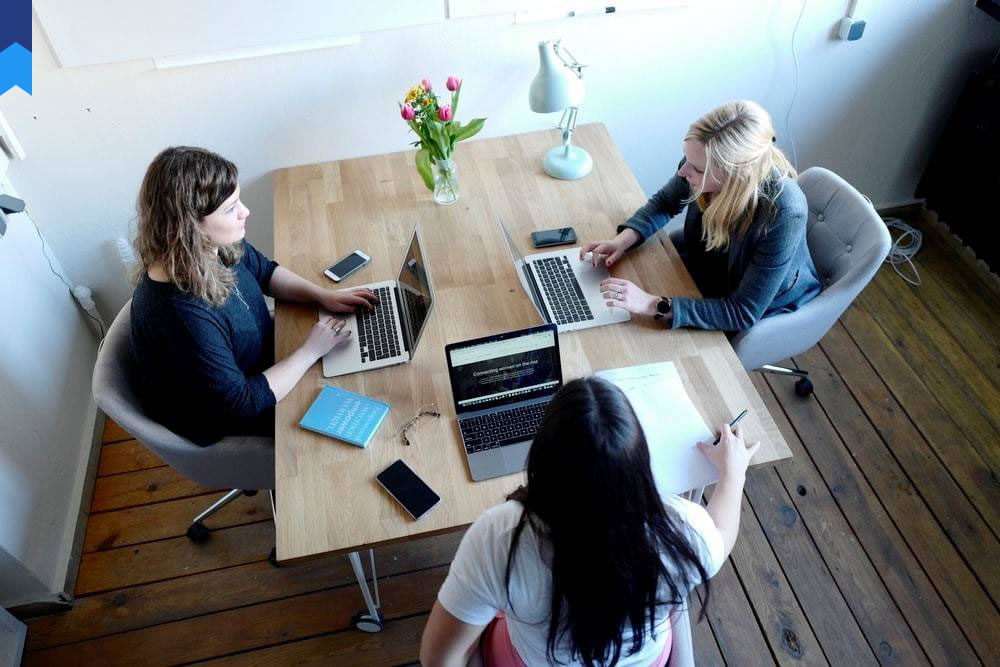
Key Features of the Common Data Model: Enhancing Healthcare Data Standardization
The Observational Medical Outcomes Partnership (OMOP) Common Data Model (CDM) is a pioneering framework designed to facilitate the collection, standardization, and analysis of healthcare data. As the volume of observational healthcare data continues to grow, the need for a unified approach to data management becomes increasingly critical. The OMOP CDM plays a vital role in enabling researchers, clinicians, and public health officials to derive meaningful insights from diverse datasets. Here, we explore the key features of the OMOP Common Data Model that contribute to its effectiveness and utility in healthcare research.
1. Standardized Vocabulary
One of the foundational features of the OMOP CDM is its use of a standardized vocabulary. The model incorporates recognized coding systems, such as:
- SNOMED CT: For clinical terms and concepts related to diagnoses, procedures, and symptoms.
- LOINC: For laboratory tests and measurements.
- RxNorm: For medication names and dosages.
By standardizing terminologies, the OMOP CDM ensures that data from various sources can be harmonized, making it easier to analyze and compare findings across different studies and institutions. This uniformity reduces ambiguity and enhances data interoperability, allowing researchers to draw accurate conclusions.
2. Modular Structure
The OMOP common data model is designed with a modular structure that allows for flexibility and adaptability. The core model consists of essential tables and fields that capture critical healthcare information, but additional tables can be integrated to accommodate specific research needs. This modularity enables organizations to tailor the model to their unique datasets and study requirements, enhancing its applicability across various healthcare contexts.
3. Support for Observational Research
The primary focus of the OMOP CDM is to support observational research. Unlike randomized controlled trials, observational studies examine real-world outcomes and treatment effects as they occur in everyday clinical settings. The OMOP CDM is specifically designed to facilitate this type of research, enabling researchers to analyze large-scale data for insights on drug safety, disease progression, and healthcare utilization patterns. This emphasis on observational data allows for a deeper understanding of how treatments perform in diverse patient populations.
4. Data Quality and Governance
Ensuring data quality is a core principle of the OMOP CDM. The model provides guidelines for data governance, emphasizing the importance of accurate data collection and management practices. By establishing clear standards and definitions, the OMOP CDM helps organizations maintain high-quality datasets that are reliable for research purposes. This focus on data quality is crucial for building trust in the findings derived from observational studies.
5. Enhanced Analytical Capabilities
The OMOP CDM is designed to support a wide range of analytical methodologies. Researchers can utilize various statistical techniques, machine learning models, and data mining approaches to extract insights from the standardized datasets. By enabling complex analyses, the OMOP CDM helps uncover patterns and associations that can inform clinical decision-making, public health strategies, and health policy.
6. Interoperability and Data Sharing
One of the standout features of the OMOP CDM is its commitment to interoperability. The model facilitates the sharing of data among researchers and institutions, breaking down silos that often hinder collaborative efforts. By adhering to a common framework, organizations can more easily exchange data and collaborate on studies, leading to larger datasets and more robust findings. This collaborative approach is essential for advancing knowledge in healthcare and improving patient outcomes.
7. Open-Source Framework
The OMOP CDM operates as an open-source initiative, which means that it is freely available for researchers and institutions to use, modify, and contribute to. This openness fosters a collaborative research environment, encouraging innovation and the continuous improvement of the model. As new data sources, technologies, and research methods emerge, the OMOP CDM can evolve to accommodate these changes, ensuring its relevance in the ever-changing landscape of healthcare.
8. Support for Real-World Evidence Generation
The OMOP CDM is instrumental in generating real-world evidence (RWE), which is increasingly recognized as critical for understanding treatment effectiveness and patient outcomes in everyday clinical practice. By leveraging observational data, the OMOP CDM helps researchers assess how well treatments work outside of controlled environments. This capability is vital for informing clinical guidelines and policy decisions, ensuring that healthcare practices are based on robust evidence.
9. Global Adoption and Collaboration
The OMOP CDM has gained traction worldwide, with numerous healthcare organizations, research institutions, and academic centers adopting the model. This global adoption fosters international collaboration, enabling researchers to conduct multi-site studies and share insights across borders. By building a worldwide network of institutions utilizing the OMOP CDM, the model contributes to a richer understanding of health and disease on a global scale.
Conclusion
The OMOP Common Data Model represents a significant advancement in the standardization and analysis of healthcare data. Its key features—including standardized vocabulary, modular structure, support for observational research, and emphasis on data quality—make it an invaluable resource for researchers and healthcare organizations. As the demand for data-driven insights in healthcare continues to grow, the OMOP CDM will play an increasingly vital role in facilitating collaboration, improving patient outcomes, and driving advancements in public health. Embracing this model is essential for harnessing the full potential of observational healthcare data in the quest for improved health outcomes.
Related Courses and Certification
Also Online IT Certification Courses & Online Technical Certificate Programs