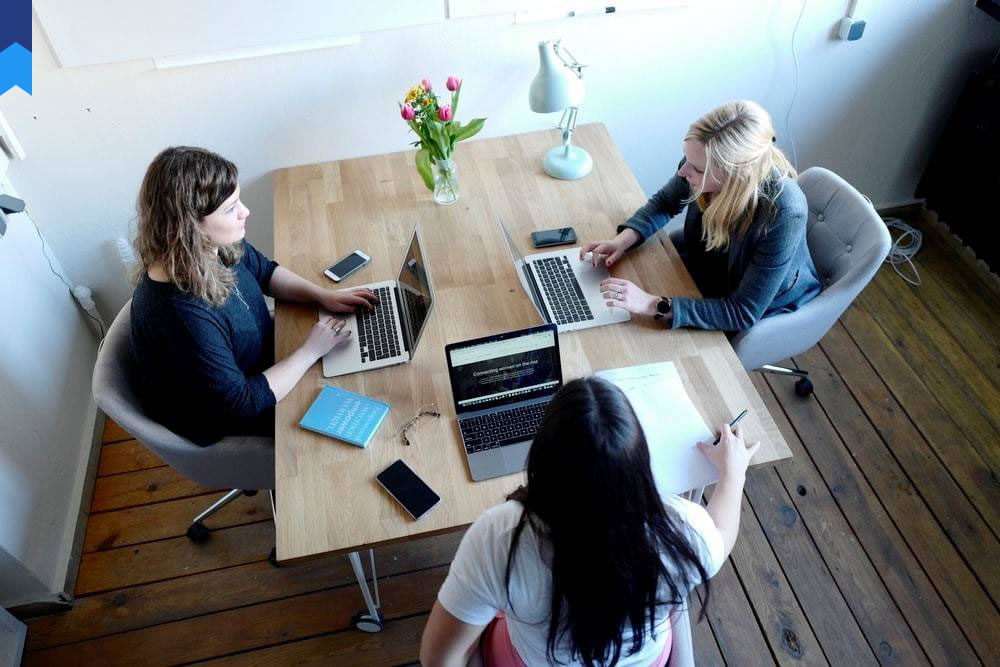
With Journalism in Decline, I’ll Take in the Fumes’: A Chatbot Helper’s Revelations
The text delves into the role human writers play in the training of large language models (LLMs) like ChatGPT, and the irony of being employed to improve technology that could one day replace them. Despite the impressive capabilities of LLMs in automating linguistic tasks, from drafting emails to generating essays, their proficiency relies heavily on extensive human input. Writers, including journalists, academics, and novelists, are being employed to provide high-quality training data for these models, ensuring they produce reliable outputs while minimizing errors such as "hallucinations," or factual inaccuracies.
The core task for human annotators working with large language models (LLMs) involves generating responses to hypothetical chatbot queries, which serve as high-quality examples of “good” writing for the AI to learn from. This process is essential for training the models to perform well, ensuring they can emulate human-like responses that are clear, grammatically correct, and informative. These examples form the "gold standard" that the AI aims to replicate, helping to improve its performance and make its output more coherent and useful.
While LLMs are trained on enormous datasets of text, including content from the internet, books, and other written sources, this vast body of text isn't always enough to guarantee optimal results. Human annotators are necessary because LLMs, despite their access to a broad array of data, still face challenges in generating high-quality, accurate, and relevant responses. One of the key reasons human intervention is so crucial is the phenomenon of "model collapse," a problem that arises when AI models are trained too heavily on synthetic data, or data generated by other AI models, rather than original, human-created content.
Model collapse happens because AI-generated data lacks the richness, diversity, and subtlety found in human writing. When LLMs are fed synthetic data in large quantities, their outputs begin to degrade, becoming more repetitive, less accurate, and even nonsensical over time. This degenerative process occurs because AI models are, at their core, sophisticated text-prediction machines, designed to anticipate what words or phrases are most likely to follow in a given context based on patterns in their training data. When the data is primarily AI-generated, it tends to reflect a narrow, repetitive set of language patterns, eroding the model's ability to generate nuanced or creative responses.
This reliance on synthetic data leads to a loss of what researchers call "minority data"—rare words, unique expressions, or unconventional knowledge that are less likely to appear in AI-generated content. Over time, the model forgets how to handle these rare data points, leading to a narrowing of its knowledge base and a decrease in the quality of its responses. As a result, the AI's performance suffers, making it less reliable and less capable of handling complex or diverse language tasks.
To prevent this from happening, human annotators play a vital role in training LLMs by providing fresh, original content that reflects the diversity of human language. By feeding the models carefully curated, high-quality examples of human writing, annotators help the AI maintain its ability to understand and produce a wide range of linguistic expressions. This ensures that the models don’t collapse into producing generic or inaccurate responses and can continue to improve in their ability to generate human-like language.
In addition to creating examples of "good" writing, human annotators also help LLMs avoid other issues, such as hallucinations—when the AI confidently provides incorrect or fabricated information. By offering examples that cite sources and integrate factual information, annotators guide the models toward more accurate and trustworthy outputs. This step is crucial for ensuring that AI-generated content remains reliable and credible, especially as these models are increasingly used in areas where accuracy is critical, such as customer service, education, and healthcare.
Despite the massive amounts of text available on the internet and in published works, human involvement remains a key factor in ensuring the continued development and success of LLMs. These models rely on human intervention to provide the kind of nuanced, detailed, and contextually appropriate language that allows them to function effectively in real-world applications. Without this ongoing input, LLMs risk becoming less reliable and less capable of fulfilling the tasks for which they were designed. Ultimately, while large language models may have made incredible advances in generating human-like text, they still depend on human creativity, expertise, and intervention to reach their full potential.
One of the key reasons human intervention remains critical is that AI models, when trained solely on synthetic data, lose their ability to capture the diversity of rare or nuanced information. As a result, language models need fresh, human-generated data to continue improving and avoid perpetuating biases or producing poor-quality results. This human involvement has spurred the growth of a high-paying industry for writers who provide this training data, even though it paradoxically sustains the very technology that threatens to make certain types of writing jobs obsolete.
The text also touches on the financial pressures facing AI companies, particularly as the expectations for continuous technological breakthroughs grow. Companies like OpenAI are pouring substantial resources into securing original content and human annotators to train their models, with licensing agreements and data budgets consuming significant portions of their financial resources. However, some experts predict that these massive investments may soon face scrutiny from investors, potentially resulting in tighter budgets for human annotation. As AI models improve, the balance between human input and automated processes may shift, raising questions about the sustainability of current spending levels on data acquisition and annotation.
Ultimately, while human writers are indispensable in the development and refinement of large language models (LLMs) today, the future remains uncertain. As AI continues to evolve, the need for human input could diminish, with technology potentially reaching a point where it can train itself more effectively, reducing reliance on human-created data. For now, however, human annotators serve a crucial function, supplying the models with the nuanced, high-quality data needed to improve their outputs and mitigate errors like hallucinations. Despite their importance, the role of human writers may be a fleeting necessity, a temporary phase in AI’s broader trajectory towards self-sufficiency and the ability to perform more complex tasks autonomously. As technology advances, the need for manual intervention could gradually fade, but in this current stage of AI development, human input is the vital "fuel" driving progress.
Related Courses and Certification
Also Online IT Certification Courses & Online Technical Certificate Programs