical Quandaries of Autonomous Systems
The development of autonomous systems, such as self-driving cars and robots, raises a plethora of ethical dilemmas. These systems are increasingly making decisions that have real-world consequences, raising questions about accountability, responsibility, and the potential for unintended harm. Defining clear ethical guidelines and frameworks is crucial to ensure responsible development and deployment of these technologies. This requires careful consideration of various scenarios and the potential for unintended consequences. For instance, in the case of a self-driving car accident, determining liability and responsibility can be complex and legally challenging.
The ethical considerations surrounding autonomous weapons systems are particularly pressing. The potential for unintended escalation and the lack of human control raise serious concerns about the use of lethal autonomous weapons. International cooperation and clear ethical guidelines are essential to prevent the proliferation of such weapons and ensure responsible development of autonomous technologies. Ethical frameworks should guide the design and deployment of these systems, emphasizing human oversight and accountability. The development of explainable AI (XAI) can enhance transparency and help in understanding the decision-making processes of autonomous systems, but this is still an active area of research.
A case study on the ethical challenges faced by developers of self-driving cars highlights the complexities of programming autonomous systems to make ethical decisions. Scenarios involving unavoidable accidents, where the system must choose between different courses of action, raise difficult questions about prioritizing human safety. Another case study explores the ethical considerations of using AI in healthcare, where decisions about resource allocation and patient treatment could have life-or-death consequences. Developing ethical guidelines for autonomous systems requires a multidisciplinary approach, involving experts in AI, ethics, law, and other relevant fields. This requires open dialogue, careful consideration of diverse perspectives, and the development of robust regulatory frameworks.
The future of autonomous systems will depend on the ability to address these ethical challenges effectively. Developing clear ethical guidelines, fostering international cooperation, and investing in research on ethical AI are essential steps towards ensuring responsible development and deployment of these powerful technologies. Failure to address these ethical concerns could lead to unintended consequences and undermine public trust in autonomous systems.
The Need for Transparency and Accountability
Transparency and accountability are paramount in the development and deployment of AI systems. The lack of transparency in many AI algorithms hinders understanding and trust. Explainable AI (XAI) is crucial to address this issue, making the decision-making processes of AI systems more understandable and interpretable. This allows for better scrutiny and identification of potential biases or errors. Moreover, clear lines of accountability are needed to determine responsibility in case of errors or harm caused by AI systems. This requires a collaborative effort between developers, users, and regulatory bodies to establish clear guidelines and mechanisms for accountability.
The development of robust auditing mechanisms is crucial for ensuring transparency and accountability in AI systems. Regular audits can identify potential biases, security vulnerabilities, and ethical concerns. These audits should be conducted by independent experts with relevant expertise and experience. The results of these audits should be publicly available to foster transparency and build trust. Furthermore, mechanisms for redress and compensation should be in place to address harm caused by AI systems. This requires clear legal frameworks and processes for resolving disputes and ensuring fair outcomes.
A case study of an AI-powered loan application system highlights the importance of transparency and accountability. The lack of transparency in the system's decision-making process led to discrimination against certain groups, underscoring the need for greater transparency and explainability. Another case study examines the challenges of ensuring accountability in the development and deployment of autonomous weapons systems, emphasizing the need for international cooperation and clear ethical guidelines. Addressing these challenges requires a multi-faceted approach involving technical solutions, legal frameworks, and ethical considerations.
The future of responsible AI development will depend on the ability to address these challenges effectively. Promoting transparency and accountability requires a collaborative effort between researchers, developers, users, and policymakers. Investing in research on XAI, developing robust auditing mechanisms, and establishing clear lines of accountability are essential steps towards building trust and ensuring responsible use of AI.
Conclusion
The real-world impact of AI extends far beyond the headlines, subtly reshaping industries, jobs, and societal norms. While the potential benefits are immense, addressing the challenges of bias, job displacement, data security, and ethical dilemmas is paramount. The path forward demands a multi-pronged approach: prioritizing transparency and accountability in AI development, fostering a culture of lifelong learning and reskilling, establishing robust data security protocols, and proactively engaging in ethical considerations. By acknowledging and addressing these complex issues, we can harness the transformative power of AI while mitigating its potential risks and ensuring a future where technology serves humanity ethically and equitably.