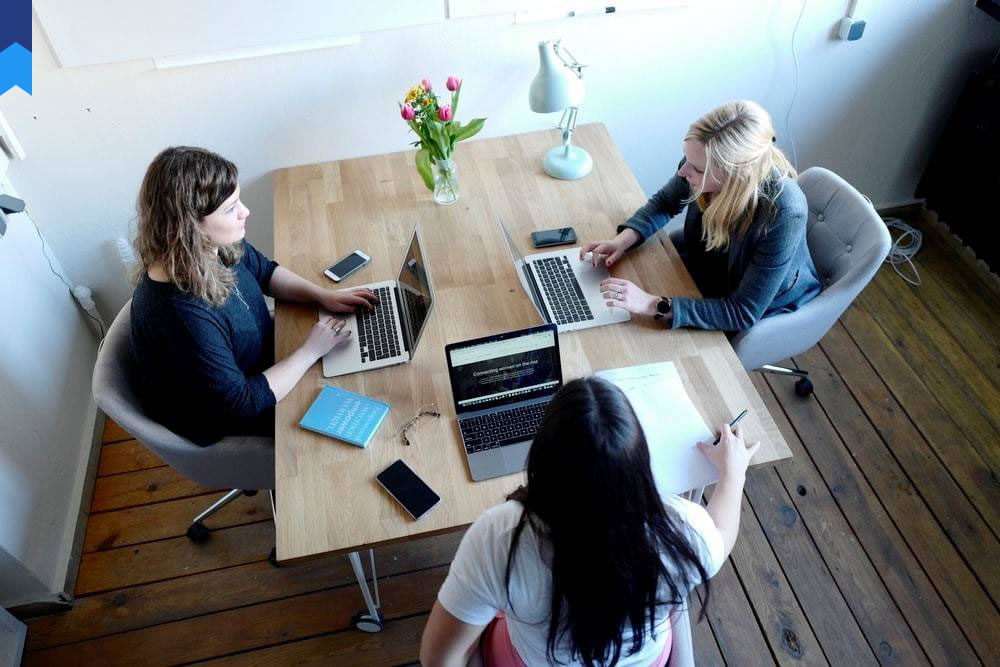
What Is Machine Learning: Definition and Examples
The process of generalization requires classifiers that input discrete or continuous feature vectors and output a class. Machine learning (ML) is a branch of artificial intelligence that systematically applies algorithms to synthesize the underlying relationships among data and information. For example, ML systems can be trained on automatic speech recognition systems (such as iPhone’s Siri) to convert acoustic information in a sequence of speech data into semantic structure expressed in the form of a string of words. As you can see, there are many applications of machine learning all around us.
The profession of machine learning definition falls under the umbrella of AI. Rather than being plainly written, it focuses on drilling to examine data and advance knowledge. It entails the process of teaching a computer to take commands from data by assessing and drawing decisions from massive collections of evidence.
Visual Representations of Machine Learning Models
This makes it possible to build systems that can automatically improve their performance over time by learning from their experiences. Computers can learn, memorize, and generate accurate outputs with machine learning. It has enabled companies to make informed decisions critical to streamlining their business operations. Such data-driven decisions help companies across industry verticals, from manufacturing, retail, healthcare, energy, and financial services, optimize their current operations while seeking new methods to ease their overall workload. Firstly, the request sends data to the server, processed by a machine learning algorithm, before receiving a response. Instead, a time-efficient process could be to use ML programs on edge devices.
- The emergence of ransomware has brought machine learning into the spotlight, given its capability to detect ransomware attacks at time zero.
- So her experience (E) increased, her performance(P) also increased, and then we noticed that as the number of attempts at this toy increased.
- This requires massive amounts of raw data for processing — and the more data that is received, the more the predictive model improves.
- To ensure a decisive advantage, data mining in such cases should be a continuous and online process.
When training a machine learning model, machine learning engineers need to target and collect a large and representative sample of data. Data from the training set can be as varied as a corpus of text, a collection of images, sensor data, and data collected from individual users of a service. Overfitting is something to watch out for when training a machine learning model. Trained models derived from biased or non-evaluated data can result in skewed or undesired predictions.
How Machine Learning Tools Can Enhance UX Design
Incidentally, Google isn’t just using machine learning; it’s providing tools for developers to create their own machine learning applications. Developers no longer need to be data scientists to create data mining applications or integrate speech recognition into the search function of their apps. Google also uses machine learning to improve search engine performance, power Google Translate with help from advanced NLP and cutting-edge artificial neural networks. Machine learning can also help decision-makers figure out which questions to ask as they seek to improve processes.
ML algorithms even allow medical experts to predict the lifespan of a patient suffering from a fatal disease with increasing accuracy. Machine learning is being increasingly adopted in the healthcare industry, credit and sensors such as wearable fitness trackers, smart health watches, etc. All such devices monitor users’ health data to assess their health in real-time.
Machine learning vs. artificial intelligence.
Read more about https://www.metadialog.com/ here.
Related Courses and Certification
Also Online IT Certification Courses & Online Technical Certificate Programs