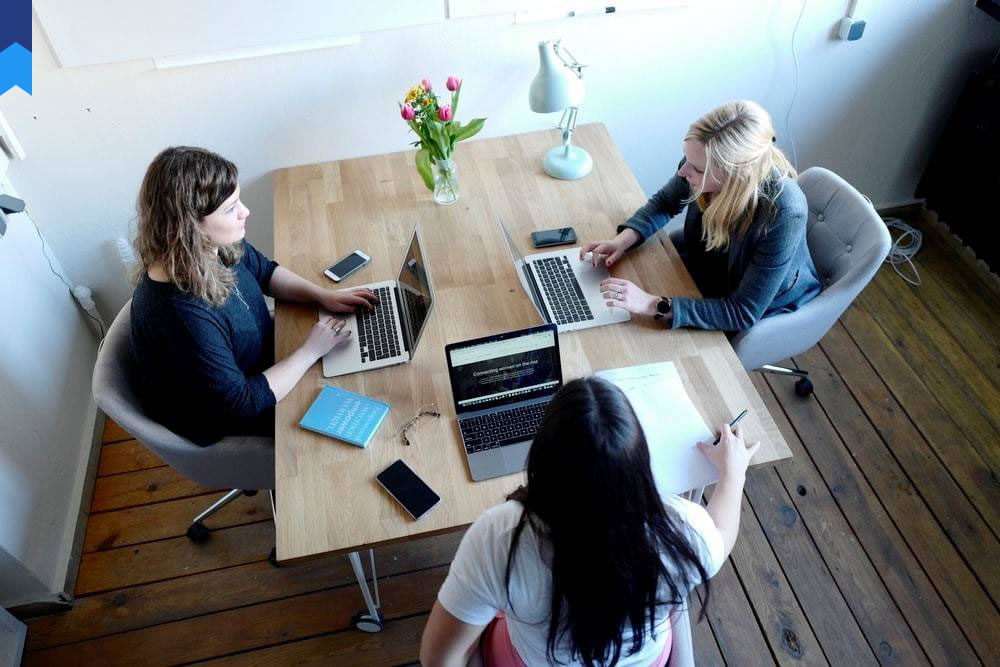
2025 Tech Outlook: What IT Decision-Makers Need to Know
AI applications within the enterprise are poised to become mainstream as companies transition from early experimentation to full-scale adoption. Most organizations are currently in the preliminary phases of integrating generative AI into their workflows, and they are beginning to see real, tangible business benefits. For instance, Nutanix, a company actively working with generative AI, aims to boost its developer productivity by 25%. This improvement is expected to stem from the use of generative AI for tasks such as code generation for unit testing and other critical functions. As businesses continue to explore these applications, AI will likely start to have a profound impact across multiple sectors, enhancing operations and optimizing various enterprise functions.
In response to the growing influence of AI, IT leaders will need to allocate significantly more resources to fund AI initiatives in order to maintain a competitive edge. While AI is undeniably expensive to implement and maintain, its benefits are clear, and this pressure will result in IT departments being forced to maximize operational efficiency. One of the key advantages of AI is its ability to identify inefficiencies within business operations, allowing companies to automate labor-intensive tasks and streamline workflows. This, in turn, could free up valuable resources and budget. As organizations invest more heavily in AI, IT leaders may adopt a “fund AI with AI” strategy, ensuring that the very systems designed to drive innovation and efficiency can sustain and support future advancements. This approach could also lead to the phase-out of non-AI products that fail to keep pace with evolving technological standards.
The increased adoption of Kubernetes, particularly for mission-critical applications, is expected to prompt a shift in how companies manage their infrastructures. Rather than leaving developers to manage Kubernetes clusters on their own, organizations will increasingly adopt a centralized approach to Kubernetes management. This will provide enhanced security, more cost-effective operations, and greater consistency across the enterprise. As Kubernetes becomes a central part of the enterprise IT landscape, its central management will play a crucial role in reducing operational complexity and improving performance.
In the realm of software development, the emergence of Software 2.0 is set to mark a significant shift in how enterprise software is created and managed. Tools like Copilot have already democratized software engineering, making it easier for a broader range of users to engage in development processes. However, the transformation of enterprise software is just beginning. By 2025, the next generation of software will evolve to learn from its usage over time. These programs will continuously adapt and improve the user experience without the need for direct intervention from developers. This new generation of self-improving software will lead to a paradigm shift in how applications are built, marking the beginning of what is expected to be a 20-year era of software evolution, where traditional workflows will give way to smarter, more adaptive solutions.
Blockchain technology, once hailed as a revolutionary force, is primed for a comeback, fueled by both regulatory fiat and the increasing need for systems that offer publicly verifiable records. As cryptocurrencies also experience a resurgence, blockchain will find new applications beyond its initial association with digital currencies. The political climate, particularly with expectations of a crypto-friendly administration, has already had a measurable effect on the price of Bitcoin, pushing it above $100,000. This renewed focus on blockchain will help it gain mainstream acceptance, particularly as industries look for more robust and transparent record-keeping solutions.
One of the critical issues that will emerge as AI adoption grows is the rising power consumption associated with AI inference at the edge. The energy demands of AI models, particularly during the inference phase, are expected to outstrip initial projections. In the United States, the projected energy demand is set to double over the next four years, reaching approximately 880 terawatt-hours (TW). If optimizations are not made, this could result in a dramatic increase in the carbon footprint of AI operations. Contrary to popular belief, AI inference, not just training, will become the most significant consumer of power. This poses a challenge for enterprises, which will need to invest in more energy-efficient infrastructure to avoid excessive energy costs and reduce their environmental impact.
The rapid advancements in AI are also pushing us closer to achieving artificial general intelligence (AGI). Reasoning models, such as OpenAI’s o1, along with competition from Meta’s LLama and Alibaba’s AI systems, are set to significantly enhance the capabilities of AI. These advanced models will be able to perform a wider array of tasks, demonstrating more flexibility and adaptability than current AI systems. This marks a critical step toward AGI, where machines will possess a deeper understanding and reasoning ability, making them capable of tackling far more complex and nuanced challenges.
The leadership landscape in generative AI will become increasingly open, small, and global. Open-source AI models are gaining traction, and we will see a shift towards these models outpacing their closed counterparts in terms of performance. Not only will open generative AI models become more capable, but they will also be able to learn from larger, more powerful models through processes like model compression. This will allow them to scale efficiently and be more accessible to a wider audience, including smaller enterprises and startups.
With the rise of multi-agent AI systems, the next frontier in AI innovation will involve the creation of systems where multiple AI agents cooperate and collaborate. This approach will require new methodologies, tools, and processes to facilitate coordination between agents. As these systems become more complex, AI agents will increasingly interact with one another, even negotiating to achieve desired outcomes. The challenge for enterprises will be in managing these systems effectively, ensuring that the agents work together seamlessly to achieve business objectives.
AI inference will become even more important as reasoning models and multi-agent systems gain prominence. The computational resources required to run these systems will put increasing pressure on enterprise infrastructure, requiring businesses to make substantial investments in both infrastructure and power efficiency. Companies will need to adopt new strategies for scaling their AI capabilities, including optimizing the way they handle inference workloads.
Cloud robotics will soon enter the enterprise, opening up new opportunities for industries like manufacturing. As these technologies become more advanced, businesses will invest in shared services and data infrastructure to support them. This will result in a new approach to infrastructure management, where systems are designed to accommodate both software agents and physical robots in a collaborative environment. The use of cloud robotics will enable businesses to optimize their operations, reduce costs, and increase automation, allowing them to stay competitive in a rapidly changing marketplace.
Emerging technologies and architectures will fuel the next generation of AI models and multi-agent systems. These innovations will help enterprises manage the increasing complexity of AI models while improving performance and efficiency. ARM architecture, which is known for its energy-efficient processing capabilities, will become more widespread, helping to power next-generation AI models that require vast computational resources.
To address the growing demand for memory bandwidth in AI tasks, in-memory compute architectures will gain popularity. Similar to Apple’s M4-style converged architectures, which combine high memory bandwidth with processing power, in-memory computing will enable faster, more efficient AI operations, particularly for tasks that rely heavily on data processing.
Finally, Smart NICs (Network Interface Cards) will evolve to take on more advanced roles, such as running entire storage controllers. This shift will help optimize network and storage performance, providing businesses with more efficient and scalable infrastructure solutions as they continue to rely on AI and other advanced technologies. These innovations will play a critical role in enabling the next generation of AI-driven enterprise operations.
Related Courses and Certification
Also Online IT Certification Courses & Online Technical Certificate Programs