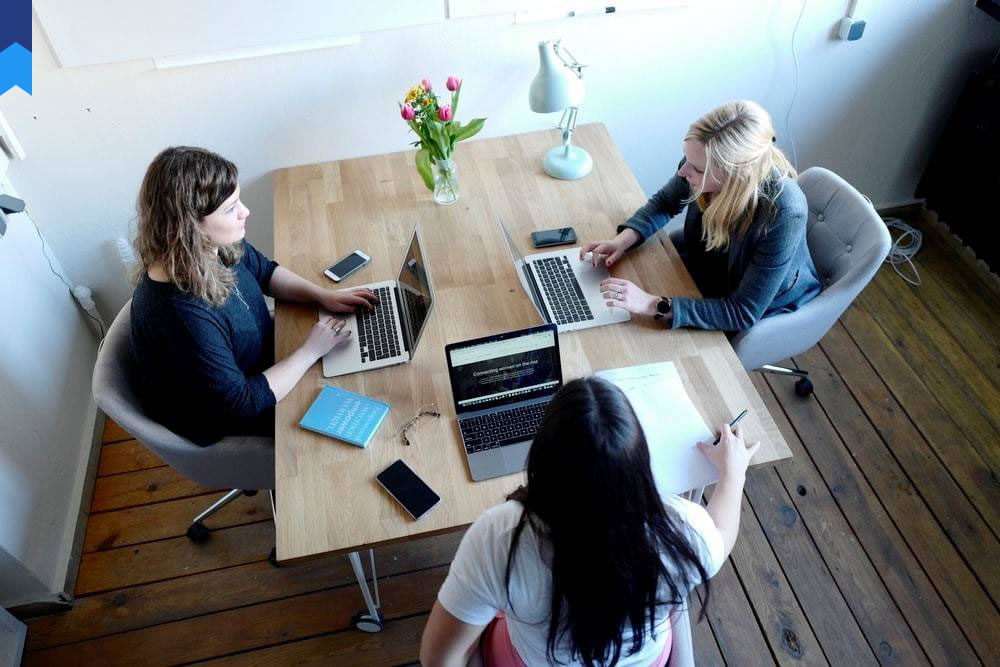
“Inner Workings Revealed: Grasping the Mechanics of A/B Testing Behind the Scenes”
A/B testing, also known as split testing, is a dynamic methodology that allows businesses to refine and optimize digital strategies based on real-time user data. Beneath its seemingly simple surface lies a complex set of mechanics that govern the process. In this exploration, we unveil the inner workings of A/B testing, shedding light on the intricacies that drive successful experimentation and optimization.
The Foundation: Randomization and Control Groups
Randomization:
Random assignment of users to different variants is the bedrock of A/B testing. It ensures a fair and unbiased distribution, minimizing the influence of external factors that could skew results. This randomness is crucial for obtaining reliable and statistically significant insights.
Control Group:
The control group, often referred to as group A, serves as the baseline against which the variant (group B) is compared. The control group experiences the existing or default version, allowing for a precise evaluation of the impact of changes introduced in the variant.
Metrics and KPIs: Navigating the Data Landscape
Quantifiable Metrics:
A/B testing relies on quantifiable metrics to measure user behavior and interactions. These metrics can include click-through rates, conversion rates, bounce rates, and other key performance indicators (KPIs) that align with the specific goals of the test.
Primary and Secondary Metrics:
While primary metrics directly reflect the test's primary goal (e.g., conversion rate for an e-commerce site), secondary metrics provide additional context and help assess broader impacts on user experience.
Statistical Significance: Deciphering Reliable Results
Statistical Tools:
Sophisticated statistical tools are employed to analyze the data and determine whether observed differences between variants are statistically significant. This involves assessing the likelihood that the results are not due to random chance.
P-Values and Confidence Intervals:
P-values and confidence intervals are key statistical measures. A low p-value indicates that the observed differences are unlikely to be due to chance, while confidence intervals provide a range within which the true effect is likely to fall.
A/B Testing Process: Step-by-Step Unveiling
Identification of Goals:
The process begins with a clear identification of goals and objectives. These could range from increasing conversion rates to improving user engagement.
Selection of Elements:
Careful consideration goes into selecting specific elements for testing. These could include headlines, images, calls-to-action (CTAs), or any other components that impact user behavior.
Creation of Variants:
Distinct versions or variants are created for the selected elements. Each variant represents a different approach or design, and users are randomly assigned to experience one of these variants.
Implementation of the Test:
The A/B test is deployed to the target audience, and users are exposed to either the control or variant, based on random assignment.
Data Collection and Analysis:
User interactions are monitored, and relevant metrics are collected. Statistical tools are then employed to analyze the data, determining the significance of observed differences.
Conclusion Drawing:
Based on the analysis, conclusions are drawn regarding which variant performed better. This involves not only statistical significance but also an assessment of the practical significance of the findings.
Implementation of Changes:
Successful A/B testing leads to informed decision-making. Changes based on insights gained from the test are implemented, and the iterative process begins anew.
Best Practices: Crafting a Roadmap for Success
Testing One Variable at a Time:
Isolating variables ensures that the impact on user behavior can be accurately attributed to specific elements, avoiding confounding factors.
Leveraging Statistical Significance:
Ensuring that results are statistically significant before drawing conclusions is fundamental to reliable insights.
User Segmentation:
Considering different user segments separately allows for more nuanced insights, as user behavior may vary based on demographics or other factors.
Prioritizing High-Impact Elements:
Focusing on elements with the potential for significant impact on user behavior ensures that A/B testing resources are efficiently allocated.
Continuous Testing:
A/B testing is an iterative process. Regularly testing and refining strategies ensures ongoing optimization and adaptation to changing user behaviors.
Tools of the Trade: Platforms Facilitating A/B Testing
Google Optimize:
A versatile tool offering A/B testing, multivariate testing, and personalization features.
Optimizely:
A comprehensive experimentation platform catering to web and mobile applications.
VWO (Visual Website Optimizer):
A user-friendly platform for A/B testing, split URL testing, and multivariate testing.
Unbounce:
Primarily designed for A/B testing of landing pages, providing insights into page performance.
Conclusion:
Unraveling the Complexity for Strategic Mastery
The inner workings of A/B testing unveil a carefully orchestrated process that goes beyond the surface of simple comparison. Randomization, control groups, statistical significance, and meticulous testing practices form the backbone of successful experimentation. As businesses strive for strategic mastery, understanding and leveraging the mechanics of A/B testing empowers them to crack the code to informed decision-making, continuous optimization, and a data-driven approach to digital strategy evolution.
SIIT Courses and Certification
Also Online IT Certification Courses & Online Technical Certificate Programs