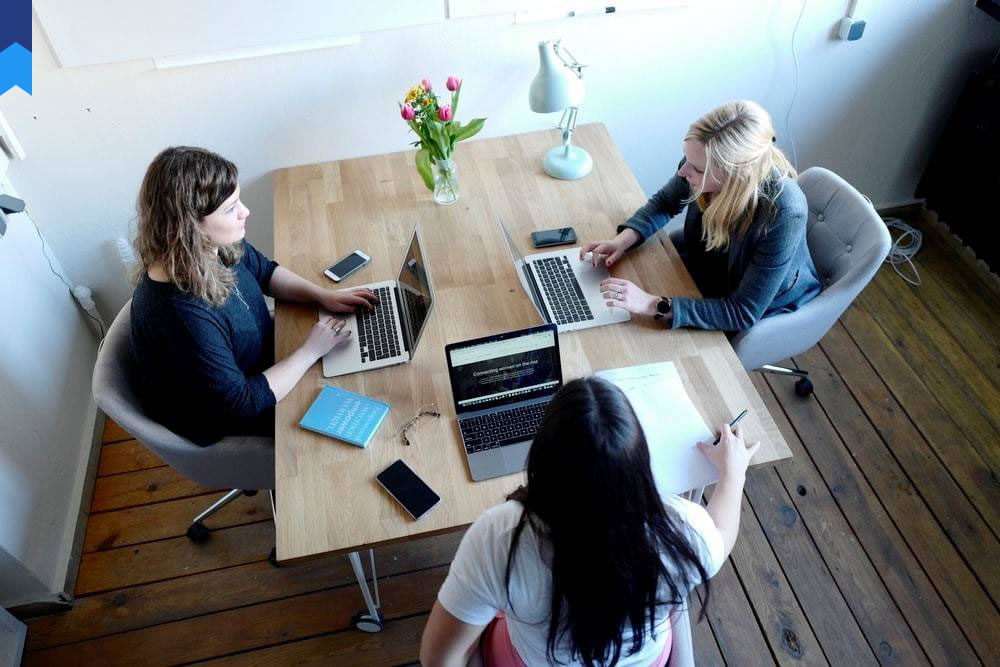
What Tech Gurus Don't Tell You About AI Integration
The rapid integration of artificial intelligence (AI) into various sectors is transforming industries and redefining how we interact with technology. However, the hype often overshadows the complexities, challenges, and unforeseen consequences of this transformative technology. This article delves into the unspoken truths behind AI integration, exploring practical aspects often omitted in mainstream discussions.
The Hidden Costs of AI Implementation
Deploying AI systems involves more than just acquiring the latest software. Significant upfront investment in infrastructure, data acquisition, and skilled personnel is often underestimated. Consider the need for powerful servers capable of processing vast amounts of data, specialized data scientists to clean, process, and analyze information, and ongoing maintenance costs. For instance, a small business might underestimate the cost of training data for a custom AI model, leading to unexpected budget overruns. Case study: Company X attempted to integrate an AI-powered customer service chatbot without considering the expense of data annotation, resulting in a flawed system with limited accuracy. Another example: Company Y failed to account for the ongoing maintenance and updates needed for their AI infrastructure, leading to significant additional expenses later in their implementation.
Beyond financial costs, the integration process itself can be disruptive. Existing workflows must be adapted, employees might require retraining, and potential job displacement needs careful consideration. Organizations need to carefully plan for change management, addressing employee concerns and proactively managing the transition. For example, the introduction of automated systems in manufacturing might lead to job losses if not properly handled. Conversely, a well-planned implementation could lead to increased productivity and job creation in other areas. A company that successfully navigated this transition is Company Z, which upskilled its workforce by providing comprehensive training programs, thereby mitigating job losses and leveraging the benefits of AI integration. The company carefully planned and executed a gradual implementation strategy, minimizing disruptions to existing workflows and ensuring a smooth transition.
Furthermore, the ongoing cost of data management and upkeep must be factored in. AI models require constant feeding of fresh, relevant data to maintain accuracy and avoid becoming obsolete. This is particularly crucial in rapidly evolving industries such as finance and healthcare, where continuous updates are critical to ensure optimal performance. Consider the case of a financial institution using AI for fraud detection. The AI model must be regularly updated with new transaction data to effectively identify emerging fraud patterns. Another company, Company W, successfully integrated AI into its healthcare system by creating a robust data pipeline that continuously fed the AI system with updated information on patients, treatments, and medical research.
In conclusion, the real cost of AI integration extends far beyond the initial investment. Companies must thoroughly assess and plan for the hidden financial, operational, and human resource costs to ensure successful AI implementation and avoid costly setbacks. Proper planning and resource allocation are crucial in this process. The long-term sustainability of any AI project hinges on this vital understanding. Ignoring these aspects can lead to failure, illustrating that initial price tags don't tell the whole story.
Ethical Considerations and Bias in AI Systems
The ethical implications of AI integration are often overlooked. AI systems are trained on data, and if that data reflects existing societal biases, the AI system will perpetuate and amplify those biases. This can lead to unfair or discriminatory outcomes, particularly in areas like loan applications, hiring processes, and even criminal justice. For instance, facial recognition systems have been shown to be less accurate in identifying individuals with darker skin tones, leading to potential misidentification and wrongful arrests. Case study: A study revealed a bias in an AI-powered hiring tool that favored male candidates over equally qualified female candidates, highlighting the importance of rigorous testing for bias. Another case study examined the use of AI in criminal justice and found evidence of racial bias in risk assessment tools.
Furthermore, the lack of transparency in some AI algorithms makes it difficult to understand how decisions are made. This "black box" nature can make it challenging to identify and correct biases, leading to distrust and a lack of accountability. This is particularly problematic in high-stakes applications where transparency and explainability are essential. For example, an AI system used for medical diagnosis should be transparent enough to allow clinicians to understand how the system arrived at its conclusions. The lack of transparency can impede trust and hinder the adoption of AI systems in critical sectors. Consider the example of an AI-powered loan application system that rejects a loan application without providing any explanation for the decision. This lack of transparency can lead to dissatisfaction and distrust among applicants.
Data privacy is another crucial ethical consideration. AI systems often require access to large amounts of personal data, raising concerns about data security and potential misuse. Robust data protection measures are crucial to ensure compliance with privacy regulations and protect individuals' rights. The General Data Protection Regulation (GDPR) is an example of a regulatory framework aimed at protecting personal data. Companies using AI must ensure their systems comply with these regulations. Case study: A healthcare provider failed to adequately secure patient data used to train its AI diagnostic system, resulting in a data breach and significant fines. Another case study demonstrated the importance of anonymizing data used in AI development to protect user privacy.
In conclusion, ethical considerations are paramount in AI integration. Organizations must prioritize fairness, transparency, and accountability in the development and deployment of AI systems. Ignoring these aspects can lead to negative consequences and undermine public trust in AI. A responsible approach to AI integration requires proactive measures to mitigate bias, ensure data privacy, and foster transparency.
The Skill Gap and Talent Acquisition Challenges
The successful integration of AI requires a skilled workforce capable of developing, deploying, and maintaining these complex systems. However, there is a significant shortage of AI talent globally, creating a major challenge for organizations seeking to adopt AI technologies. This skill gap spans various roles, including data scientists, machine learning engineers, and AI ethicists. The demand for these specialized skills far outpaces the supply, driving up salaries and making it difficult for companies to find and retain qualified professionals. Case study: A survey of leading tech companies revealed that the majority of them struggled to find qualified AI engineers, indicating a severe talent shortage in the industry. Another case study analyzed the salaries of AI professionals and found that they significantly outpaced those in other tech roles, demonstrating the high demand for these skills.
Addressing this skill gap requires a multi-pronged approach. Educational institutions need to invest in developing robust AI curricula to produce a pipeline of skilled graduates. Companies can also play a role by providing training and development opportunities for their existing employees. This can involve offering courses, workshops, and mentorship programs to upskill their workforce and prepare them for roles in the AI field. This proactive approach is essential for enabling organizations to leverage the benefits of AI while mitigating the risks associated with a lack of expertise. Case study: One company successfully addressed the skill gap by partnering with universities to develop specialized AI programs, creating a pool of talent specifically tailored to their needs. Another company implemented a comprehensive training program for its existing employees, allowing them to acquire the necessary skills for working with AI systems.
Moreover, organizations must prioritize diversity and inclusion in their AI talent acquisition strategies. A diverse workforce brings different perspectives and expertise, leading to more innovative and effective AI solutions. This is particularly important given the potential for bias in AI systems. Diverse teams are better equipped to identify and mitigate bias in the development and implementation of AI technologies. Case study: A company demonstrated a successful approach by establishing a diversity-focused recruitment strategy to attract and retain top AI talent from diverse backgrounds. Another case study showed that companies with diverse AI teams developed more robust and less biased AI models compared to companies with homogeneous teams.
In conclusion, the skill gap in AI is a significant challenge, but it is not insurmountable. A collaborative effort between educational institutions, industry, and government is necessary to cultivate a diverse and skilled workforce capable of driving innovation and responsible AI integration. Proactive measures, including investment in education and training, diversity initiatives, and talent attraction strategies, are crucial for ensuring successful AI implementation.
Data Security and Privacy Concerns in AI
The reliance of AI systems on vast amounts of data creates significant security and privacy vulnerabilities. Protecting this data from unauthorized access, theft, or misuse is paramount. Data breaches can have devastating consequences, leading to financial losses, reputational damage, and legal liabilities. Moreover, the use of sensitive personal data in AI systems raises ethical and legal concerns related to privacy. Companies need to implement robust security measures to protect data throughout its lifecycle. Case study: A major social media company experienced a significant data breach, compromising the personal information of millions of users, highlighting the importance of robust data security practices. Another case study demonstrated how a company’s failure to protect sensitive user data resulted in significant fines and reputational damage.
AI systems themselves can be targets of cyberattacks. Adversaries can attempt to manipulate AI models, introduce malicious code, or exploit vulnerabilities to gain unauthorized access to data or systems. Robust cybersecurity measures, including regular security audits, penetration testing, and intrusion detection systems, are essential to mitigate these risks. These measures must be integrated into the entire AI lifecycle, from development and deployment to ongoing maintenance and updates. Case study: A company successfully mitigated a potential AI system vulnerability by implementing a rigorous security testing program that identified and addressed potential weaknesses before they could be exploited. Another case study showed the importance of continuous monitoring of AI systems to detect and respond to security threats.
Compliance with data privacy regulations is another crucial aspect of data security in AI. Regulations like GDPR in Europe and CCPA in California impose stringent requirements on how organizations collect, use, and protect personal data. AI systems must be designed and implemented to comply with these regulations to avoid legal penalties and reputational harm. Case study: A company successfully implemented GDPR compliance measures within its AI systems by designing data governance processes and implementing data anonymization techniques. Another case study examined the practical challenges faced by organizations in complying with data privacy regulations when integrating AI systems.
In conclusion, data security and privacy are paramount in the responsible integration of AI. Organizations must prioritize the protection of data throughout its lifecycle, implement robust security measures, and comply with relevant regulations to mitigate risks and build trust. A proactive and comprehensive approach to data security is essential for successful and ethical AI deployment.
Future Trends and Implications of AI Integration
The integration of AI is poised to accelerate in the coming years, leading to transformative changes across industries. Advancements in machine learning, natural language processing, and computer vision are driving the development of more sophisticated and capable AI systems. These systems will have far-reaching implications for various sectors, from healthcare and finance to manufacturing and transportation. We can expect to see increased automation, personalized experiences, and improved decision-making across various aspects of life. Case study: The healthcare industry is expected to see significant advancements in AI-powered diagnostics, drug discovery, and personalized medicine. Another case study examines the potential of AI in revolutionizing the transportation industry through the development of autonomous vehicles.
The increasing availability of data and improved computing power are fueling the growth of AI. The exponential growth of data generated by various sources, coupled with advancements in cloud computing and specialized hardware, enables the development and deployment of increasingly complex AI models. This trend will continue to drive innovation and adoption of AI across a wide range of applications. Case study: The growth of cloud computing is enabling organizations of all sizes to access the computational power needed to train and deploy advanced AI models. Another case study demonstrates how the availability of large datasets is enabling the development of more accurate and sophisticated AI models in various fields.
However, the widespread adoption of AI also presents potential challenges and risks. The ethical considerations discussed earlier, along with concerns about job displacement, economic inequality, and the potential for misuse of AI, need to be addressed proactively. Responsible AI development and deployment are crucial to ensure that AI benefits society as a whole. Policymakers, researchers, and industry leaders must work together to establish ethical guidelines and regulations to guide the development and use of AI. Case study: A panel of experts discussed the importance of responsible AI development and the need for ethical guidelines to govern its use. Another case study examines the potential impact of AI on the workforce and strategies for mitigating job displacement.
In conclusion, the future of AI integration is bright, but it is crucial to approach it responsibly. Addressing the ethical, societal, and economic implications of AI is just as important as driving technological advancements. A collaborative effort is essential to harness the transformative potential of AI while mitigating its risks and ensuring a future where AI benefits everyone.
Conclusion
The integration of AI presents both immense opportunities and significant challenges. While the potential benefits are undeniable, a comprehensive understanding of the hidden costs, ethical implications, skill gap, data security concerns, and future trends is crucial for successful and responsible implementation. Organizations must move beyond the hype and delve into the practical realities of AI integration, planning strategically for the financial, operational, and human resource implications. A proactive approach to ethical considerations, talent acquisition, data security, and regulatory compliance is vital for navigating the complexities of this transformative technology and ensuring that its benefits are widely shared. Only through a thoughtful and responsible approach can we fully harness the transformative potential of AI and build a future where it serves humanity's best interests.
Ultimately, successful AI integration requires a holistic approach that considers the entire ecosystem – from technical infrastructure and talent acquisition to ethical considerations and future implications. It's not simply about adopting the technology; it’s about understanding its complexities, mitigating its risks, and harnessing its power for positive change. Only then can organizations truly unlock the transformative potential of AI and realize its benefits responsibly. The journey requires foresight, planning, and a commitment to responsible innovation.